Two-mode Networks: Inference with as Many Parameters as Actors and Differential Privacy.
J. Mach. Learn. Res.(2022)
摘要
Many network data encountered are two-mode networks. These networks are characterized by having two sets of nodes and links are only made between nodes belonging to different sets. While their two-mode feature triggers interesting interactions, it also increases the risk of privacy exposure, and it is essential to protect sensitive information from being disclosed when releasing these data. In this paper, we introduce a weak notion of edge differential privacy and propose to release the degree sequence of a two-mode network by adding non-negative Laplacian noises that satisfies this privacy definition. Under mild conditions for an exponential-family model for bipartite graphs in which each node is individually parameterized, we establish the consistency and asymptotic normality of two differential privacy estimators, the first based on moment equations and the second after denoising the noisy sequence. For the latter, we develop an efficient algorithm which produces a readily useful synthetic bipartite graph. Numerical simulations and a real data application are carried out to verify our theoretical results and demonstrate the usefulness of our proposal.
更多查看译文
关键词
Asymptotic normality, Consistency, Differential privacy, Synthetic graph, Two-mode network
AI 理解论文
溯源树
样例
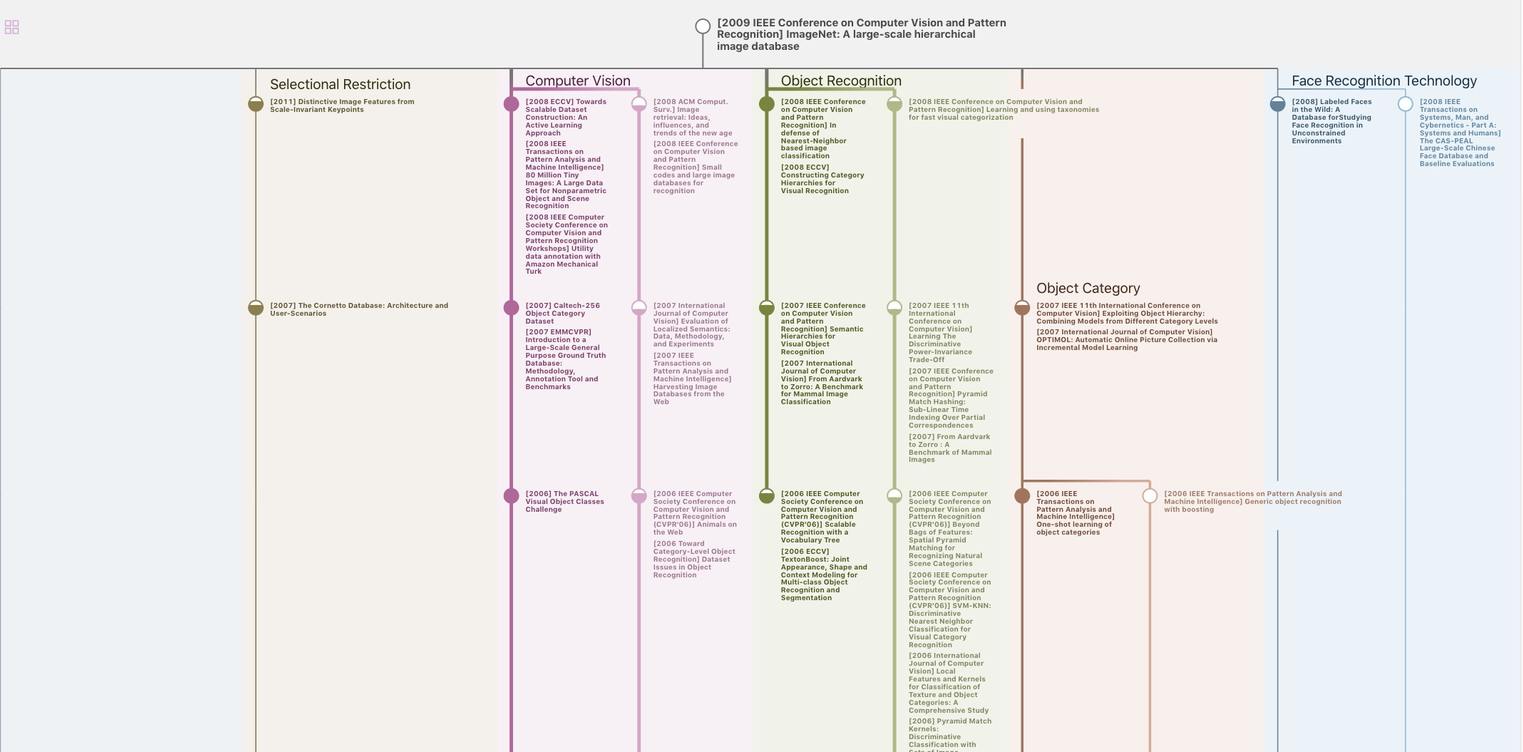
生成溯源树,研究论文发展脉络
Chat Paper
正在生成论文摘要