Data-driven quadratic stabilization and LQR control of LTI systems.
Autom.(2023)
摘要
In this paper, we propose a framework to solve the data-driven quadratic stabilization (DDQS) and the data-driven linear quadratic regulator (DDLQR) problems for both continuous and discrete-time systems. Given noisy input/state measurements and a few priors, we aim to find a state feedback controller guaranteed to quadratically stabilize all systems compatible with the a-priori information and the experimental data. In principle, finding such a controller is a non-convex robust optimization problem. Our main result shows that, by exploiting duality, the problem can be recast into a convex, albeit infinite-dimensional, functional Linear Program. To address the computational complexity entailed in solving this problem, we show that a sequence of increasingly tight finite dimensional semi-definite relaxations can be obtained using sum-of-squares and Putinar’s Positivstellensatz arguments. Finally, we show that these arguments can also be used to find controllers that minimize a worst-case (over all plants in the consistency set) closed-loop H2 cost. The effectiveness of the proposed algorithm is illustrated through comparisons against existing data-driven methods that handle ℓ∞ bounded noise.
更多查看译文
关键词
Data-driven control, Robust control, Quadratic stability, Semi-definite programming
AI 理解论文
溯源树
样例
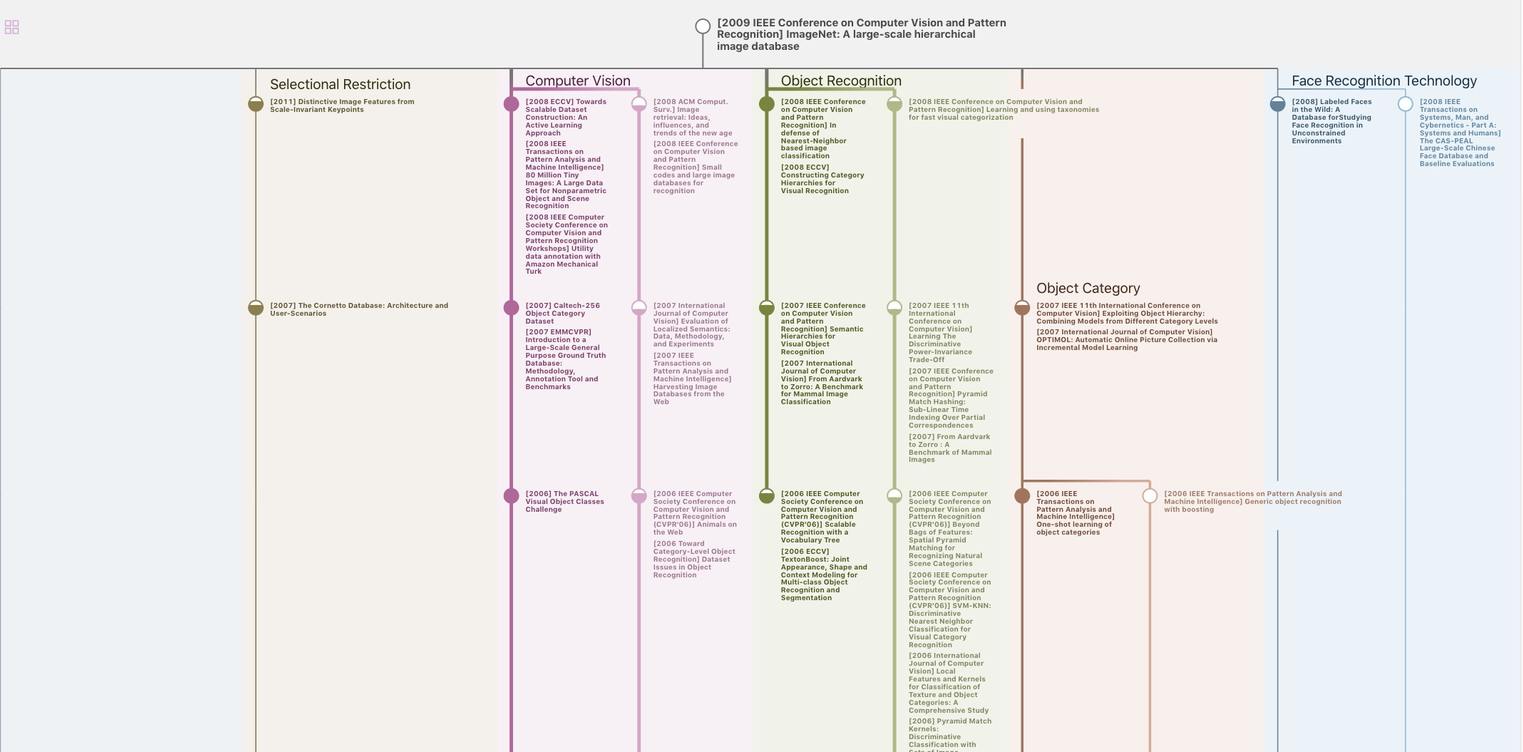
生成溯源树,研究论文发展脉络
Chat Paper
正在生成论文摘要