On Batch Teaching Without Collusion
JOURNAL OF MACHINE LEARNING RESEARCH(2022)
摘要
Formal models of learning from teachers need to respect certain criteria to avoid collusion. The most commonly accepted notion of collusion-avoidance was proposed by Goldman and Mathias (1996), and various teaching models obeying their criterion have been studied. For each model M and each concept class C, a parameter M-TD(C) refers to the teaching dimension of concept class C in model M-defined to be the number of examples required for teaching a concept, in the worst case over all concepts in C. This paper introduces a new model of teaching, called no-clash teaching, together with the corresponding parameter NCTD(C). No-clash teaching is provably optimal in the strong sense that, given any concept class C and any model M obeying Goldman and Mathias's collusion-avoidance criterion, one obtains NCTD(C) <= M-TD(C). We also study a corresponding notion NCTD+ for the case of learning from positive data only, establish useful bounds on NCTD and NCTD+, and discuss relations of these parameters to other complexity parameters of interest in computational learning theory. We further argue that Goldman and Mathias's collusion-avoidance criterion may in some set-tings be too weak in that it admits certain forms of interaction between teacher and learner that could be considered collusion in practice. Therefore, we introduce a strictly stronger notion of collusion-avoidance and demonstrate that the well-studied notion of Preference-based Teaching is optimal among all teaching schemes that are strongly collusion-avoiding on all finite subsets of a given concept class.
更多查看译文
关键词
machine teaching,collusion-freeness,VC dimension,teaching dimension,sample compression
AI 理解论文
溯源树
样例
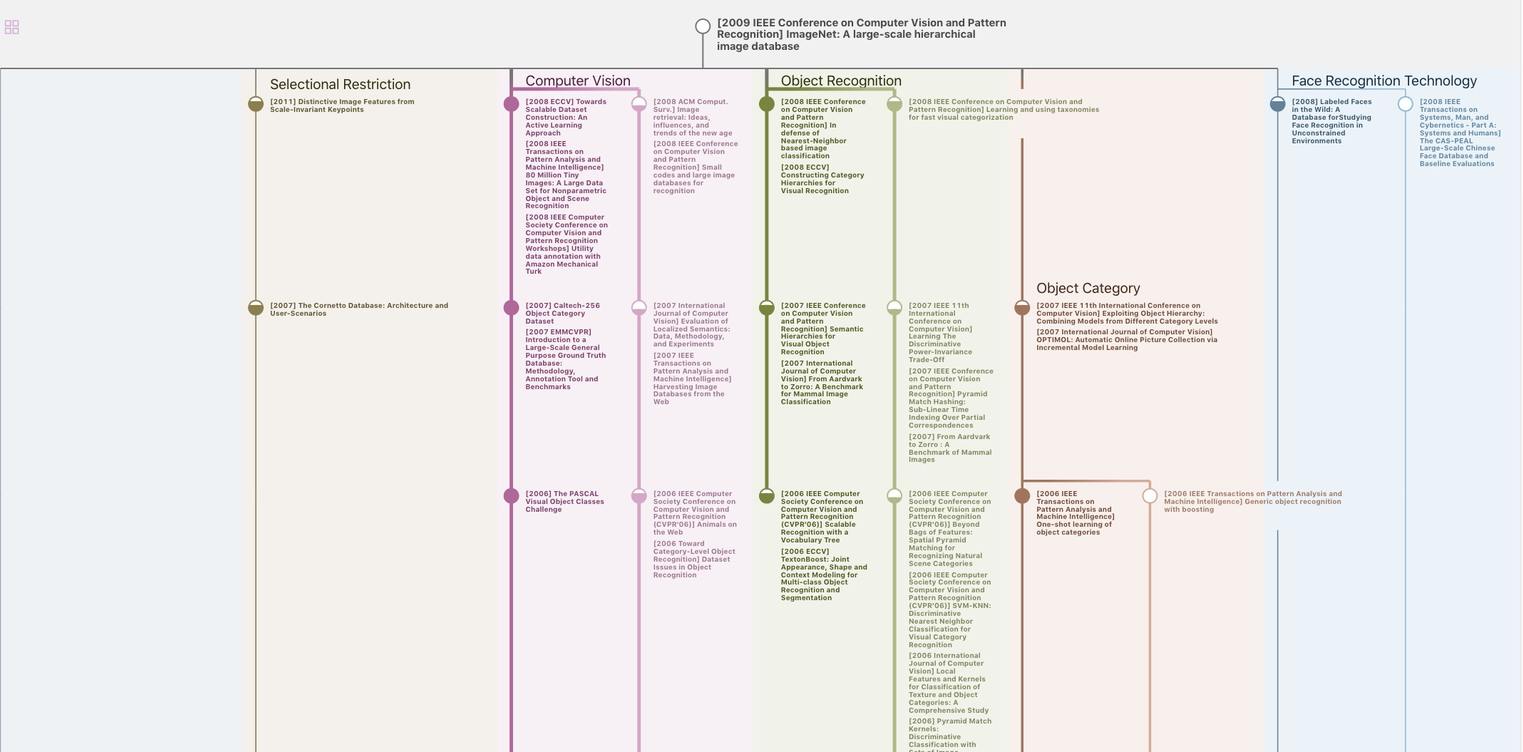
生成溯源树,研究论文发展脉络
Chat Paper
正在生成论文摘要