Editorial for special section 'ICPR 2020'.
Pattern Recognit. Lett.(2023)
摘要
Kernel logistic regression is a widely used method in machine learning applications. However, the common kernels are not flexible or representative enough due to their simplistic parametric formulation. Non-parametric learning on kernels can enlarge the representation capability of the kernel, but the low-rank property is required to suppress the model complexity. To improve the flexibility of kernels, we perform low-rank decomposition on an adjust matrix, which can explicitly control the model complexity without using complicated rank penalty terms. In this paper, we also extend our method to learn an indefinite kernel by a different decomposition. Experimental results demonstrate that the proposed learning method for non-parametric kernels outperforms other representative algorithms.
更多查看译文
关键词
‘icpr,editorial,special section
AI 理解论文
溯源树
样例
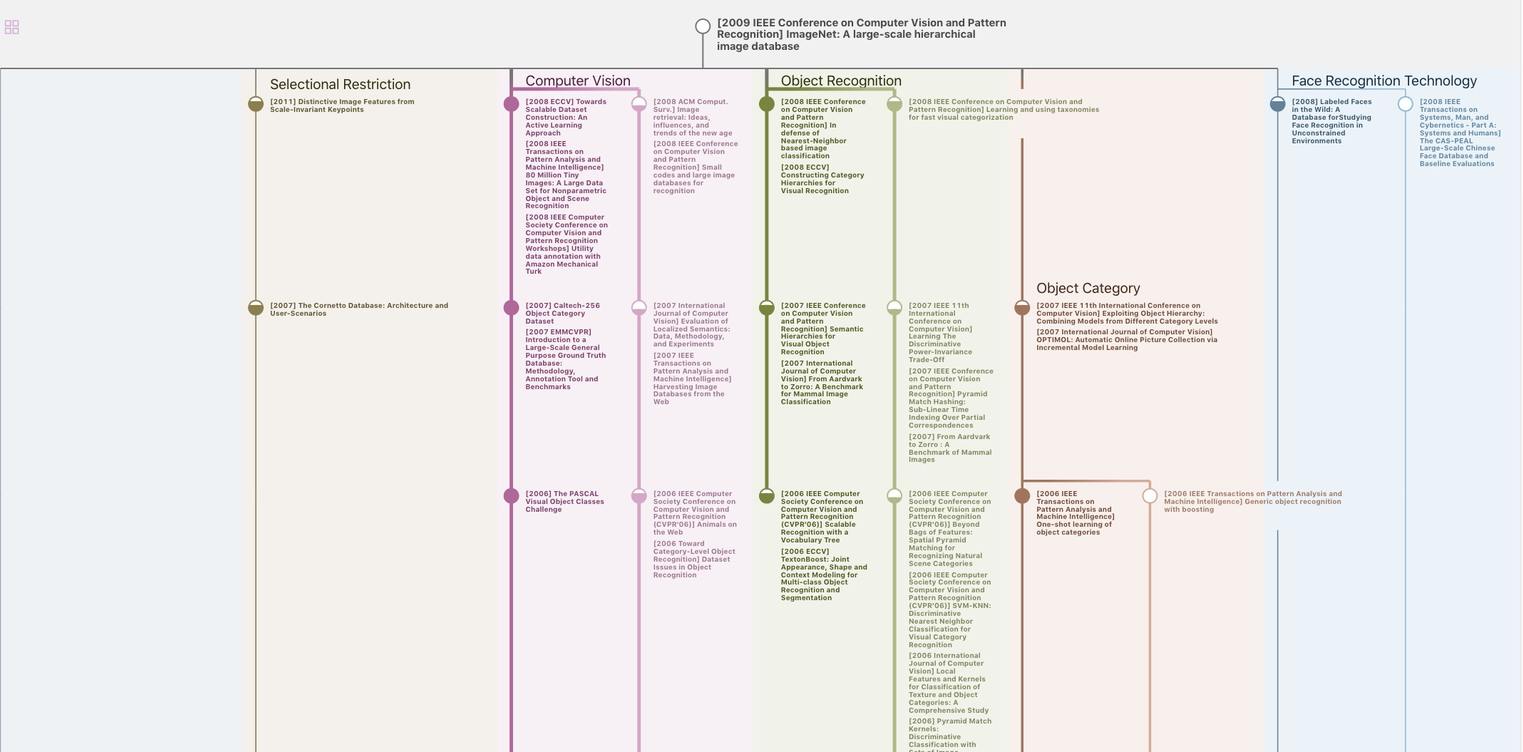
生成溯源树,研究论文发展脉络
Chat Paper
正在生成论文摘要