Swarm UAV networking with collaborative beamforming and automated ESN learning in the presence of unknown blockages.
Comput. Networks(2023)
摘要
This paper aims at designing high-data-rate swarm UAV networks with distributed beamforming capabilities. The primary challenge is that the beamforming gain in swarm UAV networks is highly affected by the UAVs’ flight altitude, their movements and the resulting intermittent link blockages, as well as the availability of channel state information (CSI) at individual UAVs. To address this challenge, we propose FlyBeam, a learning-based framework for joint flight and beamforming control in swarm UAV networks. We first present a mathematical formulation of the control problem with the objective of maximizing the throughput of swarm UAV networks by jointly controlling the flight and distributed beamforming of UAVs. Then, a distributed solution algorithm is designed based on a combination of Echo State Network (ESN) learning and online reinforcement learning. The former is adopted to approximate the utility function for individual UAVs based on online measurements, by jointly considering the unknown blockage dynamics and other factors that affect the beamforming gain. The latter is used to guide the exploitation and exploration in FlyBeam. We further design a scheme referred to as AutoESN to automate the training of the ESN model. AutoESN can update the configurable ESN parameters automatically using a combination of loss function and step size. The effectiveness of FlyBeam is evaluated through an extensive simulation campaign on UBSim, a Python-based Universal Broadband Simulator for integrated aerial and ground wireless networking. The performance of FlyBeam is compared with two benchmark schemes, one designed based on traditional optimization techniques and the other based on learning with utility function approximation through Long Short-Term Memory (LSTM). In the performance evaluation, we consider both Zero-Forcing (ZF) and Maximum Ratio Transfer (MRT) for beamforming with sequential and simultaneous channel state estimation. It is shown that significant (up to 450%) beamforming gain can be achieved by FlyBeam. We also investigate the effects of blockages and UAV flight altitude on the beamforming gain. It is found that, somewhat surprisingly, higher (rather than lower) beamforming gain can be achieved by FlyBeam with denser blockages in swarm UAV networks.
更多查看译文
关键词
collaborative beamforming,esn
AI 理解论文
溯源树
样例
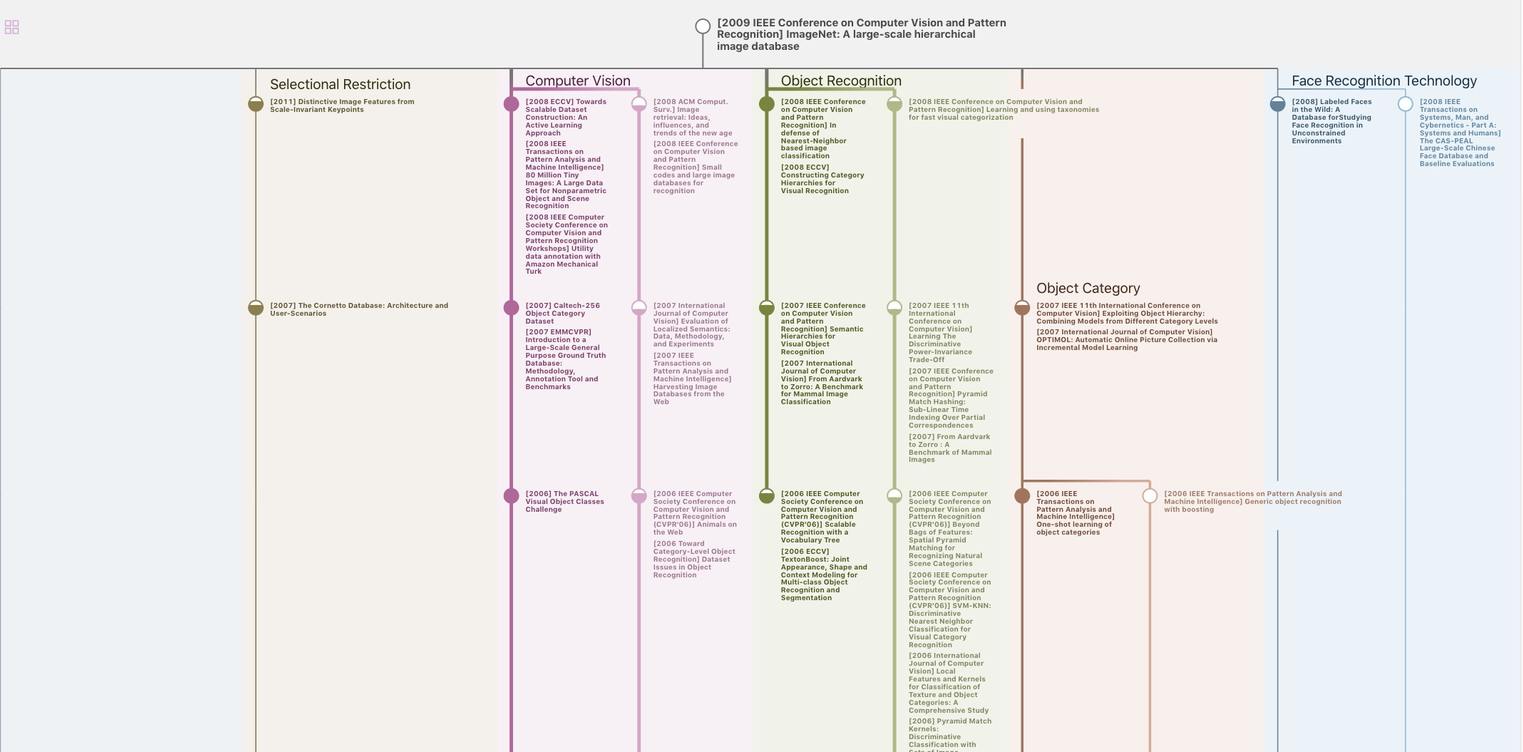
生成溯源树,研究论文发展脉络
Chat Paper
正在生成论文摘要