Automated defect identification for cell phones using language context, linguistic and smoke-word models.
Expert Syst. Appl.(2023)
摘要
Product defects are a widespread concern for manufacturers when conducting quality and customer relationship management. Prior approaches addressed many electronic products however cell phones are still unexplored. Moreover, prior work mainly focused on the lexicon, probabilistic graphic, failure mode, and effect analysis models but the utilization of word embeddings and language models are not explored. State-of-the-art contextual word embeddings and language models generate automated features and both are very effective in capturing the semantic and contextual dimensions of the text. This paper addresses these dimensions and proposes a novel approach that integrates word2vec and Bidirectional Encoder Representations from Transformers (BERT) models with smoke and domain-specific lexicon approaches to detect product defects for cell phones. Compared to existing studies, smoke and domain-specific approaches surpass the traditional sentiment methods. Moreover, BERT and word2vec presented the best performance with the logistic regression model by achieving an accuracy of 90% on training data and 84% on validation data. A case study in the Apple iPhone and Samsung cell phone industry proves the predominant performance of our approach and provides great potential in defect and customer relationship management.
更多查看译文
关键词
Cell phones,Linguistics,Language context,Defect discovery,Smoke words,Word2vec
AI 理解论文
溯源树
样例
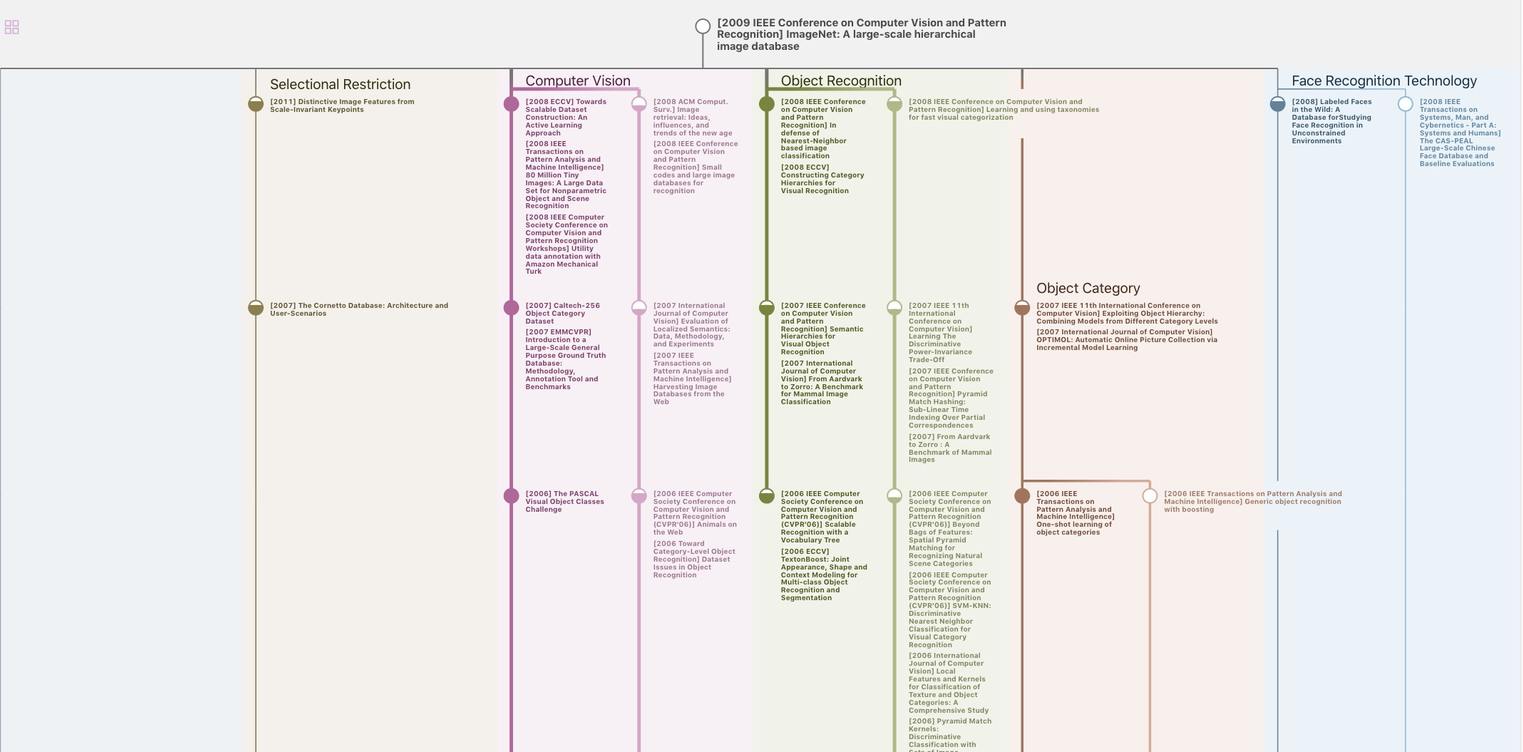
生成溯源树,研究论文发展脉络
Chat Paper
正在生成论文摘要