ProUDA: Progressive unsupervised data augmentation for semi-Supervised 3D object detection on point cloud
Pattern Recognit. Lett.(2023)
摘要
Unsupervised data augmentation (UDA) is a simple and general semi-supervised learning (SSL) frame-work. However, for the task of semi-supervised 3D object detection (SSOD-3D), due to the impact of object occlusion and point cloud resolution, the quality of pseudo labels is uncertain so that the perfor-mance of UDA is limited. In this paper, We propose an efficient and novel SSL framework progressive unsupervised data augmentation (ProUDA). At first, to minimize the overfitting risk of the inaccurate pseudo labels, we sort the unlabeled samples by prediction complexity, and present a progressive consis-tency loss to adjust the usage ratio of the unlabeled samples. After that, we employ a strategy of iteration check to select the best learning result using a few but representative validation dataset annotated from the unlabeled samples. It ensures the safe model weight updating. Extensive experiments are conducted on both the public indoor and outdoor 3D object detection datasets. Results demonstrate that ProUDA has better 3D average precision than UDA and the proposed method benefits to 3D object detector training.(c) 2023 Published by Elsevier B.V.
更多查看译文
关键词
3D Object detection,Semi-supervised learning,3D Point cloud
AI 理解论文
溯源树
样例
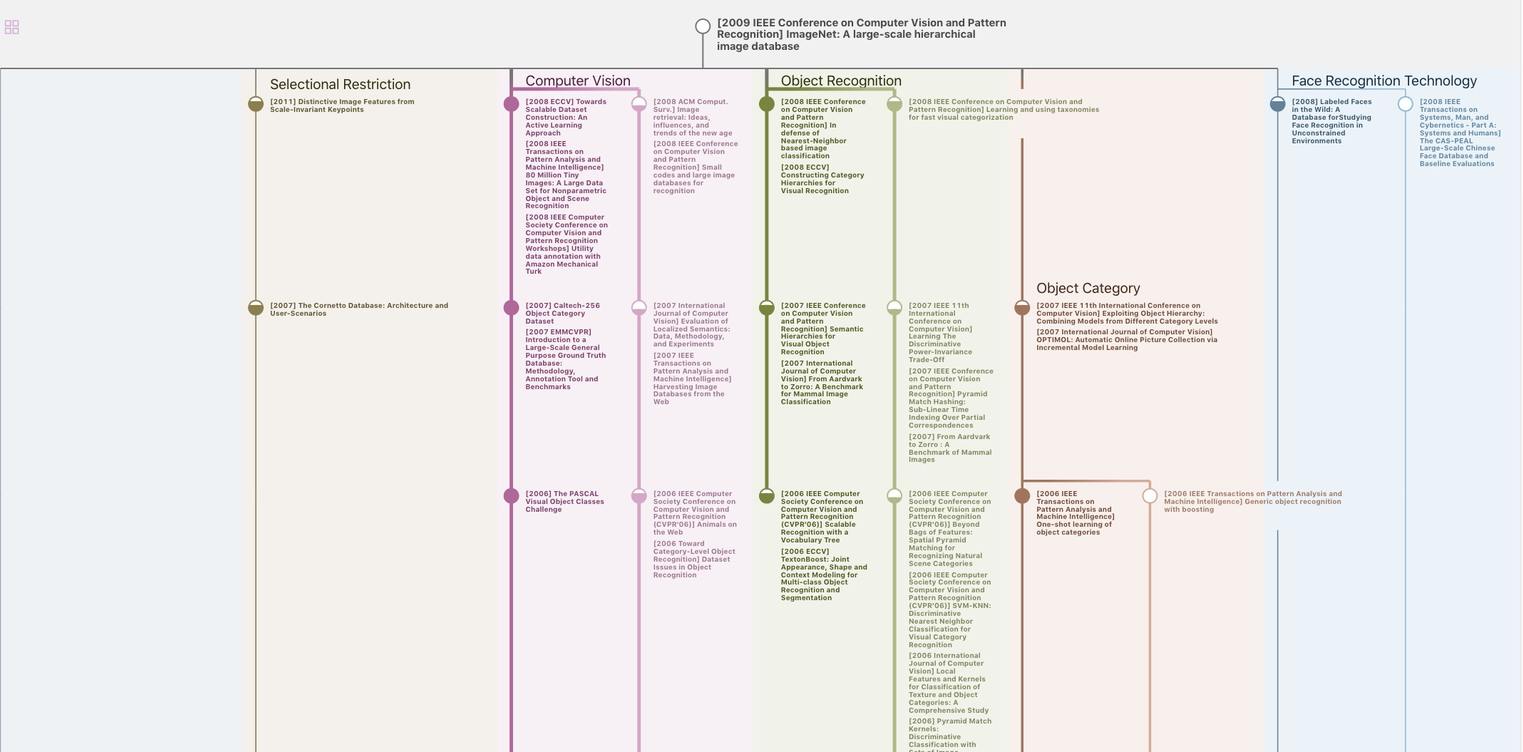
生成溯源树,研究论文发展脉络
Chat Paper
正在生成论文摘要