An Ensemble Machine Learning Technique for Stroke Prognosis.
Comput. Syst. Sci. Eng.(2023)
摘要
Stroke is a life-threatening disease usually due to blockage of blood or insufficient blood flow to the brain. It has a tremendous impact on every aspect of life since it is the leading global factor of disability and morbidity. Strokes can range from minor to severe (extensive). Thus, early stroke assessment and treatment can enhance survival rates. Manual prediction is extremely time and resource intensive. Automated prediction methods such as Modern Information and Communication Technologies (ICTs), particularly those in Machine Learning (ML) area, are crucial for the early diagnosis and prognosis of stroke. Therefore, this research proposed an ensemble voting model based on three Machine Learning (ML) algorithms: Random Forest (RF), Extreme Gradient Boosting (XGBoost), and Light Gradient Boosting Machine (LGBM). We apply data preprocessing to manage the outliers and useless instances in the dataset. Furthermore, to address the problem of imbalanced data, we enhance the minority class’s representation using the Synthetic Minority Over-Sampling Technique (SMOTE), allowing it to engage in the learning process actively. Results reveal that the suggested model outperforms existing studies and other classifiers with 0.96% accuracy, 0.97% precision, 0.97% recall, and 0.96% F1-score. The experiment demonstrates that the proposed ensemble voting model outperforms state-of-the-art and other traditional approaches.
更多查看译文
关键词
ensemble machine learning technique,stroke,machine learning,prognosis
AI 理解论文
溯源树
样例
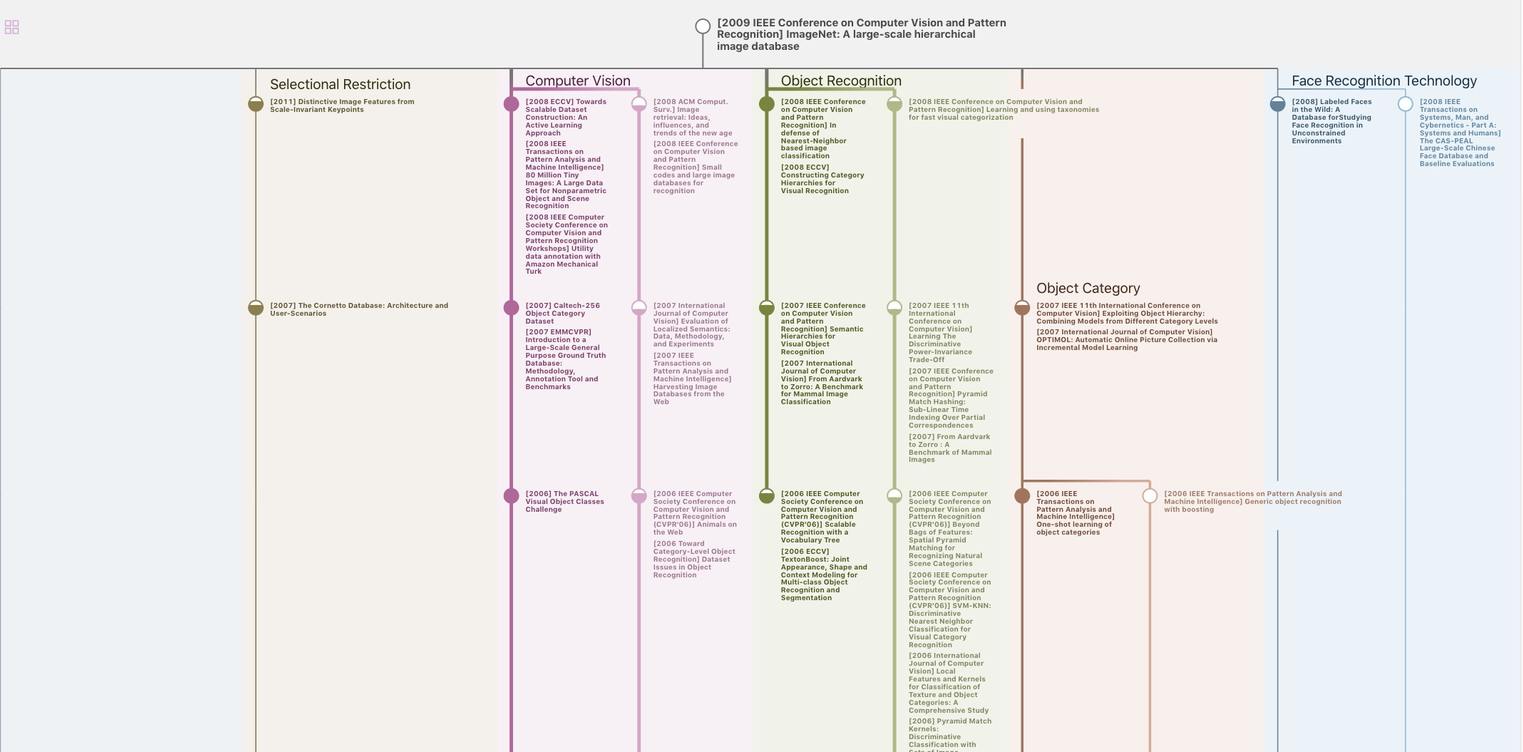
生成溯源树,研究论文发展脉络
Chat Paper
正在生成论文摘要