Learning precise feature via self-attention and self-cooperation YOLOX for smoke detection.
Expert Syst. Appl.(2023)
摘要
Smoke detection is a key process for fire warning systems. However, the existing smoke detection methods are insufficient to extract precise smoke features due to the smoke’s transparency and variability. To solve this problem, we adopt the efficient YOLOX architecture and devise three strategies to enhance it. A self-cooperation mechanism is proposed to directly remove redundancy and then condense localization and semantic information. Moreover, we utilize the light-weight self-attention mechanism to emphasize the meaningful features of smoke. Finally, we equip the network with the piece-wise focal loss to consolidate its performance towards hard samples. The proposed method is termed as self-attention and self-cooperation YOLOX (SASC-YOLOX). In addition, we build a database that contains images from real scenes and manually annotate them, named annotated real smoke database of Xi’an Jiaotong University (XJTU-RS). SASC-YOLOX obtains 72.6% and 92.1% AP on our database and a synthetic database, respectively, outperforming the state-of-the-art methods. Extensive visualization experiments also validate that SASC-YOLOX has a strong feature extraction ability. Code is available at https://github.com/jingjing-maker/SASC-YOLOX.
更多查看译文
关键词
Deep learning,Smoke detection,Precise feature extraction,SASC-YOLOX,Self-attention mechanism,Self-cooperation mechanism
AI 理解论文
溯源树
样例
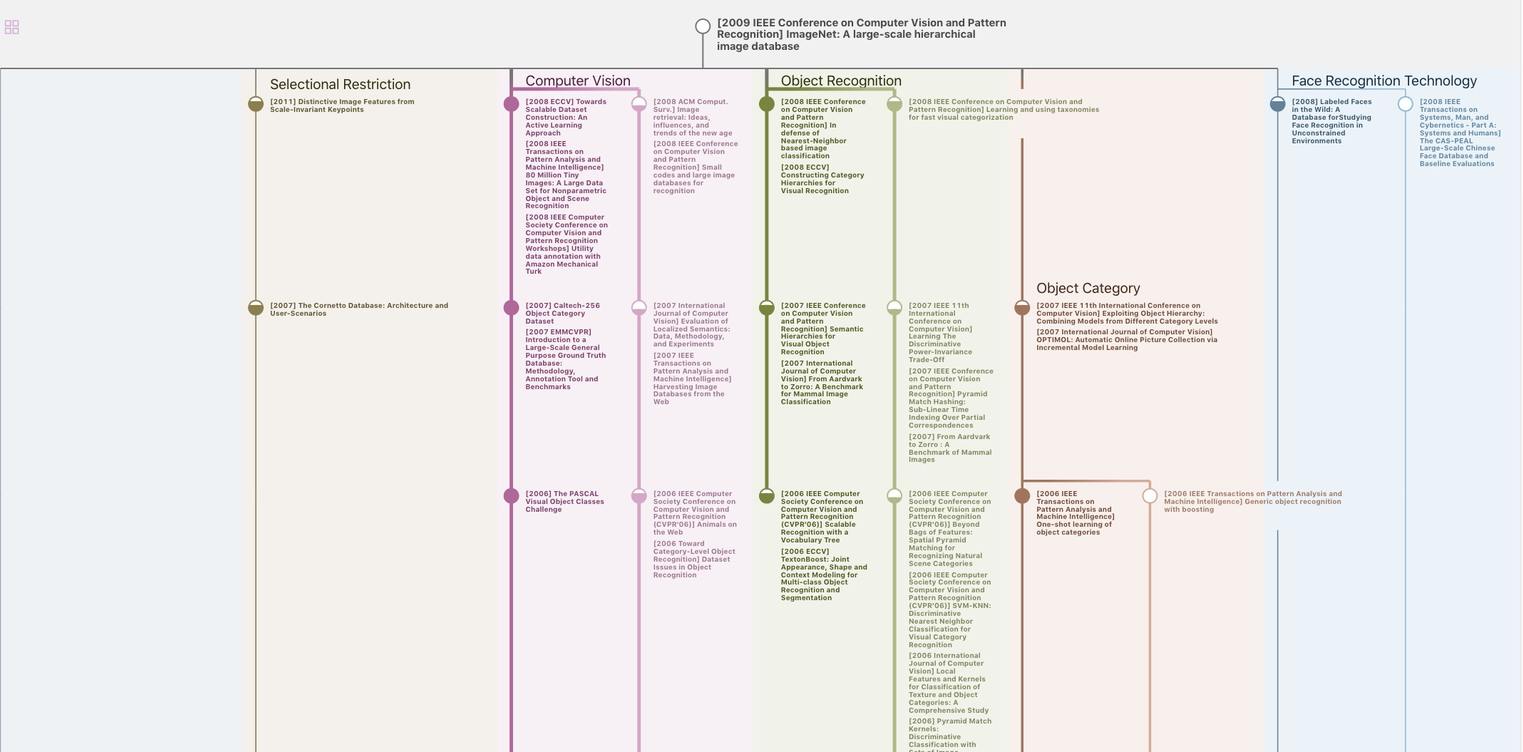
生成溯源树,研究论文发展脉络
Chat Paper
正在生成论文摘要