Optimising Stochastic Task Allocation and Scheduling Plans for Mission Workers Subject to Learning-Forgetting, Fatigue-Recovery, and Stress-Recovery Effects
Expert Systems with Applications(2023)
摘要
This study addresses the stochastic bi-objective task allocation and scheduling problems for mission workers subject to the complex (and joint) effect of learning-forgetting, fatigue-recovery, and stress-recovery processes. The mission consists of work-rest cycles with different types of tasks. Some task types are repetitive, not necessarily back-to-back, with some predecessors for other task types. The workers are multi-skilled, whose experience, learning, fatigue, and stress levels are updated, affecting their performance dynamically. A Markov Decision Process (MDP) is applied to formulate this stochastic problem, considering speed and accuracy as two measures of workforce performance. A decision could be “to repeat a task of a certain type” or “take a rest break till another worker becomes available”. The developed MDP model finds the optimal task allocation and work-break schedule for workers by minimising the sum of the tasks’ completion times and maximising their quality. Completion time is a continuous variable, and the quality index is a binary random variable, i.e., high or moderate, having a continuous probability of occurrence. The model is of a general form with a potential application in similar settings. The paper used the Sequential Greedy Assignment (SGA) and the Monte-Carlo Tree Search (MCTS) to solve the problem with their results compared. Numerical results with those from a sensitivity analysis are discussed.
更多查看译文
关键词
Markov decision process (MDP),Task planning,Optimisation,Human performance,Human factor
AI 理解论文
溯源树
样例
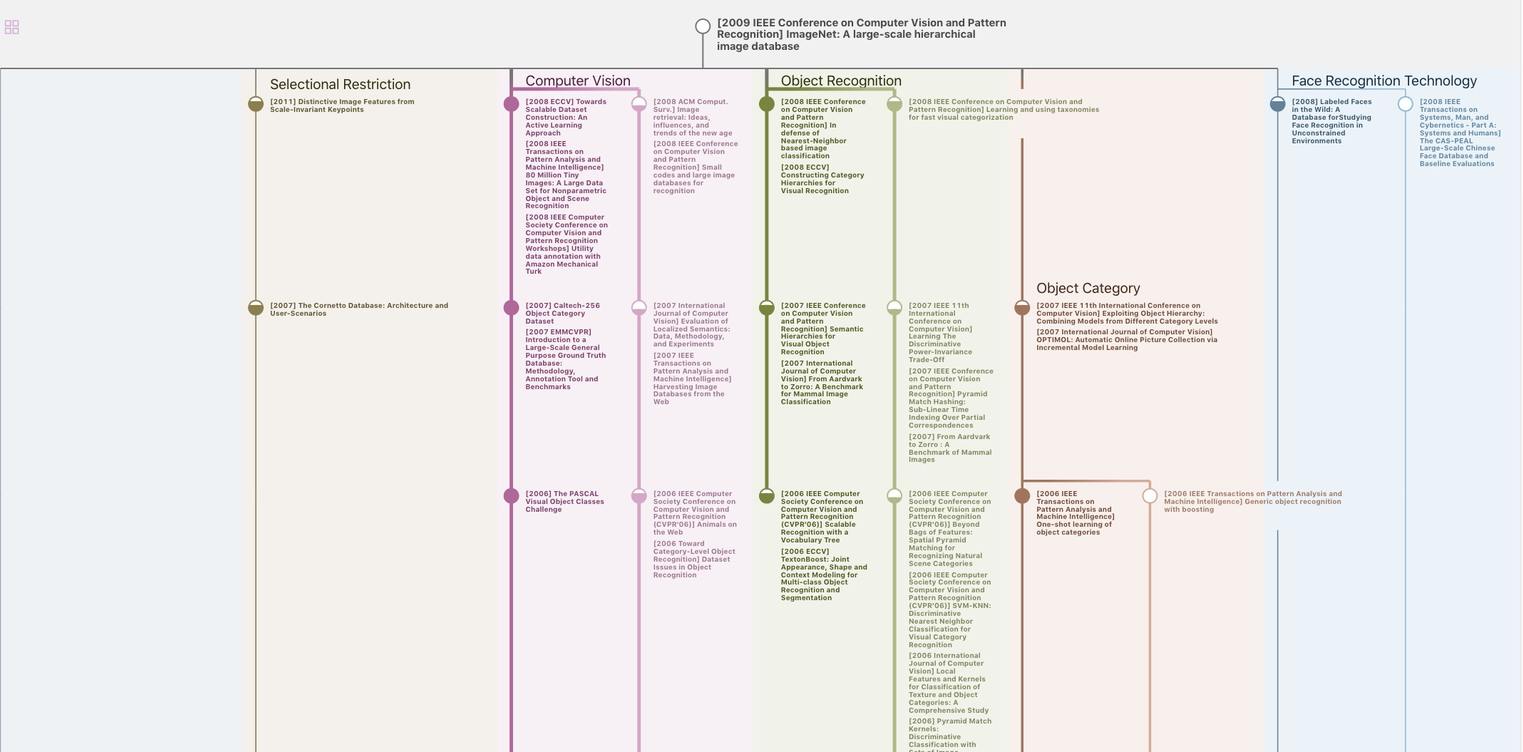
生成溯源树,研究论文发展脉络
Chat Paper
正在生成论文摘要