Hyperspectral Image Super-Resolution via Learning an Undercomplete Dictionary and Intra-Algorithmic Postprocessing.
IEEE Trans. Geosci. Remote. Sens.(2023)
摘要
This study proposes a novel hyperspectral image super-resolution (HSI-SR) method, whose fundamental requirement to set up is only a single low-resolution hyperspectral image (LR-HSI) of the target scene. To fully exploit the inherent features of hyperspectral images (HSIs), including spectral correlation, local sparsity, nonlocal self-similarity, and piecewise smoothness in the spatial domain, we first break down the HSI-SR problem into several identical subcube SR (SC-SR) problems. Then, we design an SC-SR modular model involving two parts: 1) injecting the spatio-spectral information from co-structural subcube patches (SCPs) into each other's sparse representation via learning an undercomplete dictionary and 2) intra-algorithmic postprocessing. The second part, which makes the most of the spatial piecewise smoothness characteristic, consists of two modules, wavelet-based spatial smoothing (SpaS) and adaptive filtering (AF). After merging all the super-resolved SCs and obtaining a high-resolution HSI, we use spectral smoothing (SpeS) to remove the possible remaining distortions in the spectral vector of each pixel. Experiments on the simulated- and real-LR datasets demonstrate the outperformance of our method.
更多查看译文
关键词
undercomplete dictionary,learning,super-resolution,intra-algorithmic,post-processing
AI 理解论文
溯源树
样例
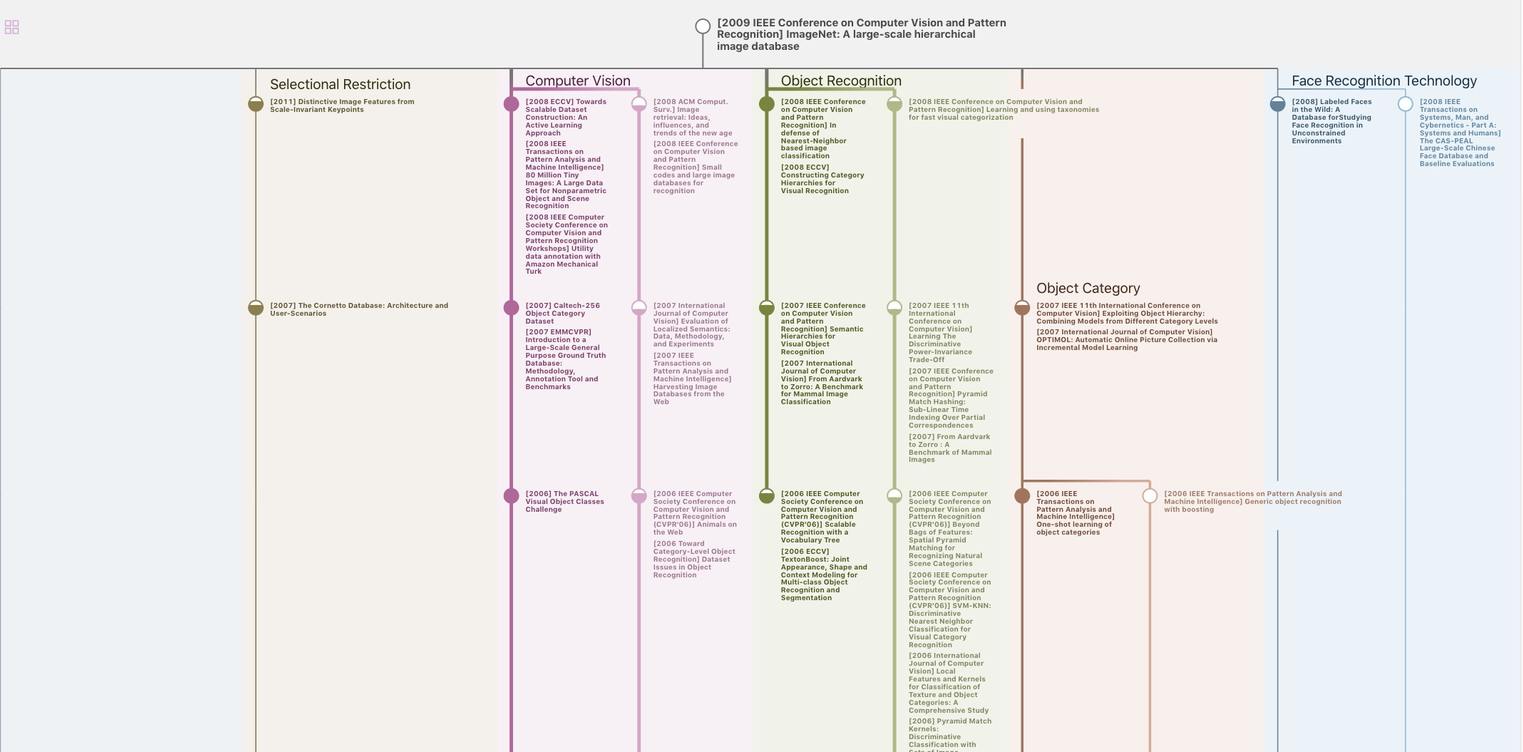
生成溯源树,研究论文发展脉络
Chat Paper
正在生成论文摘要