A Wasserstein Distance Approach for Concentration of Empirical Risk Estimates
J. Mach. Learn. Res.(2022)
摘要
This paper presents a unified approach based on Wasserstein distance to derive concentration bounds for empirical estimates for two broad classes of risk measures defined in the paper. The classes of risk measures introduced include as special cases well known risk measures from the finance literature such as conditional value at risk (CVaR), optimized certainty equivalent risk, spectral risk measures, utility-based shortfall risk, cumulative prospect theory (CPT) value, rank dependent expected utility and distorted risk measures. Two estimation schemes are considered, one for each class of risk measures. One estimation scheme involves applying the risk measure to the empirical distribution function formed from a collection of i.i.d. samples of the random vari-able (r.v.), while the second scheme involves applying the same procedure to a truncated sample. The bounds provided apply to three popular classes of distributions, namely sub-Gaussian, sub -exponential and heavy-tailed distributions. The bounds are derived by first relating the estimation error to the Wasserstein distance between the true and empirical distributions, and then using recent concentration bounds for the latter. Previous concentration bounds are available only for specific risk measures such as CVaR and CPT-value. The bounds derived in this paper are shown to ei-ther match or improve upon previous bounds in cases where they are available. The usefulness of the bounds is illustrated through an algorithm and the corresponding regret bound for a stochastic bandit problem involving a general risk measure from each of the two classes introduced in the paper.
更多查看译文
关键词
Concentration bounds, Wasserstein distance, conditional value-at-risk, optimized cer-tainty equivalent risk, spectral risk measure, utility-based shortfall risk, cumulative prospect theory, rank-dependent expected utility, distorted risk measures, risk-sensitive bandits
AI 理解论文
溯源树
样例
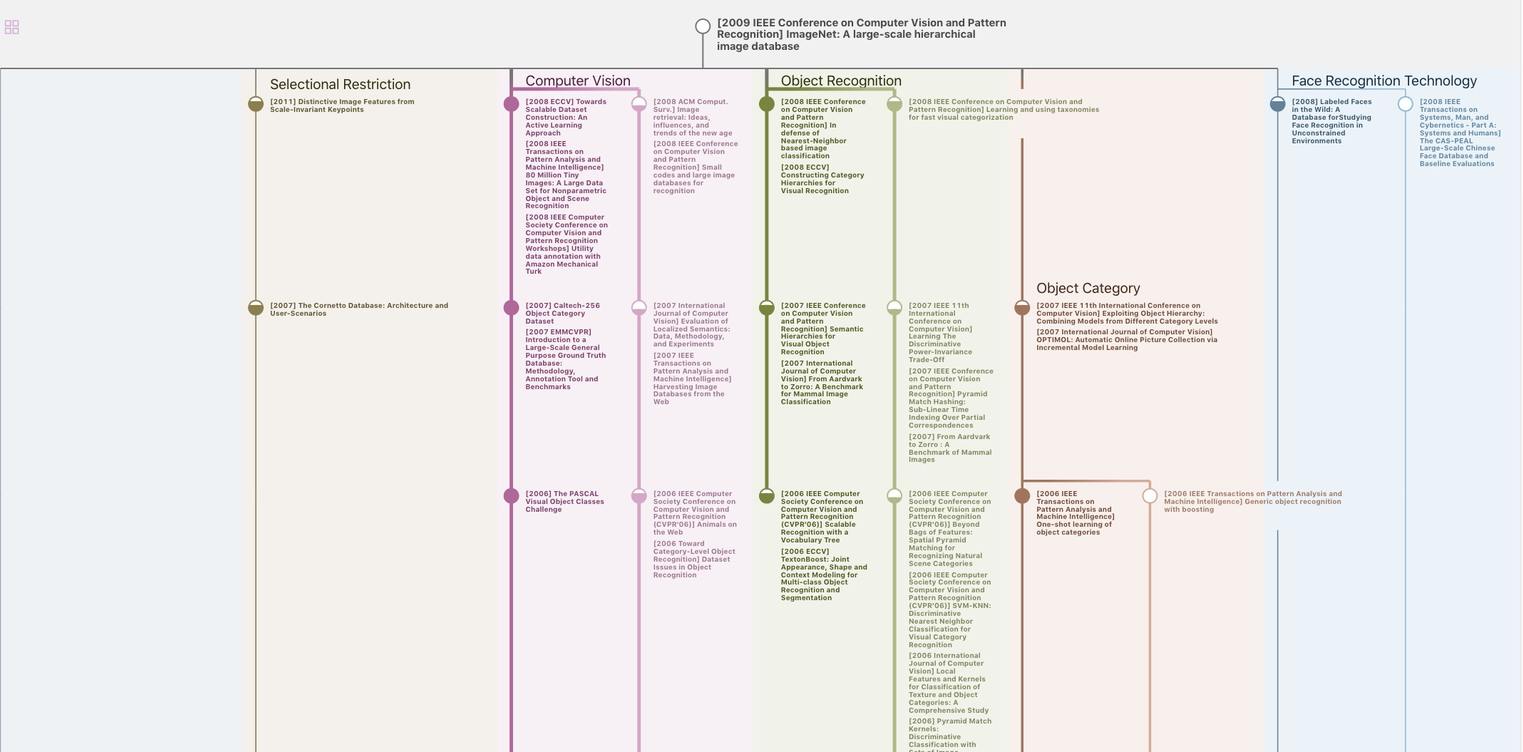
生成溯源树,研究论文发展脉络
Chat Paper
正在生成论文摘要