A deep-learning-based time of arrival estimation using kernel sparse encoding scheme.
Signal Process.(2023)
摘要
This letter proposes a deep-learning-based method for time of arrival (TOA) estimation with a new sparse encoding scheme, aiming to solve the problems caused by quantization errors and off-grid effects. The proposed method utilizes a convolutional neural network (CNN) to learn the relationship between the training signals and the TOA parameters encoded by the proposed kernel sparse representation. It en-codes true TOA values into an approximate sparse representation by the sinc-kernel function. This scheme can reserve more off-grid features of the estimated TOA parameters to enhance the optimization effi-ciency of the CNN-based network, so it can effectively minimize the quantization errors and improve the estimation performance of different off-grid distributions. Numerical simulations show that the proposed encoding model is superior to the existing encoding models under the same CNN framework, and the proposed method achieves better TOA estimation accuracy than the competitive end-to-end CNN-based estimation methods and outperforms in accuracy and speed compared with other existing off-grid esti-mation methods.(c) 2023 Elsevier B.V. All rights reserved.
更多查看译文
关键词
Time of arrival (TOA) estimation,Off-grid estimation,Deep learning,Sparse representation,Kernel encoding
AI 理解论文
溯源树
样例
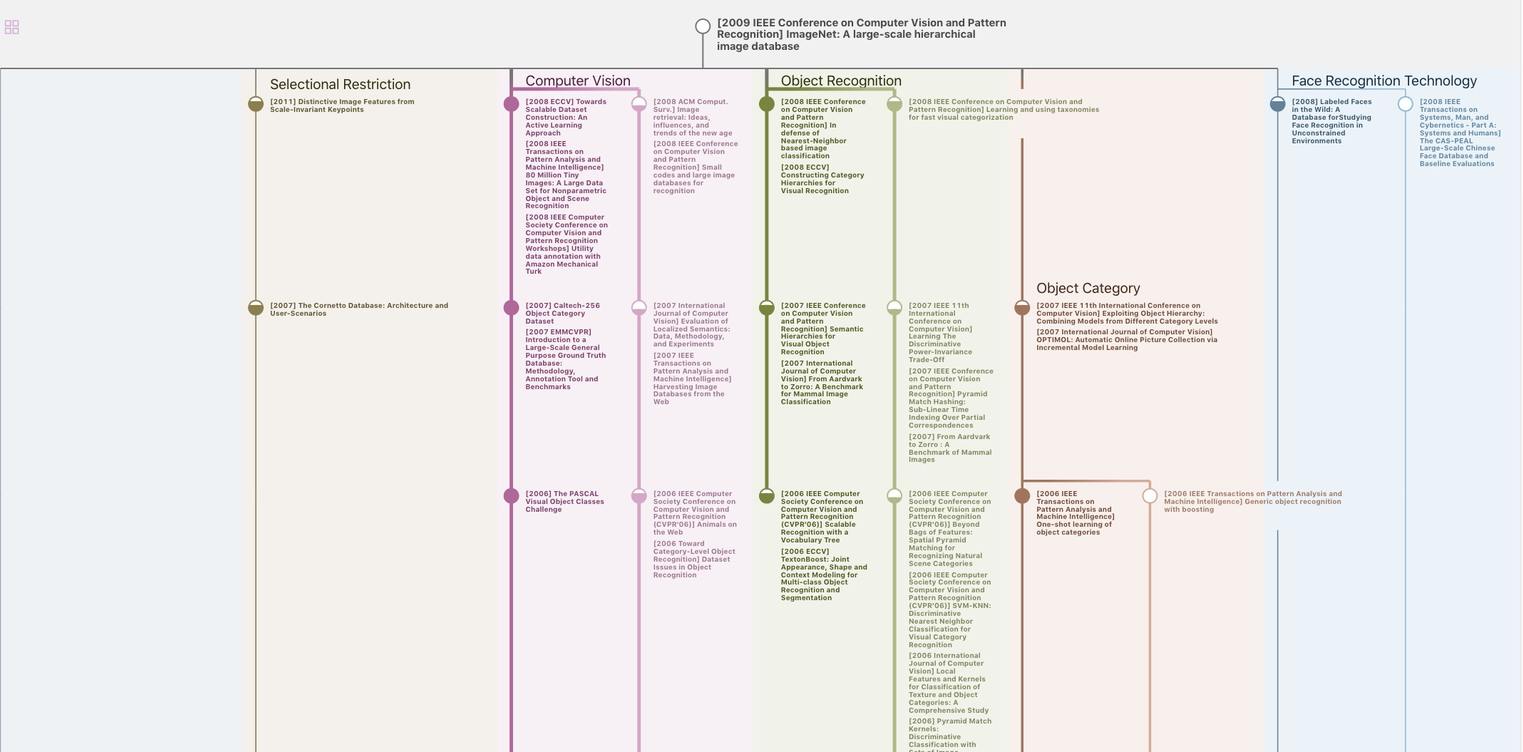
生成溯源树,研究论文发展脉络
Chat Paper
正在生成论文摘要