Graph-Based Active Learning With Uncertainty and Representativeness for Industrial Anomaly Detection.
IEEE Trans. Instrum. Meas.(2023)
摘要
Anomaly detection is essential for automated industrial production. Sufficiently labeled samples play an important role in improving the detection capability of the model. However, existing anomaly detection methods cannot balance the detection performance against the labeling cost in practice owing to the lack of an effective and efficient sample selection strategy. To address this issue, a graph-based active anomaly detection (AAD) method called GAAD is proposed in this article. In the proposed method, a graph structure is adopted to rapidly spread the labeling information, and a heuristic strategy is designed to select samples combined with uncertainty and representativeness, which can rapidly and adequately explore the sample distribution with limited labeled samples. Moreover, a simple but effective autoencoder (AE) is proposed to confuse low-level features and preserve the locality by a pretrained model, which achieves a better image encoding and anomaly detection performance. We created a new through-hole technology (THT) solder joint dataset and conducted extensive comparative experiments with mainstream active and semisupervised anomaly detection methods on both the THT and the publicly available NEU datasets. In the experiments, GAAD achieved the best detection performance and had an extremely low update time of 0.0071 s during active learning, demonstrating its superiority for industrial applications.
更多查看译文
关键词
active learning,anomaly detection,uncertainty,graph-based
AI 理解论文
溯源树
样例
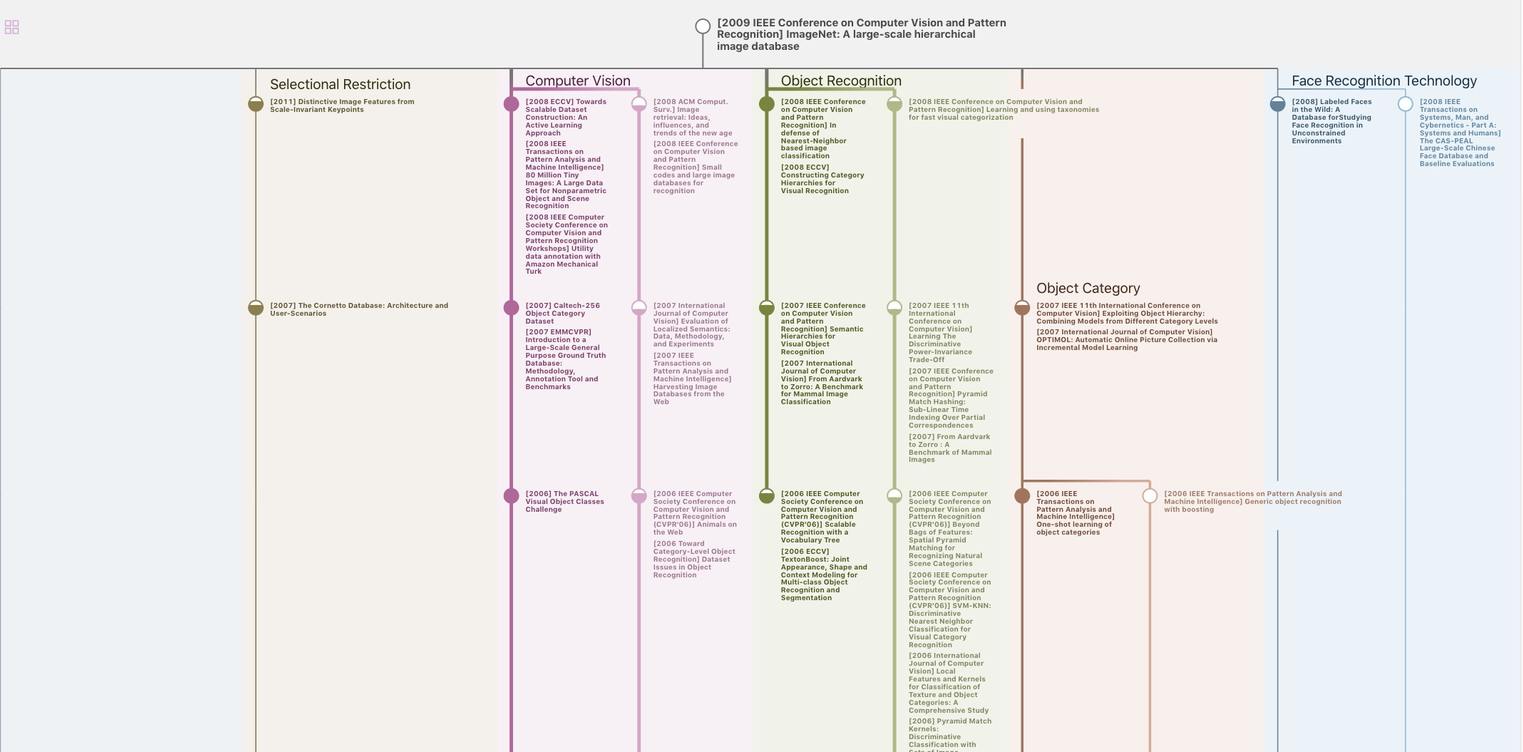
生成溯源树,研究论文发展脉络
Chat Paper
正在生成论文摘要