TAGU-Net: Transformer Convolution Hybrid-Based U-Net With Attention Gate for Atypical Meningioma Segmentation.
IEEE Access(2023)
摘要
Meningioma is derived from the cap cells that reside on the arachnoid membrane. The atypical meninges of Grade II, a classification established by the World Health Organization, are included in one of the grades of meningioma. It has been discovered that early surgical resection significantly reduces the recurrence rate and mortality of tumors. Accurate segmentation of magnetic resonance images of brain tumors is crucial for diagnosing and treating atypical meningiomas. However, the traditional automatic segmentation framework heavily relies on convolution. The convolution-based segmentation network has limitations such as the size of the convolution kernels, a restricted receptive field, and a lack of spatial aggregation ability. To overcome these limitations, this paper presents a novel hybrid architecture named TAGU-Net, which combines Transformer and convolution based on U-Net with an attention gate. The TAGU-Net architecture extracts features of different resolution feature scales using convolutional neural network and Transformer. This approach effectively captures the image's long-distance dependency and global characteristics in the encoder stage, relying on the global self-attention mechanism of the Transformer. Additionally, the inductive bias of the convolution neural network is combined to enhance the local modeling information and improve the model's overall modeling ability. In the decoder phase, the attention gate is introduced to adaptively learn the skip connection information and up-sampling information in the network. This information is weighted and fused to highlight important features and suppress irrelevant features. To obtain better model training and avoid the vanishing gradient, deep supervision technology is used in the training process. Supplementary loss is added in some stages to supervise the training and achieve the best effect of atypical meningioma segmentation. The proposed method is evaluated on both the private atypical meningioma dataset and the publicly available BraTs2018 dataset.TAGU-Net has achieved Dice Scores of 97.67% and 97.62% and Jaccard index of 96.35% and 95.35% on the private atypical meningioma dataset and BraTs2018 dataset respectively, which is a state-of-the-art segmentation result beyond existing methods. According to the research results, the TAGU-Net model significantly improves atypical meningioma segmentation and can effectively assist doctors in processing MRI images.
更多查看译文
关键词
attention gate,segmentation,tagu-net,hybrid-based
AI 理解论文
溯源树
样例
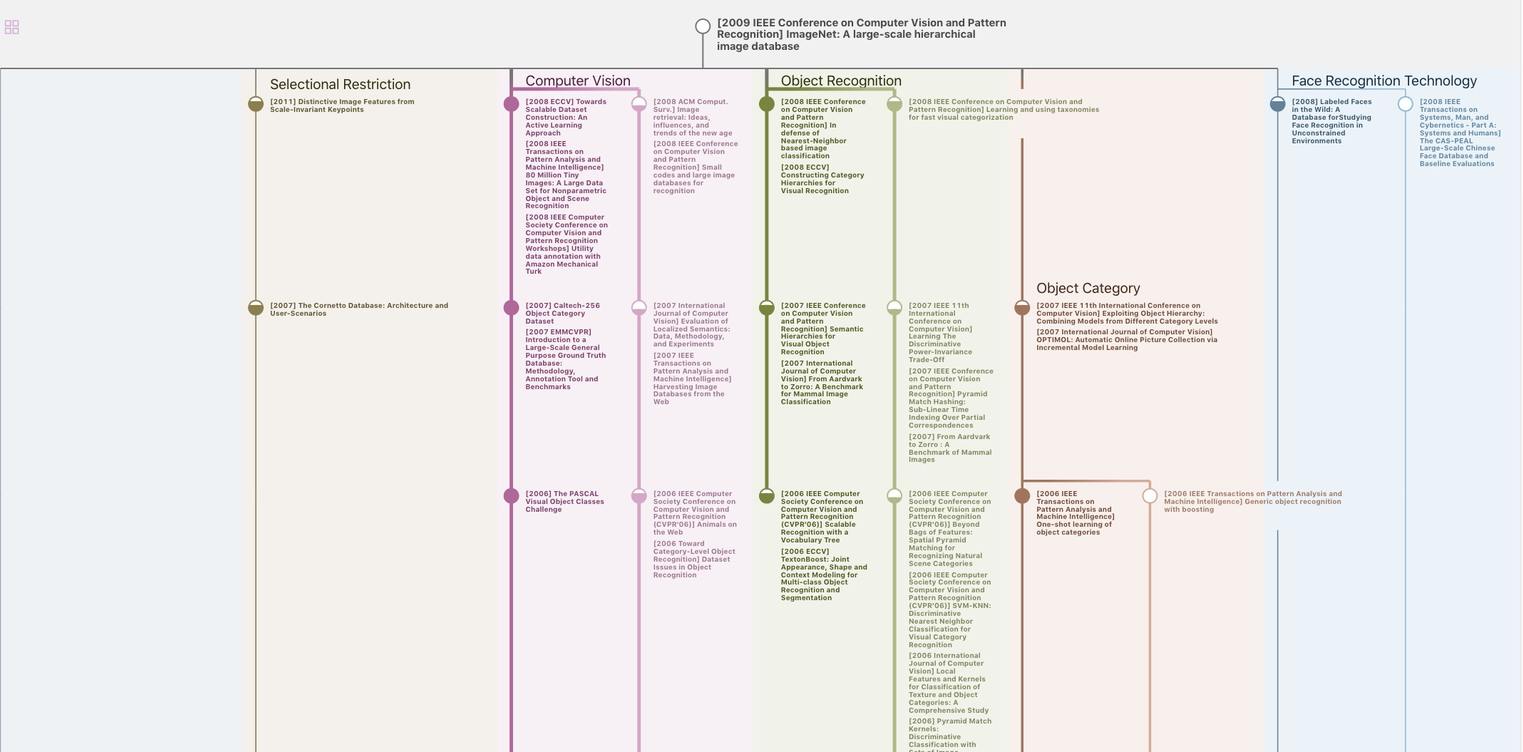
生成溯源树,研究论文发展脉络
Chat Paper
正在生成论文摘要