Toward Accurate and Efficient Road Extraction by Leveraging the Characteristics of Road Shapes.
IEEE Trans. Geosci. Remote. Sens.(2023)
Abstract
Automatically extracting roads from very high-resolution (VHR) remote sensing images is of great importance in a wide range of remote sensing applications. However, complex shapes of roads (i.e., long, geometrically deformed, and thin) always affected the extraction accuracy, which is one of the challenges of road extraction. Based on the insight into road shape characteristics, we propose a novel road shape-aware network (RSANet) to achieve efficient and accurate road extraction. First, we introduce the efficient strip transformer module (ESTM) to efficiently capture the global context to model the long-distance dependence required by long roads. Second, we design a geometric deformation estimation module (GDEM) to adaptively extract the context from the shape deformation caused by shooting roads from different perspectives. Third, we provide a simple but effective road edge focal loss (REF loss) to make the network focus on optimizing the pixels around the road to alleviate the unbalanced distribution of foreground and background pixels caused by the roads being too thin. Finally, we conduct extensive evaluations on public datasets to verify the effectiveness of RSANet and each of the proposed components. Experiments validate that our RSANet outperforms state-of-the-art methods for road extraction in remote sensing images.
MoreTranslated text
Key words
efficient road extraction,road shapes
AI Read Science
Must-Reading Tree
Example
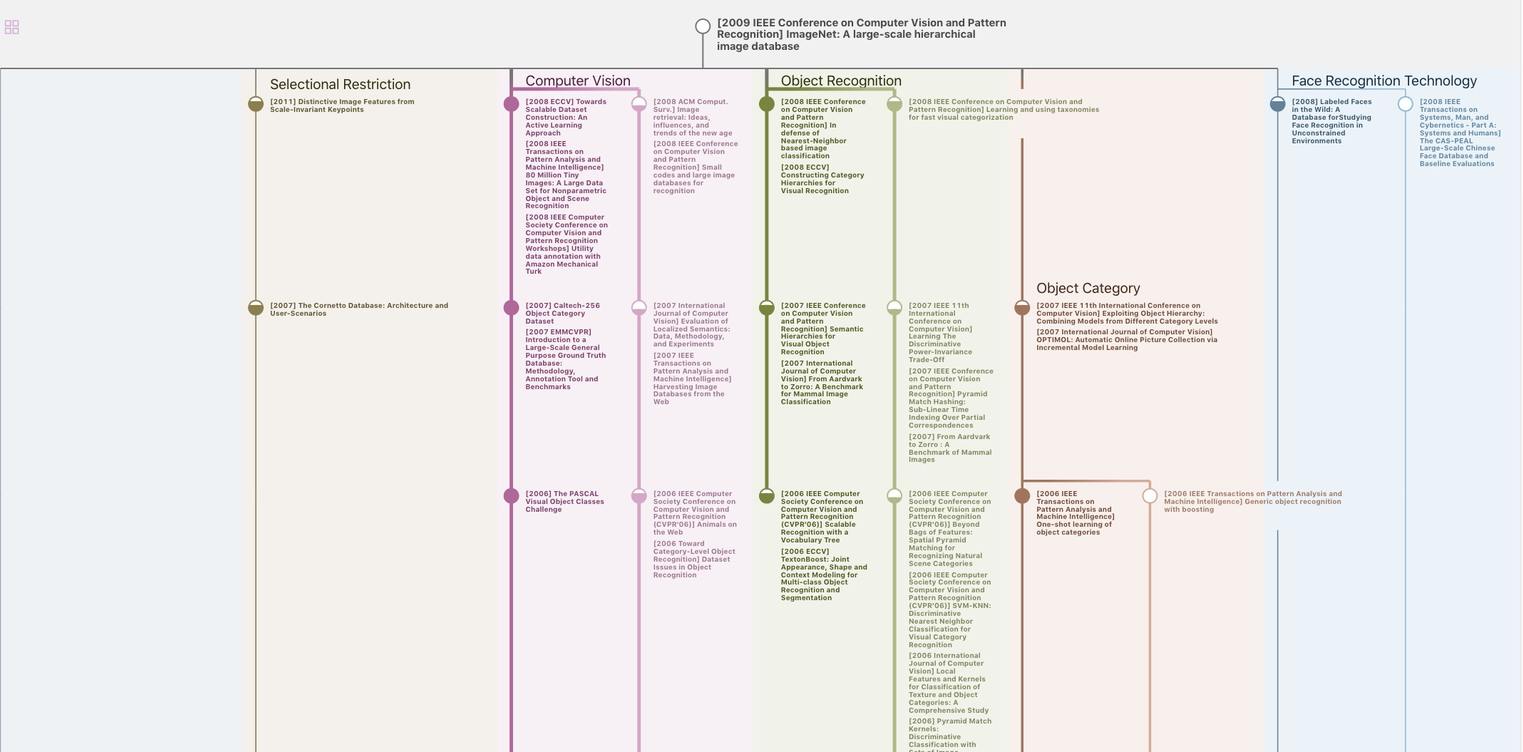
Generate MRT to find the research sequence of this paper
Chat Paper
Summary is being generated by the instructions you defined