Learning resource recommendation via knowledge graphs and learning style clustering.
J. Intell. Fuzzy Syst.(2023)
摘要
An e-learning system should recommend learners appropriate learning resources according to their actual needs and cognitive status for improving their learning performance. To overcome the deficiencies of existing approaches (e.g., poor interpretability, limited efficiency and accuracy of recommendation), we propose a new recommendation approach to learning resources via knowledge graphs and learning style clustering. In this approach, the knowledge graphs of an online learning environment are constructed based on a generic ontology model, and the graph embedding algorithm and graph matching process are applied to optimize the efficiency of graph computation for identifying similar learning resources. By introducing learning style theory, learners are clustered based on their learning styles. Based on the clustering results, the learners’ degrees of interest in similar learning resources are measured, and the recommendation results are obtained according to the degrees of interest. Finally, the experiments demonstrate that the proposed approach significantly enhances the computational efficiency and the quality of learning resource recommendation compared with the existing approaches in large-scale graph data scenarios.
更多查看译文
关键词
resource recommendation,knowledge graphs
AI 理解论文
溯源树
样例
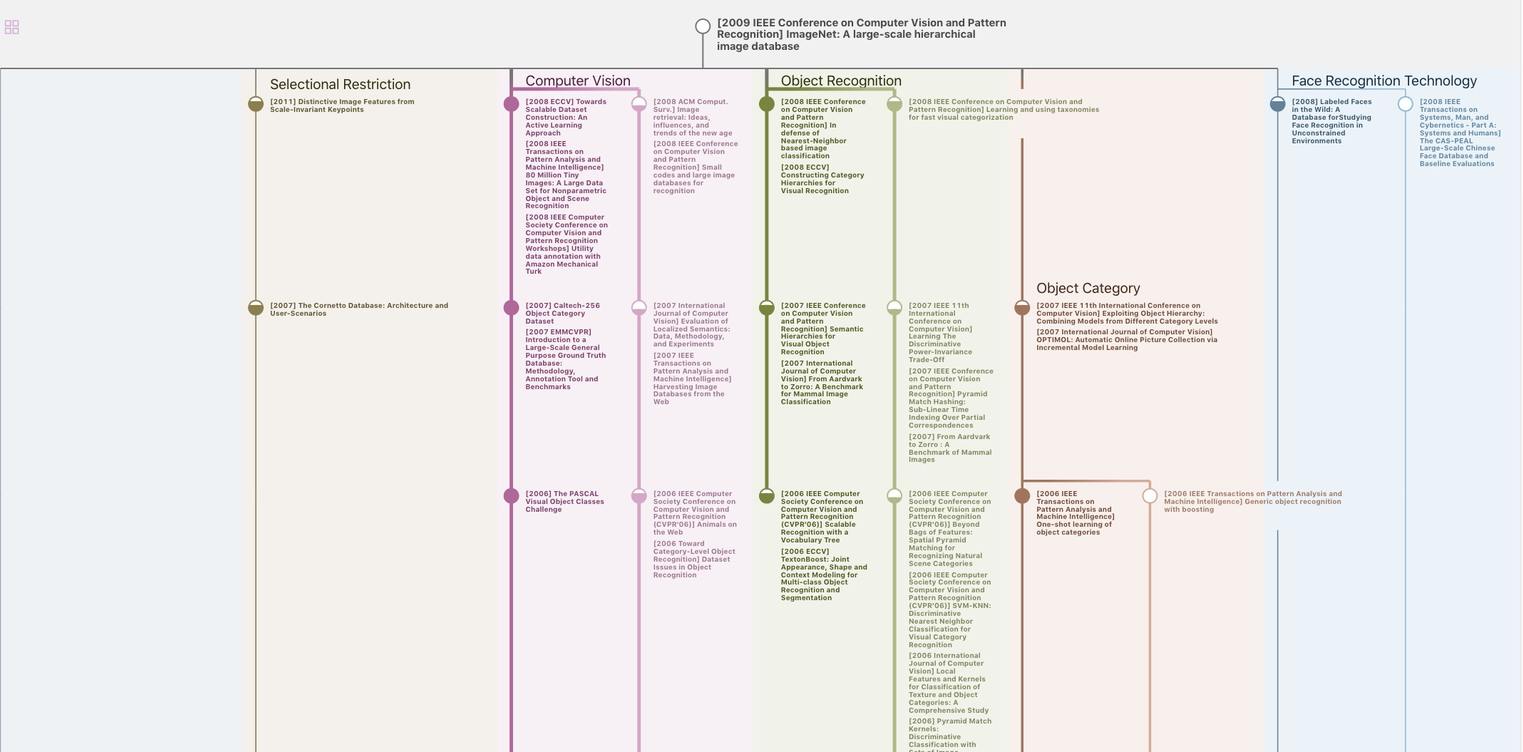
生成溯源树,研究论文发展脉络
Chat Paper
正在生成论文摘要