Pose Graph Relocalization with Deep Object Detection and BIM-Supported Object Landmark Dictionary
J. Comput. Civ. Eng.(2023)
摘要
Indoor localization is a prerequisite for autonomous robot applications in the construction industry. However, traditional localization techniques rely on low-level features and do not exploit construction-related semantics. They also are sensitive to environmental factors such as illumination and reflection rate, and therefore suffer from unexpected drifts and failures. This study proposes a pose graph relocalization framework that utilizes object-level landmarks to enhance a traditional visual localization system. The proposed framework builds an object landmark dictionary from Building Information Model (BIM) as prior knowledge. Then a multimodal deep neural network (DNN) is proposed to realize 3D object detection in real time, followed by instance-level object association with false-positive rejection, and relative pose estimation with outlier removal. Finally, a keyframe-based graph optimization is performed to rectify the drifts of traditional visual localization. The proposed framework was validated using a mobile platform with red-green-blue-depth (RGB-D) and inertial sensors, and the test scene was an indoor office environment with furnishing elements. The object detection model achieved 62.9% mean average precision (mAP). The relocalization technique reduced translational drifts by 64.67% and rotational drifts by 41.59% compared with traditional visual-inertial odometry.
更多查看译文
关键词
pose graph relocalization,deep object detection,landmark,bim-supported
AI 理解论文
溯源树
样例
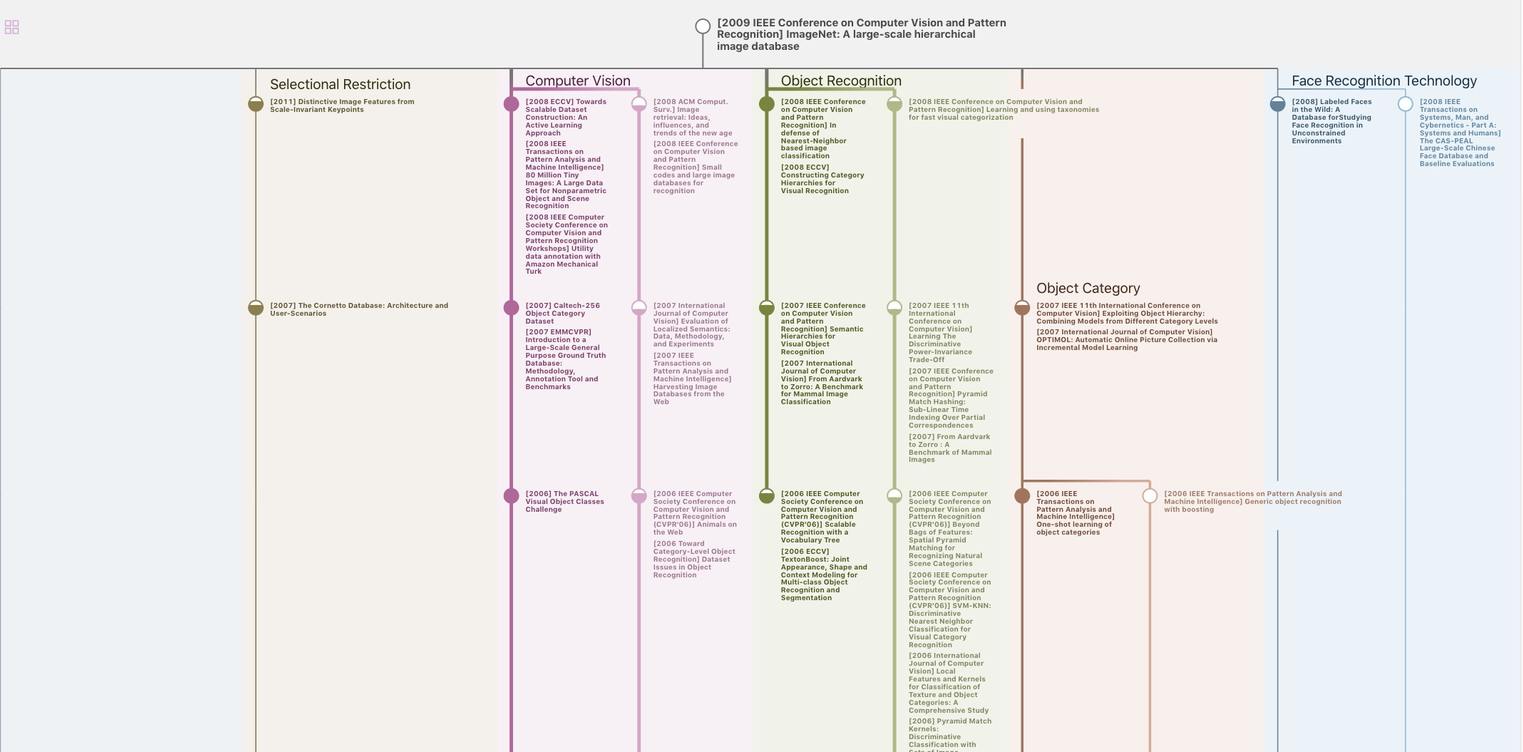
生成溯源树,研究论文发展脉络
Chat Paper
正在生成论文摘要