CPSS-FAT: A Consistent Positive Sample Selection for Object Detection with Full Adaptive Threshold
Pattern recognition(2023)
Abstract
Recent CNN-based methods for object detection largely focus on training the backbone of the object detector, neglecting the key part of selecting positive/negative samples. Instead, based on our analyses about the limitations and inconsistency of existing positive sample selection, we propose a novel Consis-tent Positive Sample Selection (CPSS) method to select the positive samples automatically, which joints Box-IoU and normalized central distance of object-anchor by mutual weighting. Meanwhile, we employ a consistent approach to location quality evaluation for suppressing the false-positive predicted box when inferencing. Furthermore, an auxiliary Full Adaptive Threshold (FAT) post-processing is also adopted ac-cording to the objects' occlusion level to improve the recall ratio. We implement the proposed CPSS-FAT detector using MS COCO 2017 and CityScapes datasets, the comparing results indicate that our approaches are effective and robust to the different objects in an open world. Especially, we achieve 52.2% AP and 43.1% ARS, outperforming most existing detectors.(c) 2023 Published by Elsevier Ltd.
MoreTranslated text
Key words
Object detection,Label assignment,Location quality estimation,Adaptive threshold
AI Read Science
Must-Reading Tree
Example
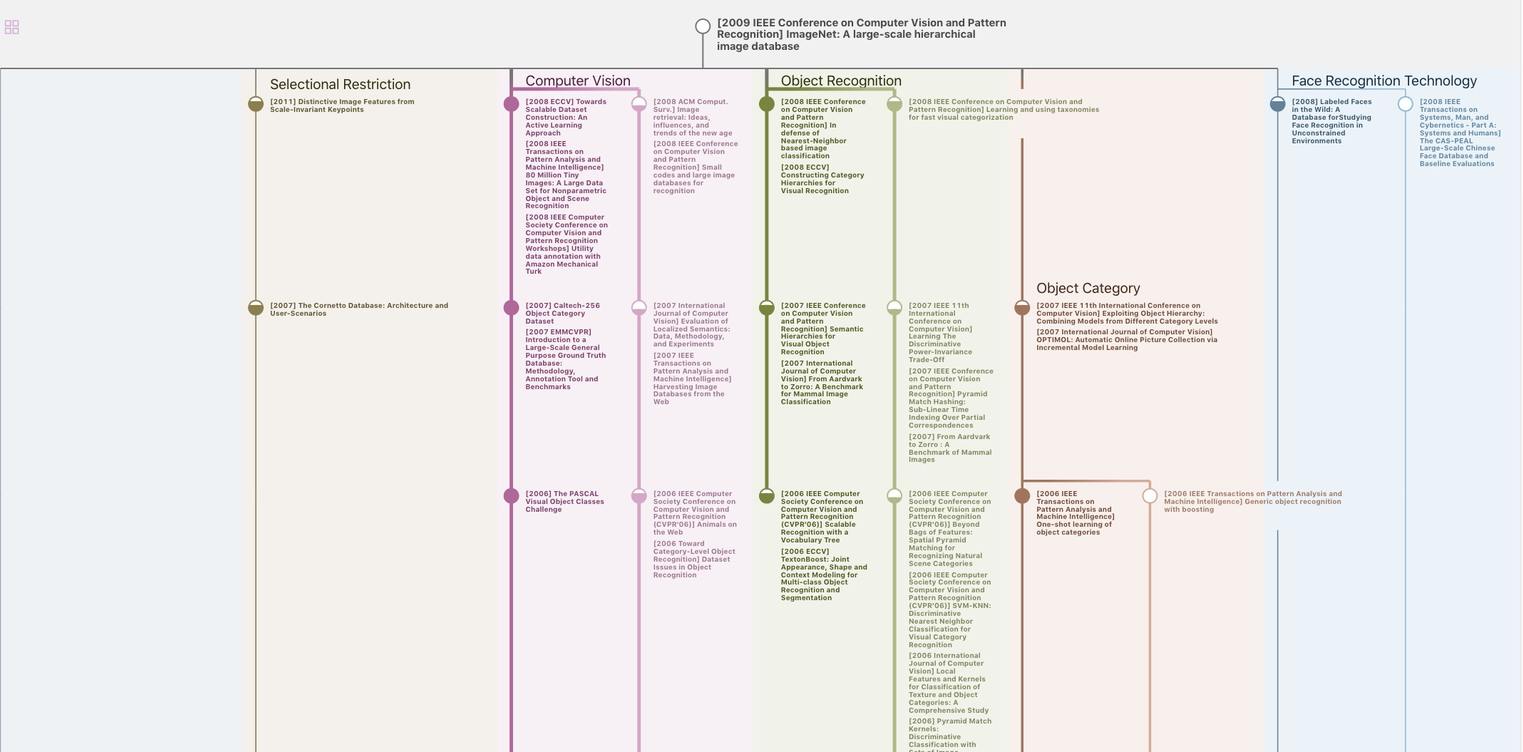
Generate MRT to find the research sequence of this paper
Chat Paper
Summary is being generated by the instructions you defined