Efficient improvement of classification accuracy via selective test-time augmentation.
Inf. Sci.(2023)
摘要
Test-time augmentation (TTA) is typically used in image classification tasks to improve the classification accuracy of convolutional neural networks (CNNs). In the inference phase, TTA aggregates the predictions of a CNN over multiple random transformations of a query image to obtain the final prediction. However, TTA incurs a high computational cost owing to its effectiveness. In this study, we propose a selective-TTA method for reducing computational costs while maintaining the high classification accuracy. Given a query image, we quantify the predictive uncertainty of the CNN on the image to determine whether TTA should be performed. We perform TTA to obtain an enhanced prediction only when the uncertainty is high. Experimental results on benchmark datasets demonstrated that the proposed method significantly reduced average computational costs while attaining comparable or higher classification accuracy than conventional full-TTA. When the proportion of performing TTA was set to 10%, the computational cost was reduced by 90% with no performance degradation on most datasets.
更多查看译文
关键词
Image classification, Data augmentation, Test-time augmentation, Uncertainty estimation
AI 理解论文
溯源树
样例
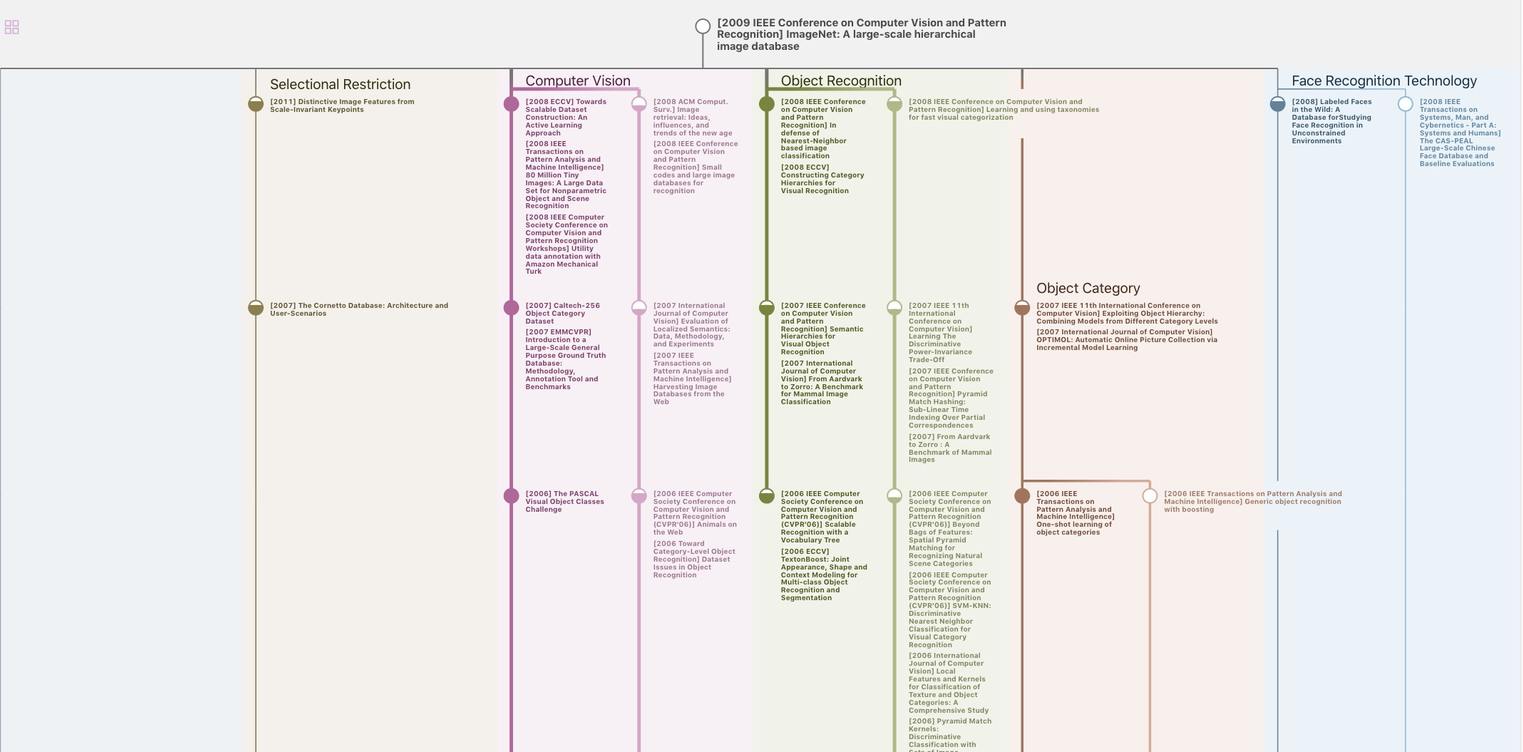
生成溯源树,研究论文发展脉络
Chat Paper
正在生成论文摘要