A Statistical Dependence Framework Based on a Multivariate Normal Copula Function and Stochastic Differential Equations for Multivariate Data in Forestry.
Axioms(2023)
摘要
Stochastic differential equations and Copula theories are important topics that have many advantages for applications in almost every discipline. Many studies in forestry collect longitudinal, multi-dimensional, and discrete data for which the amount of measurement of individual variables does not match. For example, during sampling experiments, the diameters of all trees, the heights of approximately 10% of the trees, and the tree crown base height and crown width for a significantly smaller number of trees are measured. In this study, for estimating five-dimensional dependencies, we used a normal copula approach, where the dynamics of individual tree variables (diameter, potentially available area, height, crown base height, and crown width) are described by a stochastic differential equation with mixed-effect parameters. The approximate maximum likelihood method was used to obtain parameter estimates of the presented stochastic differential equations, and the normal copula dependence parameters were estimated using the pseudo-maximum likelihood method. This study introduced the normalized multi-dimensional interaction information index based on differential entropy to capture dependencies between state variables. Using conditional copula-type probability density functions, the exact form equations defining the links among the diameter, potentially available area, height, crown base height, and crown width were derived. All results were implemented in the symbolic algebra system MAPLE.
更多查看译文
关键词
normal copula, stochastic differential equation, marginal distributions, conditional distributions, entropy, normalized interaction information
AI 理解论文
溯源树
样例
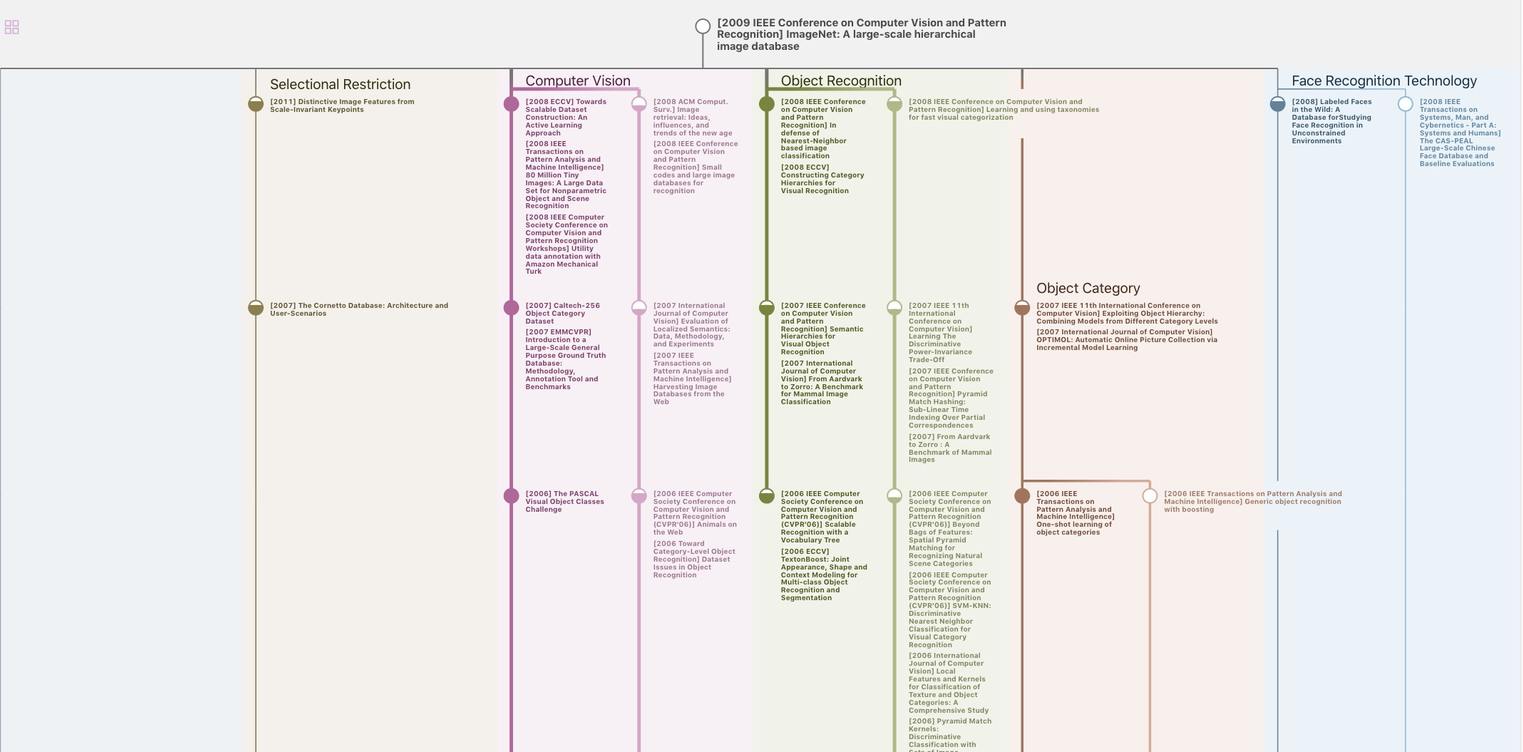
生成溯源树,研究论文发展脉络
Chat Paper
正在生成论文摘要