Optimal machine learning methods for prediction of high-flow nasal cannula outcomes using image features from electrical impedance tomography.
Comput. Methods Programs Biomed.(2023)
摘要
•Electrical impedance tomography (EIT) and machine learning methods are combined to predict High-flow nasal cannula (HFNC) outcomes promptly by EIT image features.•For the balanced data proceeded by the synthetic minority oversampling technique, the specificity of KNN, xgboost, random forest, GBDT, bernoulli bayes and AdaBoost significantly reduced (p<0.05) while the area under curve did not improve considerably (p>0.05); and the accuracy and recall decreased significantly (p<0.05).•The xgboost method showed better overall performance for balanced EIT image features, which may be considered as the ideal machine learning method for early prediction of HFNC outcomes.
更多查看译文
关键词
High-flow nasal cannula,Electrical impedance tomography,Image features,Early prediction,Machine learning
AI 理解论文
溯源树
样例
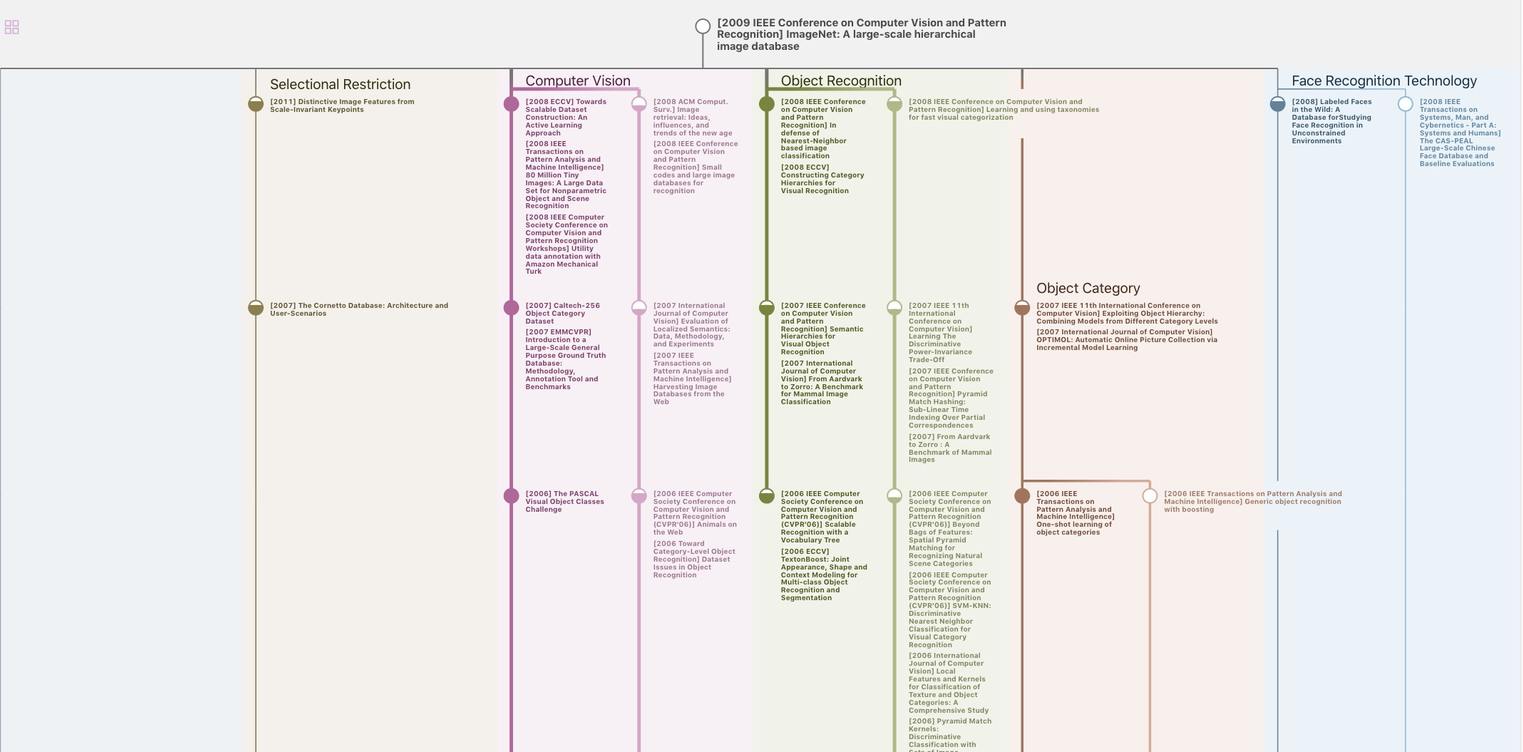
生成溯源树,研究论文发展脉络
Chat Paper
正在生成论文摘要