CLEGAN: Toward Low-Light Image Enhancement for UAVs via Self-Similarity Exploitation
IEEE Trans. Geosci. Remote. Sens.(2023)
摘要
Low-light remote sensing image enhancement for unmanned aerial vehicles (UAVs) has significant scientific and practical value because unfavorable lighting conditions make capture more difficult, resulting in undesired images. As acquiring real-world low-light/normal-light image pairs in the field of remote sensing is almost infeasible, performing low-light image enhancement (LLIE) in an unpaired manner is practical and valuable. However, without the paired data as the supervision, learning an LLIE network is challenging. To address the challenges, this article proposes a novel yet effective method to unpaired LLIE, which maximizes the mutual information between low-light and restored images through self-similarity contrastive learning (SSCL) in a fully unsupervised fashion within a single deep generative adversarial network (GAN) framework, named CLEGAN. Instead of supervising the learning using ground truth data, we propose to regularize the unpaired training using the information extracted from the input itself. The nonlocal patch sampling strategy in SSCL naturally makes the negative samples differ from the positive samples for discriminative representation. Moreover, the single GAN embeds the dual illumination perception module (DIPM) to handle the internal recurrence of information and overall uneven illumination distribution in remote sensing images. DIPM mainly consists of two cooperative blocks: the spatial adaptive light adjustment module (SALAM) and the global adaptive light adjustment module (GALAM). Specifically, SALAM exploits the internal recurrence of information in remote sensing images to encode a wider range of contextual information into local features and make a proper light estimation. Simultaneously, GALAM enhances the most valuable illumination-related channels in the feature map to achieve better light estimation. The experiments on several datasets including low-light remote sensing image dataset and public low-light image datasets show that CLEGAN performs favorably against the existing unpaired LLIE approaches, and even outperforms several fully supervised methods.
更多查看译文
关键词
Contrastive learning (CL),dual illumination perception module (DIPM),low-light image enhancement (LLIE),unmanned aerial vehicle (UAV),unsupervised learning
AI 理解论文
溯源树
样例
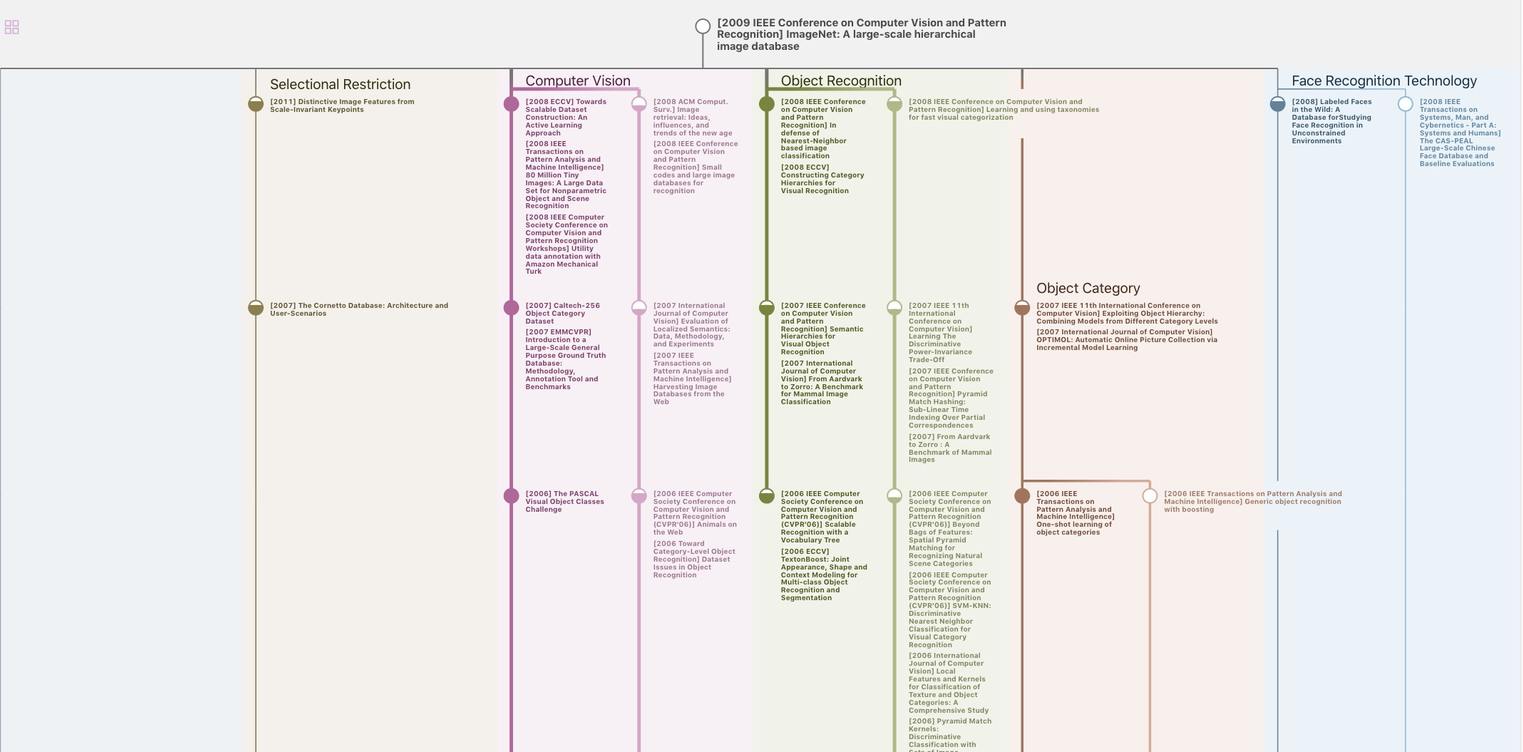
生成溯源树,研究论文发展脉络
Chat Paper
正在生成论文摘要