Artificial Rabbits Optimizer With Machine Learning Based Emergency Department Monitoring and Medical Data Classification at KSA Hospitals.
IEEE Access(2023)
摘要
The Emergency Departments (EDs) in health centres located in the main areas of Saudi Arabia have heavy patient inflow because of the pandemic, viral infections, and even on some special occasions like Umrah or Hajj, where pilgrims who travel from one place to another with serious disorders. Other than the EDs, it was important to observe the patient's activities from ED to other wards in the region or the hospital to track the spread of viral diseases. In this case, deep learning (DL) and machine learning (ML) methods have been used to track the target audience and classify the data into many classes. With this motivation, this study develops an artificial rabbit optimization with a machine learning-based healthcare data classification (AROML-HDC) technique for EDs. The AROML-HDC technique monitors and tracks the patient visit data, treatment given, and length of stay (LOS). In addition, the AROML-HDC technique designs an effective ARO algorithm for the optimal selection of feature subsets. Next, the class-specific cost regulation extreme learning machine (CSCR-ELM) classifier is applied for effective medical data classification. Finally, the grasshopper optimization algorithm (GOA) was used to adjust the parameters related to the CSCR-ELM classifier. The experimental outcome of the AROML-HDC approach is tested on the benchmark Cleveland dataset and the Statlog dataset comprising 297 and 270 samples, respectively. The simulation results signify the improved performance of the AROML-HDC technique over other recent methods with maximum accuracy of 93.22% and 94.05% Cleveland dataset and the Statlog dataset, respectively.
更多查看译文
关键词
medical data classification,emergency department,machine learning
AI 理解论文
溯源树
样例
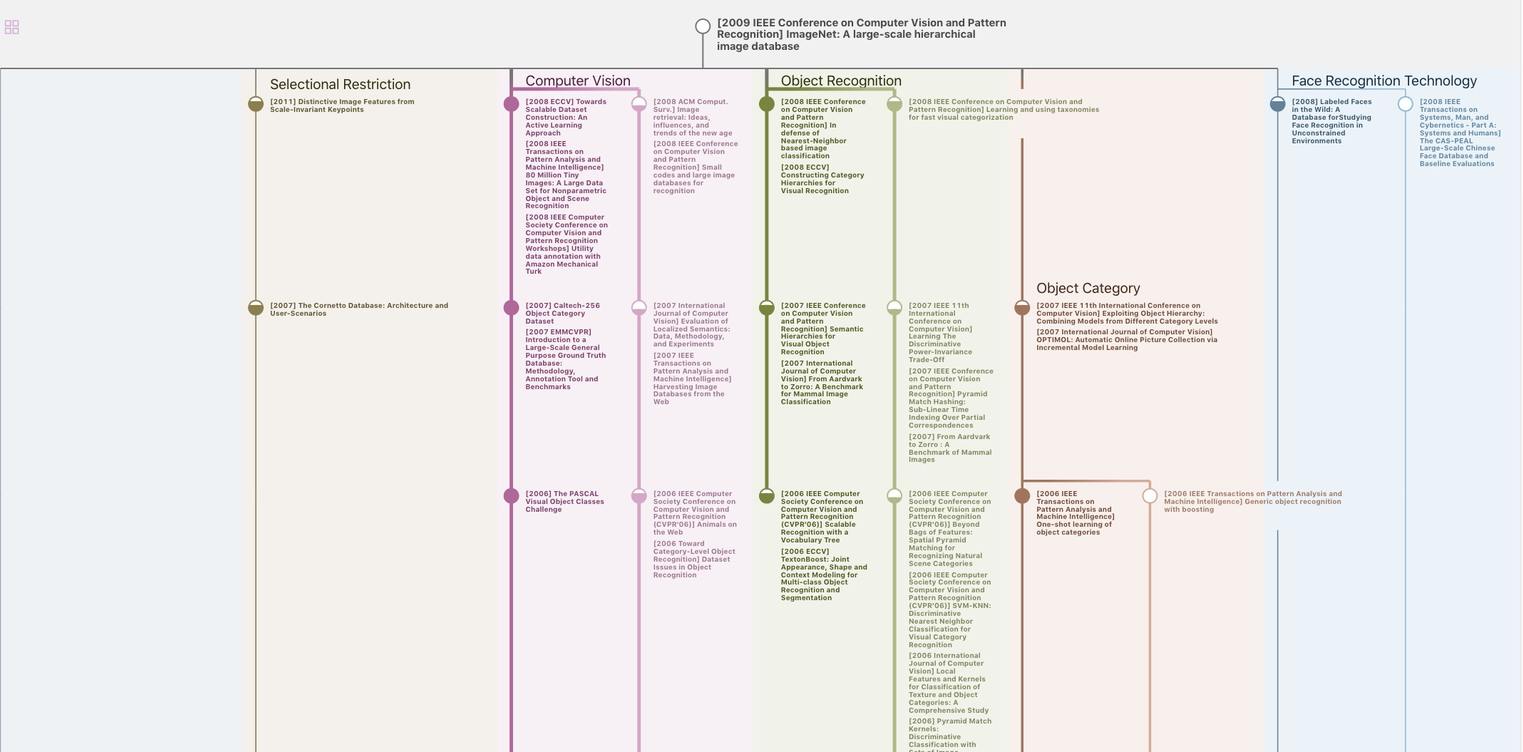
生成溯源树,研究论文发展脉络
Chat Paper
正在生成论文摘要