Recovering Gravity from Satellite Altimetry Data Using Deep Learning Network.
IEEE Trans. Geosci. Remote. Sens.(2023)
摘要
The satellite altimetry missions could measure high-accuracy sea surface heights (SSHs) that can be used to recover the marine gravity field. Traditional methods for estimating the marine gravity field from SSHs all rely on approximate physical correlations between SSHs and gravity, which may neglect nature's complex nonlinearity. This work presents a new deep network-based method to recover the gravity anomaly. This new method uses a multichannel convolutional neural network (MCCNN) architecture to capture the nonlinear features between ship-borne gravity and a group of input parameters including deflections of the vertical (DOVs), submarine topography, and the geo-locations. To validate the gravity, ship-borne gravity anomalies on the two independent cruises were not used in the deep learning process. For comparison, we also estimated the gravity using the traditional inverse Vening Meinesz (IVM) method. Our results indicate that the MCCNN method can derive high-quality marine gravity anomalies. The assessments using 1-mGal-accuracy ship-borne gravity anomalies show that the average accuracy for gravity from the MCCNN method is higher than 3 mGal and this method achieves 0.05-0.50 mGal improvement over benchmark methods IVM. Assessed by marine gravity anomaly models with the accuracy of 1-2 mGal, the MCCNN method has been shown to improve the accuracy of gravity by at least 4%. Comparisons with the IVM results show that improvements in the MCCNN method were mainly in wavelengths between 8 and 100 km due to the use of bathymetry. The results show that our deep learning method maintains good performance and is promising for gravity recovery.
更多查看译文
关键词
satellite altimetry data,deep learning,gravity
AI 理解论文
溯源树
样例
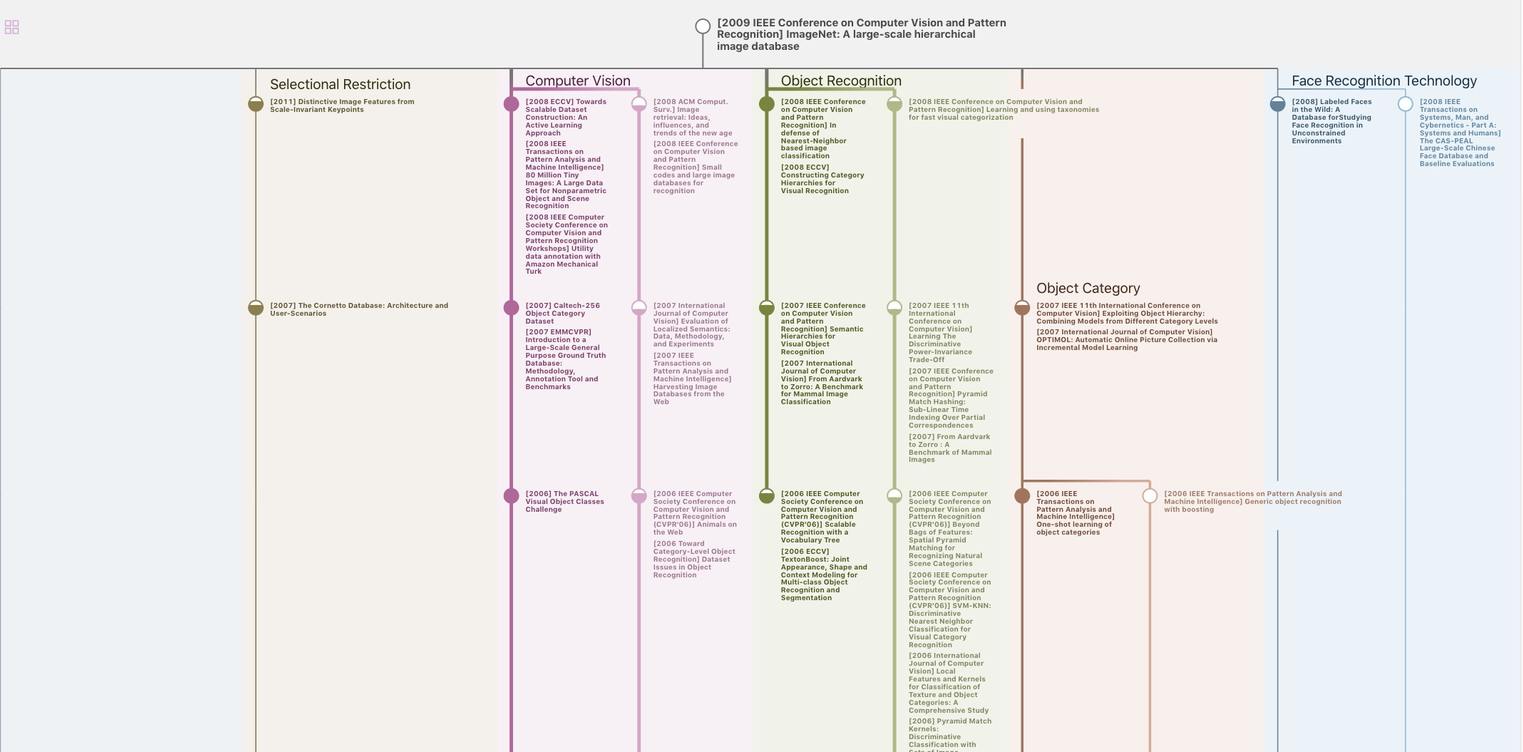
生成溯源树,研究论文发展脉络
Chat Paper
正在生成论文摘要