Context-Aware and Click Session-Based Graph Pattern Mining With Recommendations for Smart EMS Through AI.
IEEE Access(2023)
摘要
In the field of Artificial Intelligence (AI), Smart Enterprise Management Systems (Smart EMS) and big data analytics are the most prominent computing technologies. A key component of the Smart EMS system is E-commerce, especially Session-based Recommender systems (SRS), which are typically utilized to enhance the user experience by providing recommendations analyzing user behavior encoded in browser sessions. Also the work of the recommender is to predict users' next actions (click on an item) using the sequence of actions in the current session. Current developments in session-based recommendation have primarily focused on mining more information accessible within the current session. On the other hand, those approaches ignored sessions with identical context for the current session that includes a wealth of collaborative data. Therefore this paper proposed Context-aware and Click session-based graph pattern mining with recommendations for Smart EMS through AI. It employs a novel Triple Attentive Neural Network (TANN) for SRS. Specifically, TANN contains three main components, i.e., Enhanced Sqrt-Cosine Similarity based Neighborhood Sessions Discovery (NSD), Frequent Subgraph Mining (FSM) using Neighborhood Click session-based graph pattern mining and Top-K possible Next-clicked Items Discovery (TNID). The NSD module uses a session-level attention mechanism to find m most similar sessions of the query session, and the FSM module also extracts the frequent subgraphs from the already discovered m most similar sessions of the query session via item-level attention. Then, TNID module is used to discover the top-K possible next-clicked items using the NSD and FSM module via a target-level attention. Finally, we perform comprehensive experiments on one big dataset, DIGINETICA, to verify the effectiveness of the TANN model, and the results of this experiment clearly illustrate the performance of TANN.
更多查看译文
关键词
graph pattern mining,smart ems,recommendations,context-aware,session-based
AI 理解论文
溯源树
样例
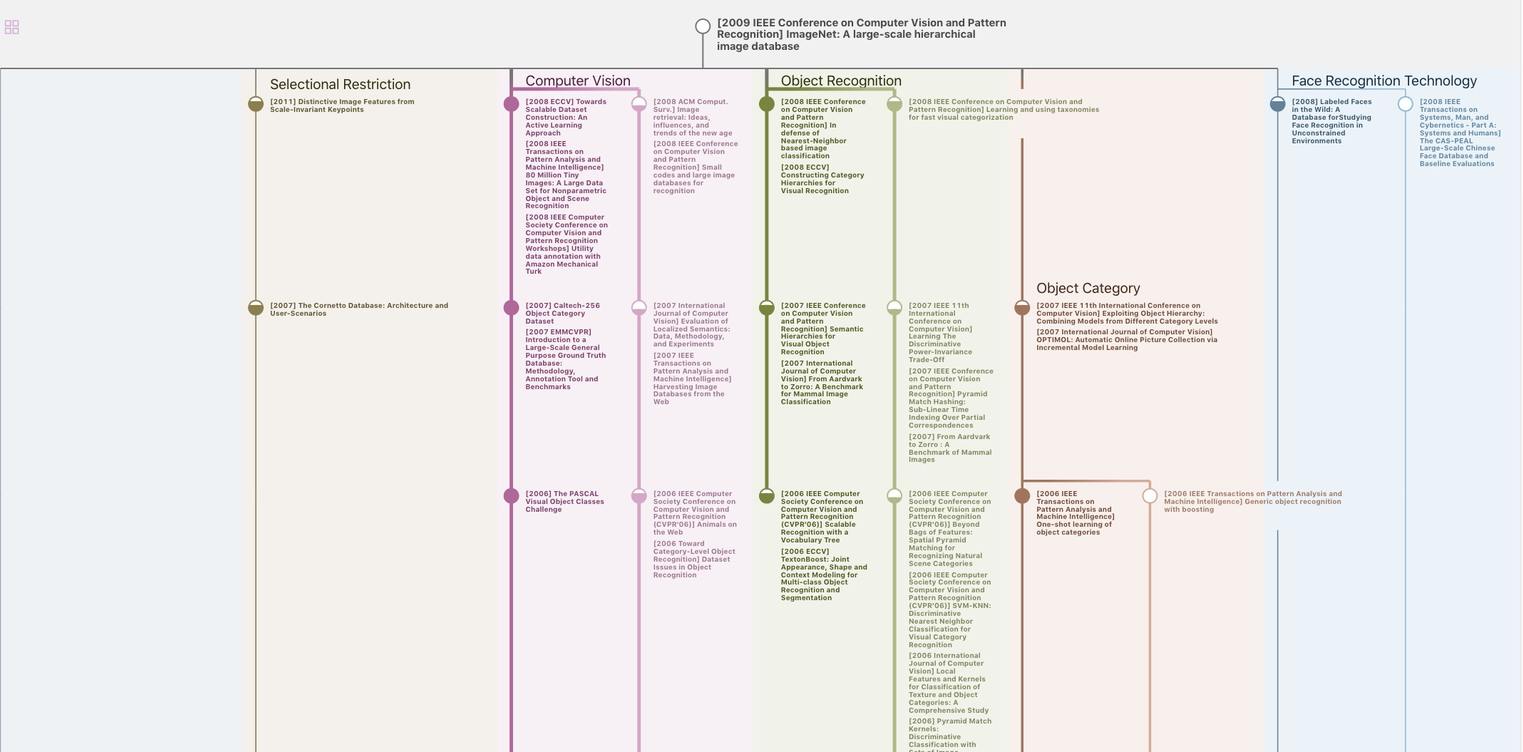
生成溯源树,研究论文发展脉络
Chat Paper
正在生成论文摘要