Medical entity recognition and knowledge map relationship analysis of Chinese EMRs based on improved BiLSTM-CRF.
Comput. Electr. Eng.(2023)
摘要
With the development of medical informatization, a large number of patients’ electronic medical records (EMRs) have been accumulated in the hospital information system, which is characterized by multi-structured data form, diversified professional vocabulary categories and fuzzy vocabulary demarcation. Natural language processing (NLP) provides a silver lining for parsing electronic medical records, and the mainstream methods include dictionary-based methods, rule-based and statistical methods, and machine learning methods. Due to the semantic richness and structural diversity of Chinese text, Chinese EMRs analysis methods are still scarce compared with English EMRs. In order to overcome the problems of unstructured, multiple meanings of words and unclear word boundaries in text of Chinese electronic medical records, this paper proposes a medical entity recognition method based on RoFormerV2-BiLSTM-CRF fusion model, using BIO annotation method to annotate the recognized medical entities, and using knowledge graph to analyze the medical entity relationships identified in single patient medical record, multiple patient medical records respectively. The relationships between the medical entities identified in a single patient record and multiple patient records are analyzed using knowledge graphs. The experimental analysis was conducted on the expert-annotated dataset CCKS2019, and the results showed that the proposed method was effective for recognizing “Diseases and Diagnoses”, “Laboratory Tests”, “Imaging Examinations”, “Anatomical Sites”, “Drugs” and “Surgery” in the dataset. The average accuracy, recall and F1-Score of the proposed method for the identification of the six medical entities, are 84.8%, 83.5% and 83.9%, respectively, which were 5.3%, 8.6% and 6.8% higher than the traditional Word2Vec-BiLSTM-CRF model, and the existing Word2Vec-BiLSTM-CRF and BERT-BiLSTM-CRF models were iteratively trained with the same evaluation. The experimental results show that the proposed model performs better in medical entity recognition.
更多查看译文
关键词
Chinese EMRs processing,Natural language processing,Deep learning,Knowledge graph
AI 理解论文
溯源树
样例
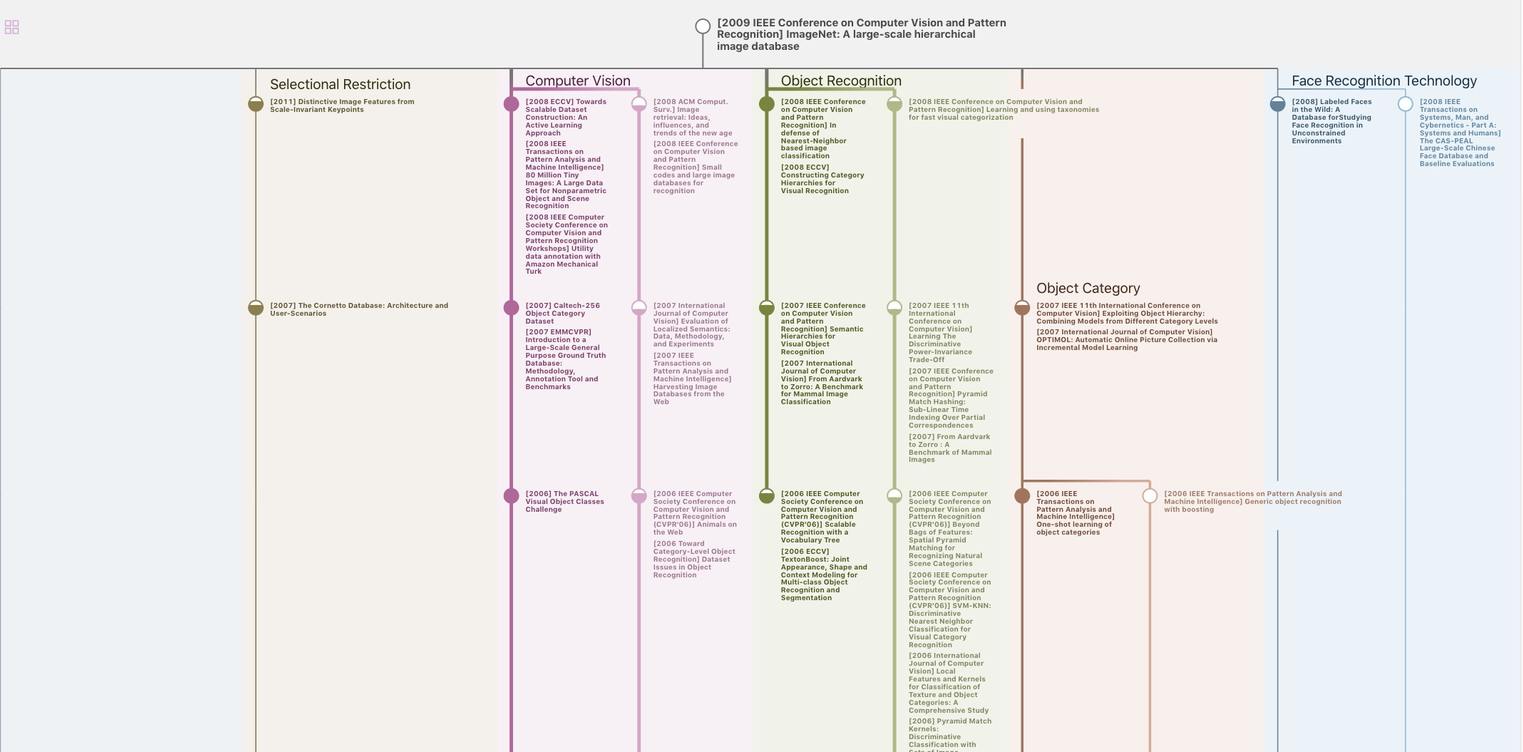
生成溯源树,研究论文发展脉络
Chat Paper
正在生成论文摘要