GREB: gradient re-balanced loss for long-tailed multi-lable classification
J. Ambient Intell. Humaniz. Comput.(2023)
摘要
Image classification has witnessed a remarkable advancement in class-balanced benchmarks. However, the natural distribution of datasets in real-world scenarios are long-tailed. Long-tailed classification has become a significant challenge in critical real-world image classification applications. A deep learning network trained on a long-tailed dataset tends to classify tail classes with few samples as head classes with many samples. The severe sample imbalance leads to the overwhelming dominance of negative samples on the tail classes; then, the massive gradient descent of negative samples leads to the classifier’s performance poorly. To tackle this problem, we propose a gradient re-balanced (GREB) loss with two synergistic factors, i.e., balance factor and correction factor. First, GREB estimates the balance and correction factors by accumulating the classifier outputs and their corresponding labels during the training process. Then, GREB dynamically reweights the gradients of positive and negative samples based on the balance factor to minimize the classification bias and improve the classifier performance. Finally, GREB compensates for sample gradients based on the correction factor to minimize the occurrence of misclassifications and improve the precision rate. Experiment results show that our GREB loss achieves state-of-the-art performance on long-tailed multi-label classification datasets (MSCOCO and MultiMNIST) and long-tailed single-label classification datasets (CIFAR10-LT and CIFAR100-LT).
更多查看译文
关键词
Long-tailed Classification,Multi-label Classification,Long-tailed Multi-label Classification,Rebalancing Methods
AI 理解论文
溯源树
样例
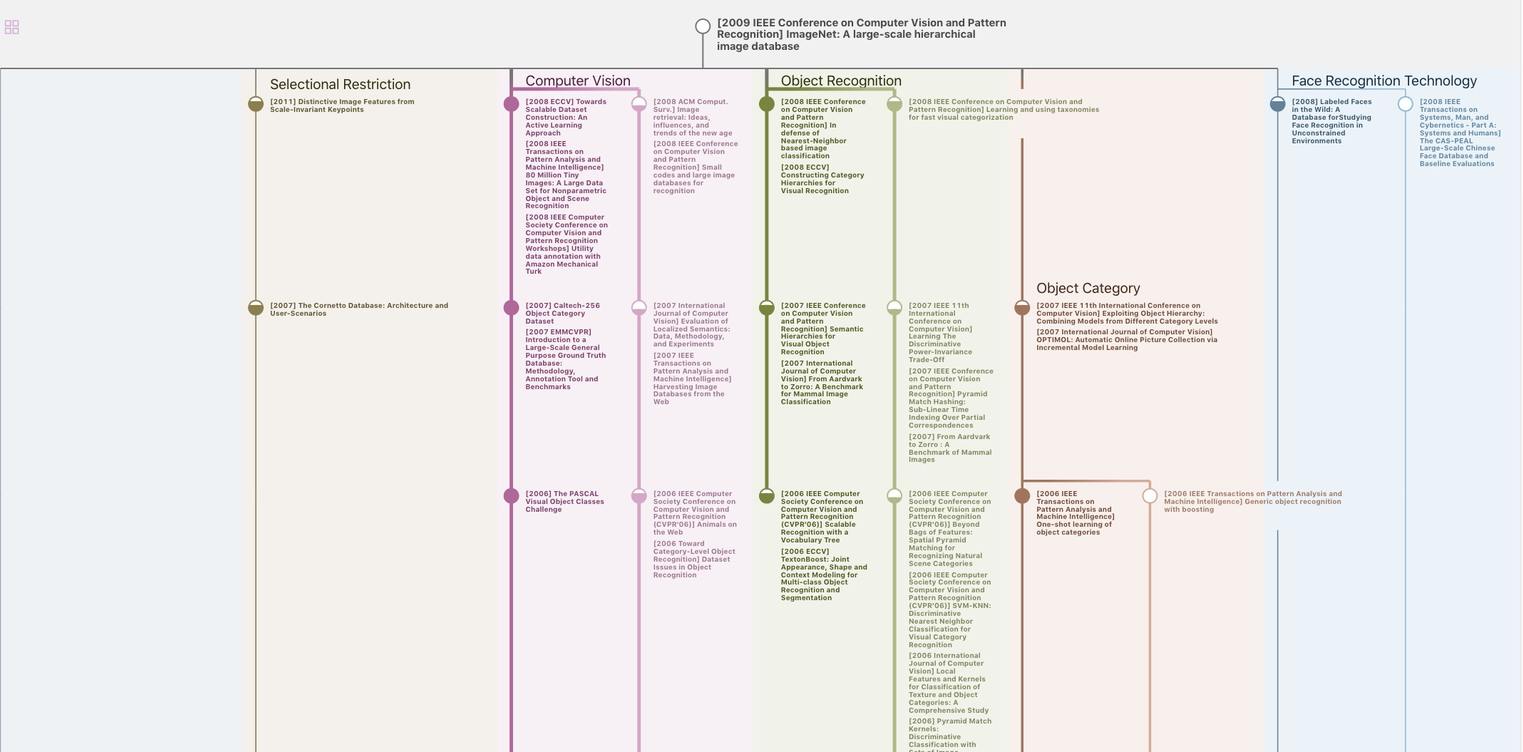
生成溯源树,研究论文发展脉络
Chat Paper
正在生成论文摘要