Combination of Snow Process Model Priors and Site Representativeness Evaluation to Improve the Global Snow Depth Retrieval Based on Passive Microwaves
IEEE Trans. Geosci. Remote. Sens.(2023)
摘要
The spatiotemporal distribution of snow depth (SD) has a significant impact on the energy and water balances of the Earth's system. However, passive microwave (PMW) remote sensing widely used for SD estimation has large uncertainties due to the variations in snow physical properties. In this study, we demonstrate a new method to minimize these uncertainties and to increase the accuracy of SD estimation. Our method is based on the synergy between the PMW AMSR2 brightness temperature (T-B) and a physical snow process model [snow thermal model (SNTHERM)] to estimate snow grain size, snow density, and first-guess SD as priors. On the one hand, we used TB from three frequencies and removed nonrepresentative ground measurements at the stations to improve the deep snow estimation. Then, we applied a machine learning (ML) algorithm based on both the AMSR2 TB and the SNTHERM simulations to retrieve the global SD. The results showed that the root mean-squared error (RMSE) of the retrieved SD was 12.4 cm at the meteorological stations. Independent validations showed that our method significantly reduced the SD and snow water equivalent (SWE) underestimation in the mountains compared to the current satellite products.
更多查看译文
关键词
Snow,Pollution measurement,Scattering,Microwave measurement,Temperature measurement,Particle measurements,Microwave theory and techniques,Passive microwave (PMW) remote sensing,snow depth (SD),snow process model,snow water equivalent (SWE)
AI 理解论文
溯源树
样例
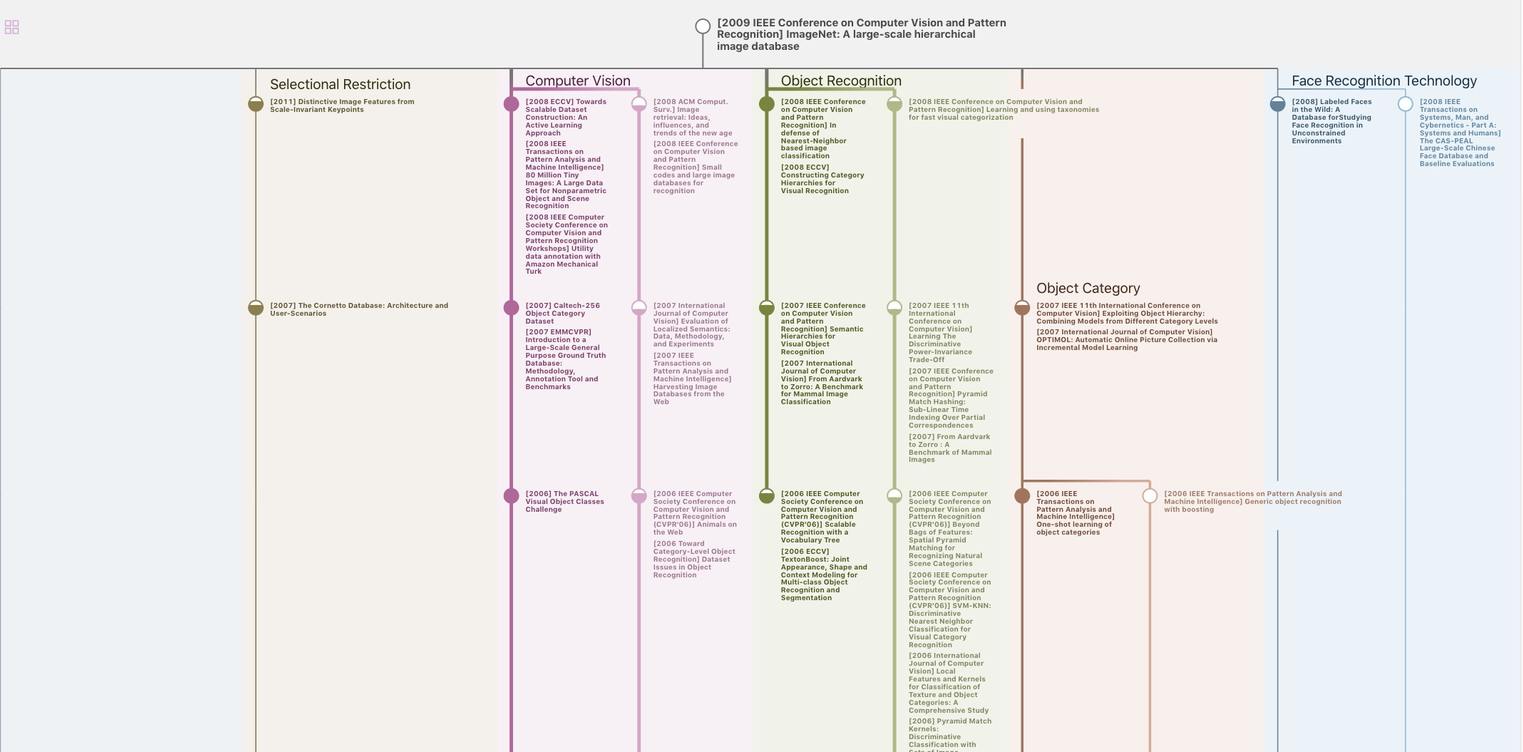
生成溯源树,研究论文发展脉络
Chat Paper
正在生成论文摘要