Trust-Region Adaptive Frequency for Online Continual Learning
Int. J. Comput. Vis.(2023)
摘要
In the paradigm of online continual learning, one neural network is exposed to a sequence of tasks, where the data arrive in an online fashion and previously seen data are not accessible. Such online fashion causes insufficient learning and severe forgetting on past tasks issues, preventing a good stability-plasticity trade-off, where ideally the network is expected to have high plasticity to adapt to new tasks well and have the stability to prevent forgetting on old tasks simultaneously. To solve these issues, we propose a trust-region adaptive frequency approach, which alternates between standard-process and intra-process updates. Specifically, the standard-process replays data stored in a coreset and interleaves the data with current data, and the intra-process updates the network parameters based on the coreset. Furthermore, to improve the unsatisfactory performance stemming from online fashion, the frequency of the intra-process is adjusted based on a trust region, which is measured by the confidence score of current data. During the intra-process, we distill the dark knowledge to retain useful learned knowledge. Moreover, to store more representative data in the coreset, a confidence-based coreset selection is presented in an online manner. The experimental results on standard benchmarks show that the proposed method significantly outperforms state-of-art continual learning algorithms.
更多查看译文
关键词
Online continual learning,Catastrophic forgetting,Trust-region,Deep learning
AI 理解论文
溯源树
样例
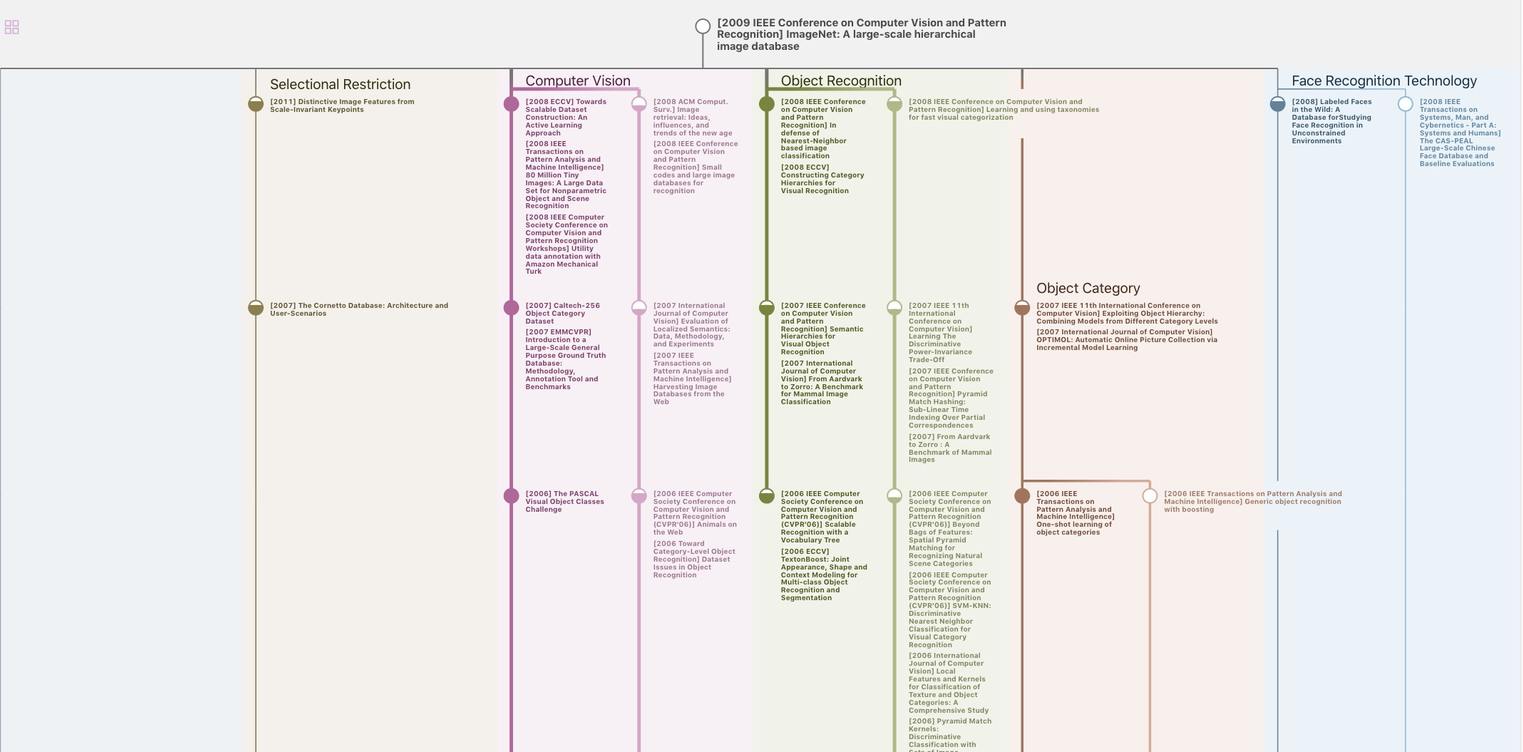
生成溯源树,研究论文发展脉络
Chat Paper
正在生成论文摘要