Low cost network traffic measurement and fast recovery via redundant row subspace-based matrix completion
Connect. Sci.(2023)
摘要
Traffic matrices (TMs) are essential for managing networks. Getting the whole TMs is difficult because of the high measurement cost. Several recent studies propose sparse measurement schemes to reduce the cost, which involve taking measurements on only a subset of origin and destination pairs (OD pairs) and inferring data for unmeasured OD pairs through matrix completion. However, existing sparse network measurement schemes suffer from the problems of high computation costs and low recovery quality. This paper investigates the coherence feature of real traffic flow data traces (Abilene and GEANT). Both data sets are high coherence, with column coherence greater than row coherence. According to the coherence feature of both data sets, we propose our Redundant Row Subspace-based Matrix Completion (RRS-MC). RRS-MC involves several techniques. Firstly, we design an algorithm to identify subspace rows (OD pairs) from historical data. Secondly, based on the identified subspace rows, we design our sampling scheduling algorithm, which takes full measurement samples in subspace rows while taking partial measurement samples in the remaining rows. Moreover, we propose a redundant sampling rule prevent the recovery accuracy decrease caused by the subspace rows varying. Finally, we design a completion algorithm to recover the partially measured rows. We conduct extensive experiments. Results indicate that the proposed scheme is superior to the state-of-the-art sampling and completion scheme in computation costs and recovery accuracy.
更多查看译文
关键词
Sparse measurement technique,high column coherence,subspace-based matrix completion
AI 理解论文
溯源树
样例
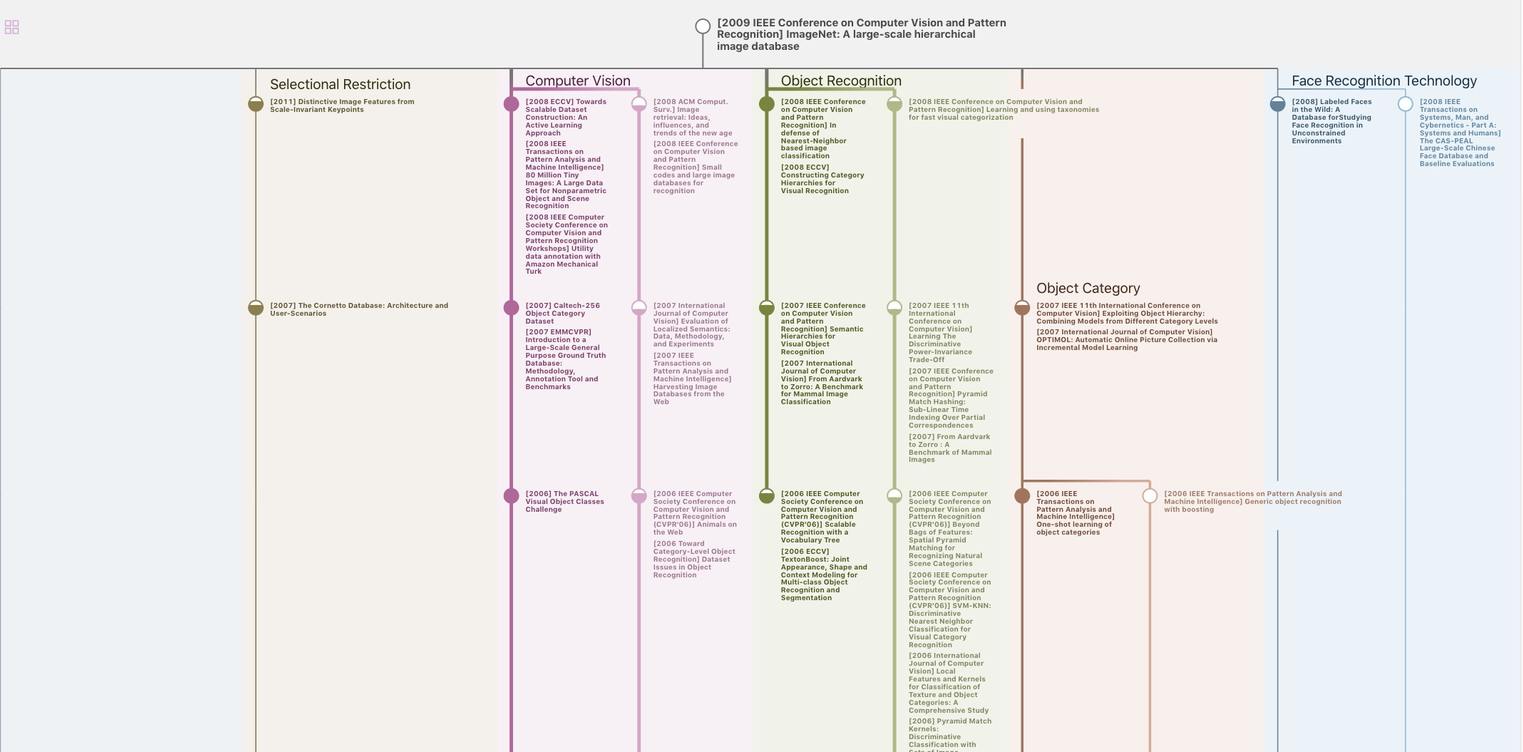
生成溯源树,研究论文发展脉络
Chat Paper
正在生成论文摘要