Hybrid mutation moth flame optimization with deep learning-based smart fabric defect detection.
Comput. Electr. Eng.(2023)
摘要
The occurrence of faults in textile manufacturing methods results in major wastage of the properties. Additionally, it also affects the quality of the fabric products. Manual inspection methods fail to detect the defects with respect to efficiency, accuracy, and consistency due to size of the detects, carelessness, and optical illusion. Therefore, automatic fabric inspection has become a powerful tool to optimize the quality of fabric. In this background, the current study develops a novel Hybrid Mutation Moth Flame Optimization with Deep Learning-Based Smart Fabric Defect Detection (HMFODL-FDD) technique for sustainable manufacturing. The proposed HMFODL-FDD technique exploits the Computer Vision (CV) and Deep Learning (DL) techniques for the detection of defects in fabric. To accomplish this objective, the presented HMFODL-FDD technique employs contrast enhancement process to boost the quality of the images. For feature extraction, the HMFODL-FDD technique uses Inception v3 model with HMFO algorithm-based hyperparameter optimizer. Finally, the classification of the fabric defects is performed using Back Propagation Neural Network (BPNN) model. The experimental outcomes confirmed that the proposed HMFODL-FDD technique achieved superior performance over DL techniques in terms of effective defect classification in fabric images with a maximum accuracy of 95.47%.
更多查看译文
关键词
Fabric defect detection,Deep learning,Textile industry,Smart manufacturing,Sustainability
AI 理解论文
溯源树
样例
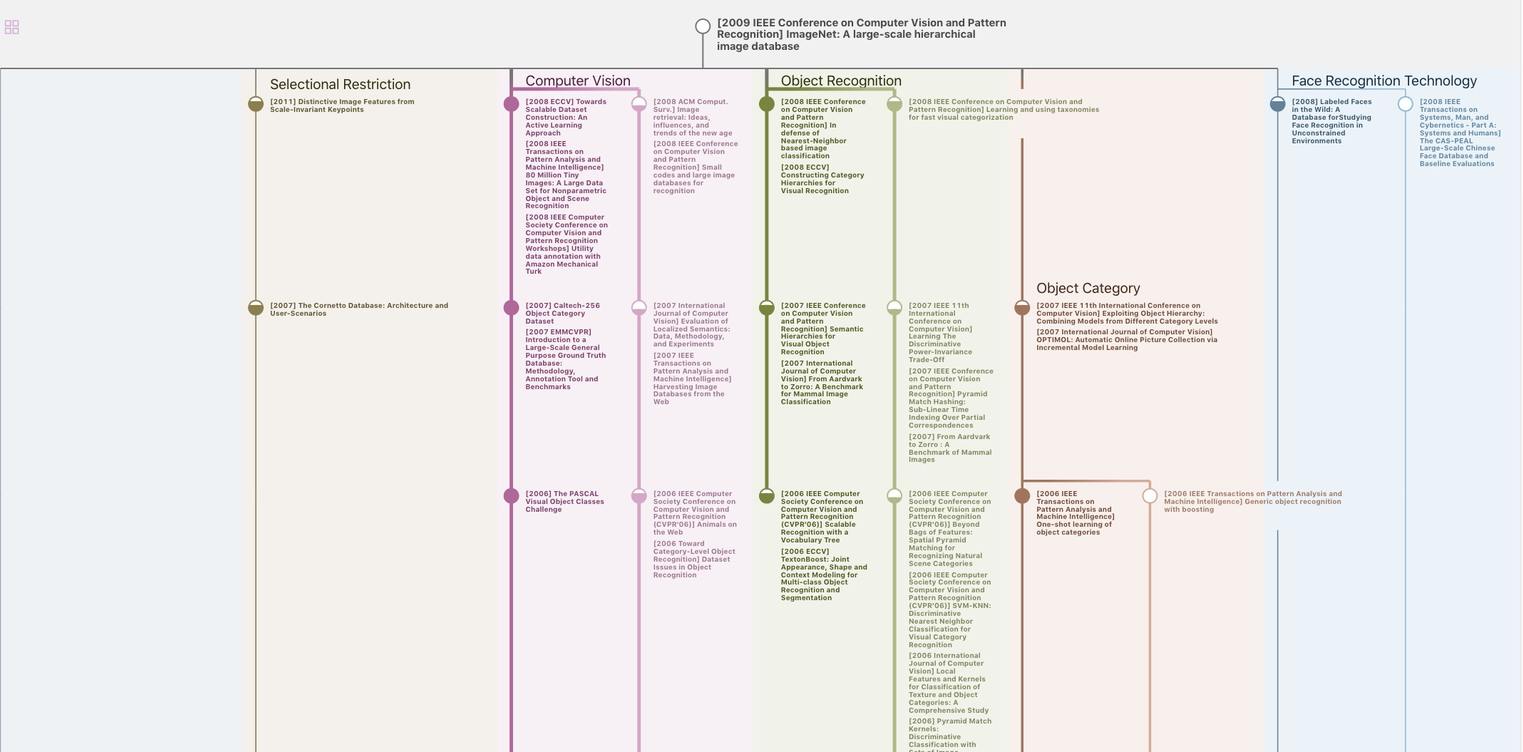
生成溯源树,研究论文发展脉络
Chat Paper
正在生成论文摘要