An Automatic Identification Framework for Complex Power Quality Disturbances Based on Ensemble CNN
IEEE access(2023)
摘要
A large number of electric vehicles (EVs) are connected to the grid, increasing the risk of power quality deterioration. Meanwhile, power quality disturbances (PQDs) directly affect EV charging safety. Intelligent identification of complex PQDs is the basis for solving the power quality problem, which is very meaningful for improving EV charging quality. This paper proposes an automatic recognition framework for complex PQDs based on ensemble convolution neural network (ECNN). Firstly, a multifusion structure on account of the time and frequency domain feature of PQDs signals is introduced. In addition, a composite convolution is proposed to reduce network complexity, which is using the standard convolution and depthwise separable convolution. Then, we design an adaptive-context mechanism to extend the versatility of ECNN. At the same time, the need to use batch normalization to accelerate training convergence and prevent training overfitting is verified. Furthermore, some visualization methods are performed to analyze the inner mode and illustrate the working mechanism of ECNN. Finally, we apply various experiments to prove the effectiveness of ECNN. Compared to other advanced deep neural networks and traditional methods, ECNN has better noise resistance, higher accuracy, and lower training cost.
更多查看译文
关键词
Power quality disturbances (PQDs),ensemble convolutional neural network (ECNN),composite convolution,identification,visualization
AI 理解论文
溯源树
样例
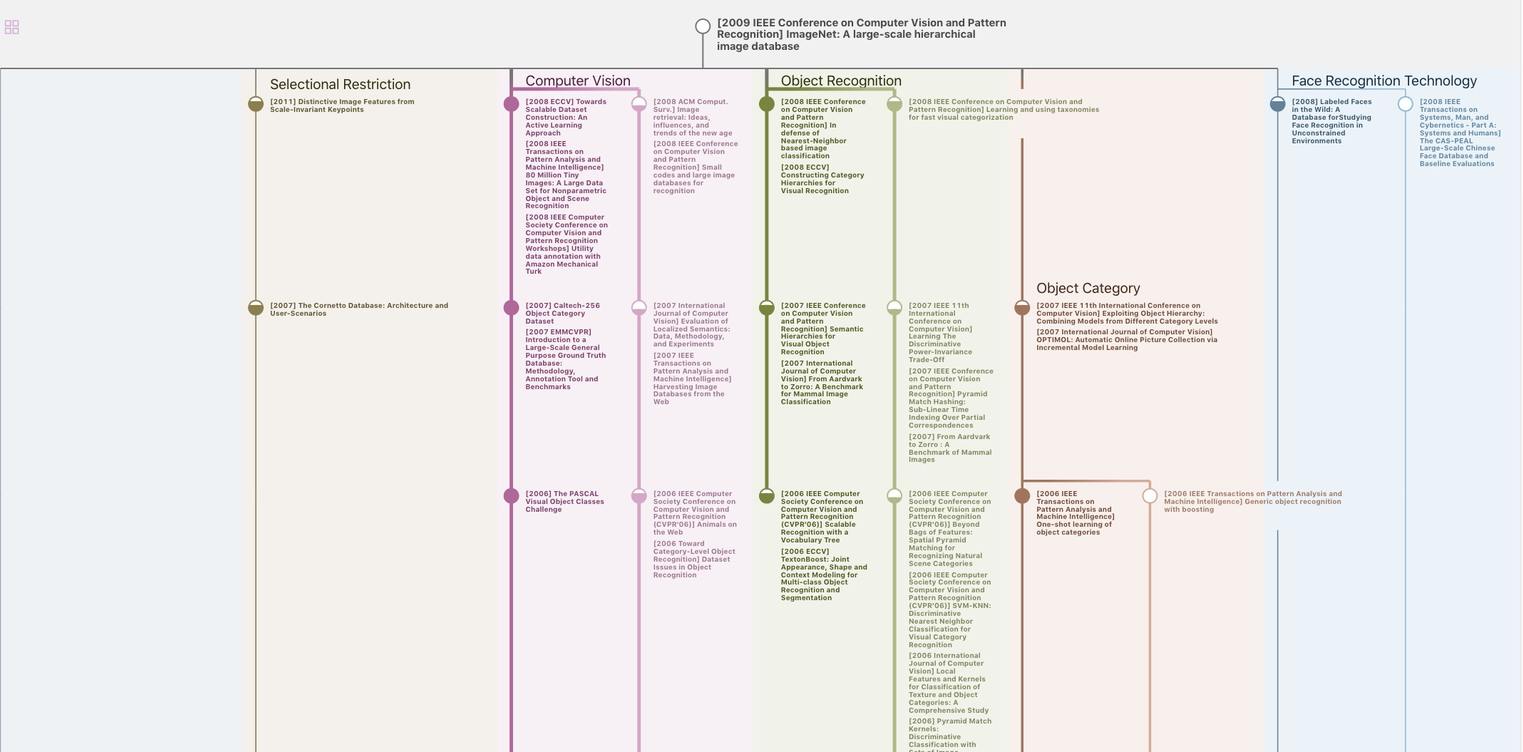
生成溯源树,研究论文发展脉络
Chat Paper
正在生成论文摘要