Learning MLatent Representations for Generalized Zero-Shot Learning
IEEE Trans. Multim.(2023)
摘要
In generative adversarial network (GAN) based zero-shot learning (ZSL) approaches, the synthesized unseen visual features are inevitably prone to seen classes since the feature generator is merely trained on seen references, which causes the inconsistency between visual features and their corresponding semantic attributes. This visual-semantic inconsistency is primarily induced by the non-preserved semantic-relevant components and the non-rectified semantic-irrelevant low-level visual details. Existing generative models generally tackle the issue by aligning the distribution of the two modalities with an additional visual-to-semantic embedding, which tends to cause the hubness problem and ruin the diversity of visual modality. In this paper, we propose a novel generative model named learning modality-consistent latent representations GAN (LCR-GAN) to address the problem via embedding the visual features and their semantic attributes into a shared latent space. Specifically, to preserve the semantic-relevant components, the distributions of the two modalities are aligned by maximizing the mutual information between them. And to rectify the semantic-irrelevant visual details, the mutual information between original visual features and their latent representations is confined within an appropriate range. Meanwhile, the latent representations are decoded back to both modalities to further preserve the semantic-relevant components. Extensive evaluations on four public ZSL benchmarks validate the superiority of our method over other state-of-the-art methods.
更多查看译文
关键词
Generative adversarial network,latent representations,mutual information,semantic-relevant,semantic-irrelevant,zero-shot learning
AI 理解论文
溯源树
样例
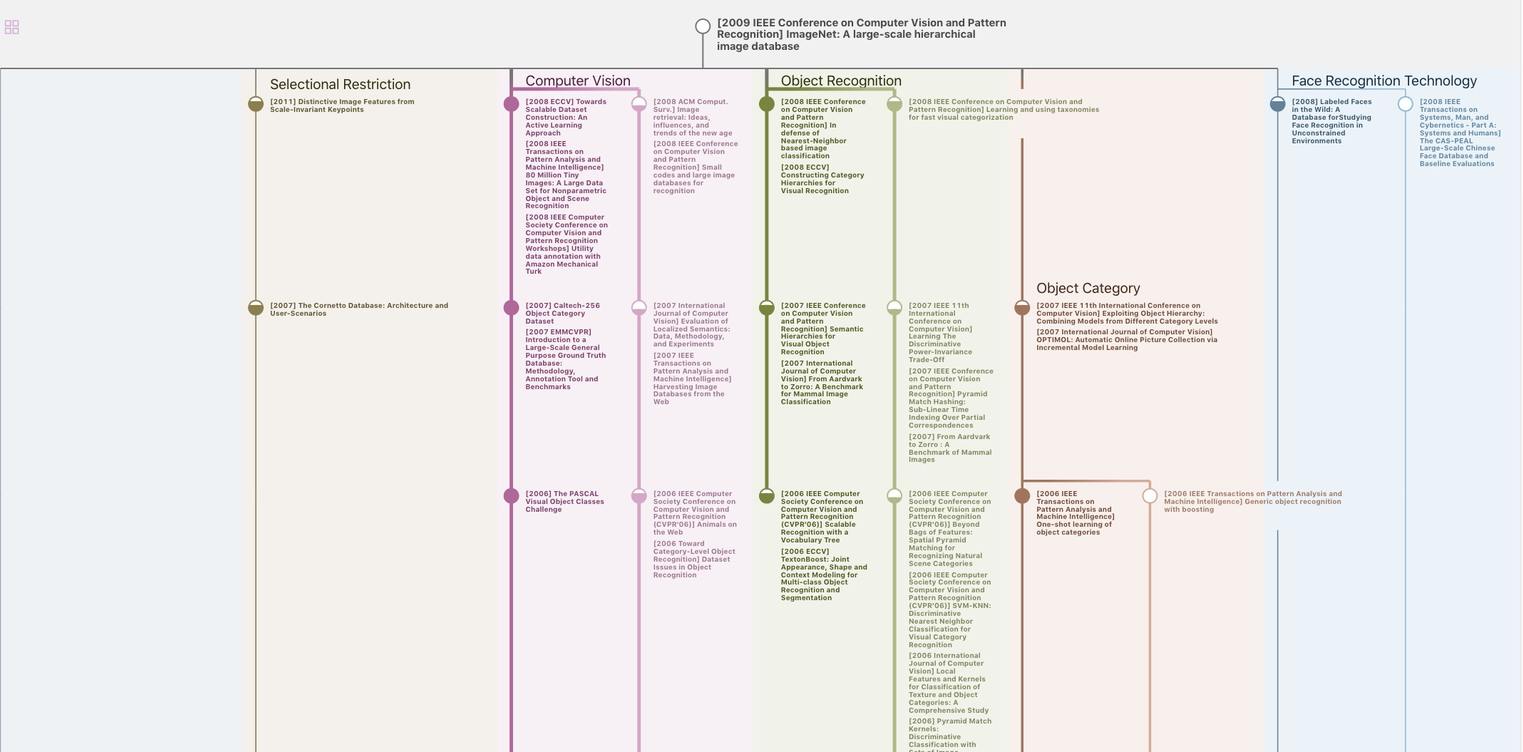
生成溯源树,研究论文发展脉络
Chat Paper
正在生成论文摘要