Classification of health deterioration by geometric invariants.
Comput. Methods Programs Biomed.(2023)
摘要
BACKGROUND AND OBJECTIVES:Prediction of patient deterioration is essential in medical care, and its automation may reduce the risk of patient death. The precise monitoring of a patient's medical state requires devices placed on the body, which may cause discomfort. Our approach is based on the processing of long-term ballistocardiography data, which were measured using a sensory pad placed under the patient's mattress.
METHODS:The investigated dataset was obtained via long-term measurements in retirement homes and intensive care units (ICU). Data were measured unobtrusively using a measuring pad equipped with piezoceramic sensors. The proposed approach focused on the processing methods of the measured ballistocardiographic signals, Cartan curvature (CC), and Euclidean arc length (EAL).
RESULTS:For analysis, 218,979 normal and 216,259 aberrant 2-second samples were collected and classified using a convolutional neural network. Experiments using cross-validation with expert threshold and data length revealed the accuracy, sensitivity, and specificity of the proposed method to be 86.51 CONCLUSIONS: The proposed method provides a unique approach for an early detection of health concerns in an unobtrusive manner. In addition, the suitability of EAL over the CC was determined.
更多查看译文
关键词
health deterioration,geometric invariants,classification
AI 理解论文
溯源树
样例
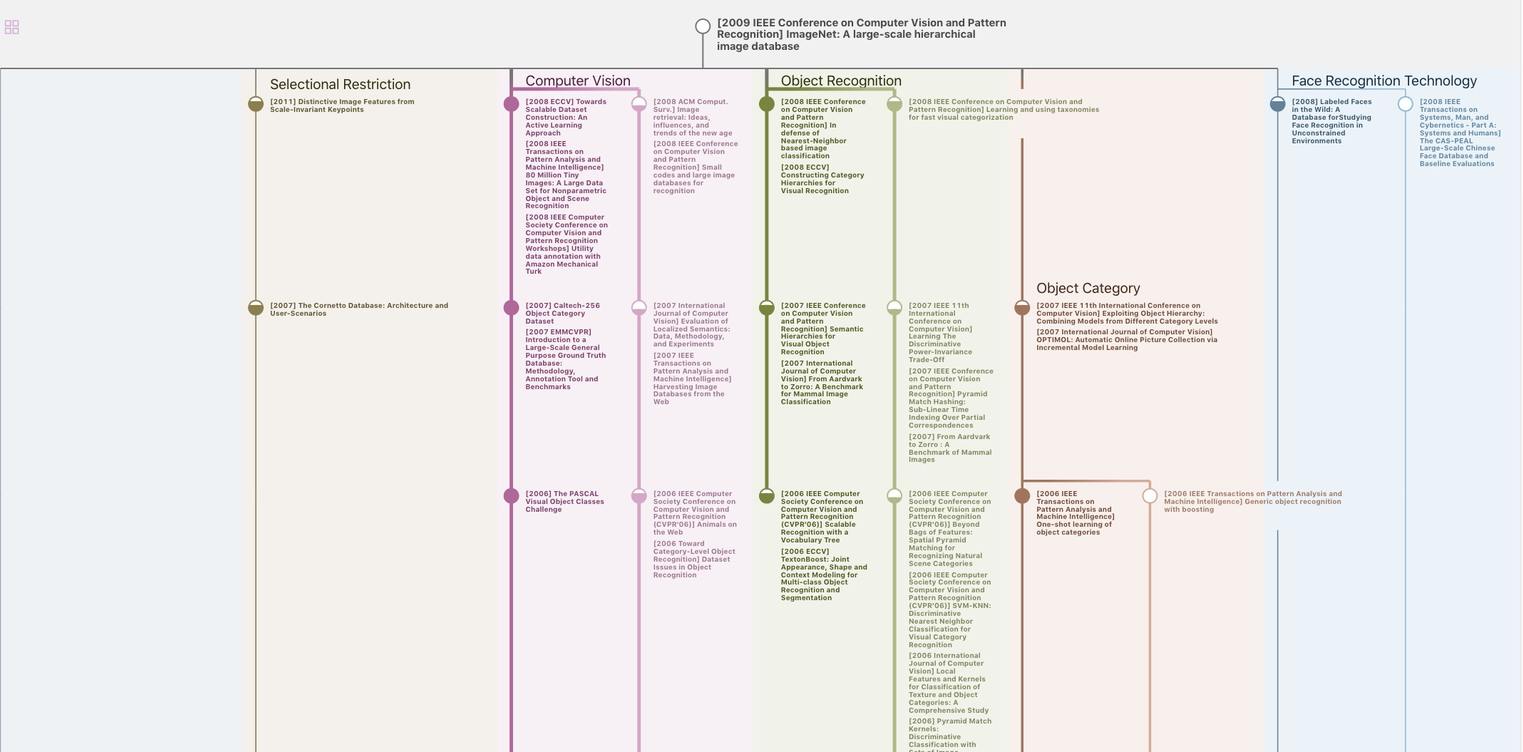
生成溯源树,研究论文发展脉络
Chat Paper
正在生成论文摘要