Signal-control refined dynamic traffic graph model for movement-based arterial network traffic volume prediction.
Expert Syst. Appl.(2023)
摘要
Forecasting the forthcoming intersection movement-based traffic volume enables adaptive traffic control systems to dynamically respond to the fluctuation of traffic demands. In this paper, a deep-learning based Signal-control Refined Dynamic Traffic Graph (ScR-DTG) Model is proposed for advancing the network-level movement-based traffic volume prediction task. The proposed model attempts to further improve the-state-of-art and practice algorithms in traffic prediction for arterial network adaptive signal control utilizing tradition traffic flow theory boosted deep-learning methodology. For precisely inferencing the movement-based demand at cycle-to-cycle level, the proposed model incorporates spatial graph convolution inferencing layer and temporal inferencing layer to explore both the intricate spatial temporal dependencies, respectively. A signal control refining module is contrived to deduce the controlled movement saturation flow and introduce the essential control inferences, which is of great significance but frequently neglected in the previous researches. Additionally, according to the real-time movement specified travel time, this paper creatively constructs an adjacent graph with dynamic order for more accurately capturing the ever-changing spatial relevancies. Field experiments with multiple signal schemes were conducted in the downtown area of Zhangzhou (China). The promising results demonstrated the state-of-the-art accuracy than other high-performance volume prediction algorithms. Implementing the proposed model enables to obtain accurate movement-based volume predictions, which would assist the traffic management agencies in adjusting signal timing adaptively and further improve the efficiency of signal intersection.
更多查看译文
关键词
traffic,prediction,signal-control,movement-based
AI 理解论文
溯源树
样例
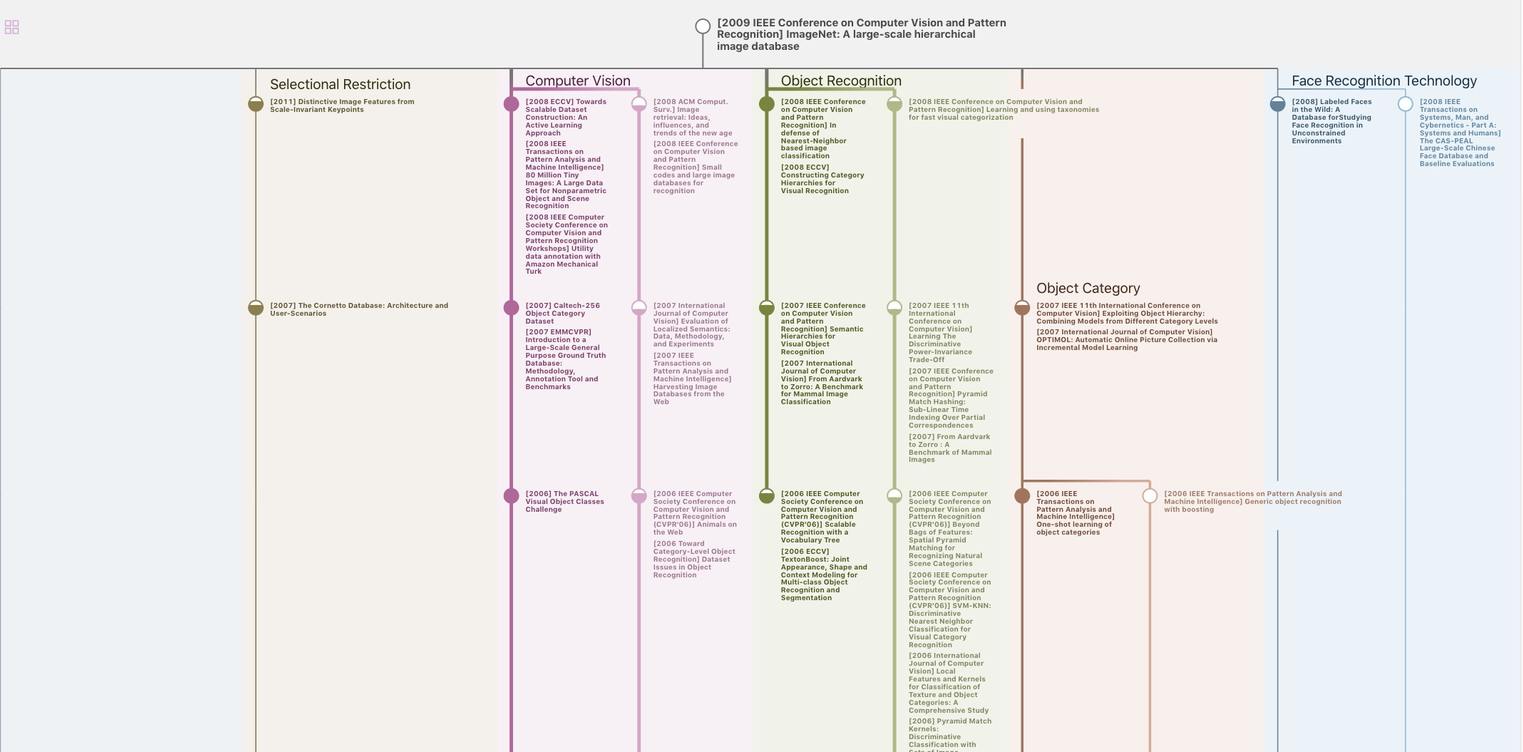
生成溯源树,研究论文发展脉络
Chat Paper
正在生成论文摘要