Facial Expression and Electrodermal Activity Analysis for Continuous Pain Intensity Monitoring On The X-ITE Pain Database
crossref(2021)
摘要
Abstract Automatic systems enable continuous monitoring of patients' pain intensity as shown in prior studies. Facial expression and physiological data such as electrodermal activity (EDA) are very informative for pain recognition. The features extracted from EDA indicate the stress and anxiety caused by different levels of pain. In this paper, we investigate using the EDA modality and fusing two modalities (frontal RGB video and EDA) for continuous pain intensity recognition with the X-ITE Pain Database. Further, we compare the performance of automated models before and after reducing the imbalance problem in heat and electrical pain datasets that include phasic (short) and tonic (long) stimuli. We use three distinct real-time methods: A Random Forest (RF) baseline methods [Random Forest classifier (RFc) and Random Forest regression (RFr)], Long-Short Term Memory Network (LSTM), and LSTM using sample weighting method (called LSTM-SW). Experimental results (1) report the first results of continuous pain intensity recognition using EDA data on the X-ITE Pain Database, (2) show that LSTM and LSTM-SW outperform guessing and baseline methods (RFc and RFr), (3) confirm that the electrodermal activity (EDA) with most models is the best, (4) show the fusion of the output of two LSTM models using facial expression and EDA data (called Decision Fusion = DF). The DF improves results further with some datasets (e.g. Heat Phasic Dataset (HTD)).
更多查看译文
AI 理解论文
溯源树
样例
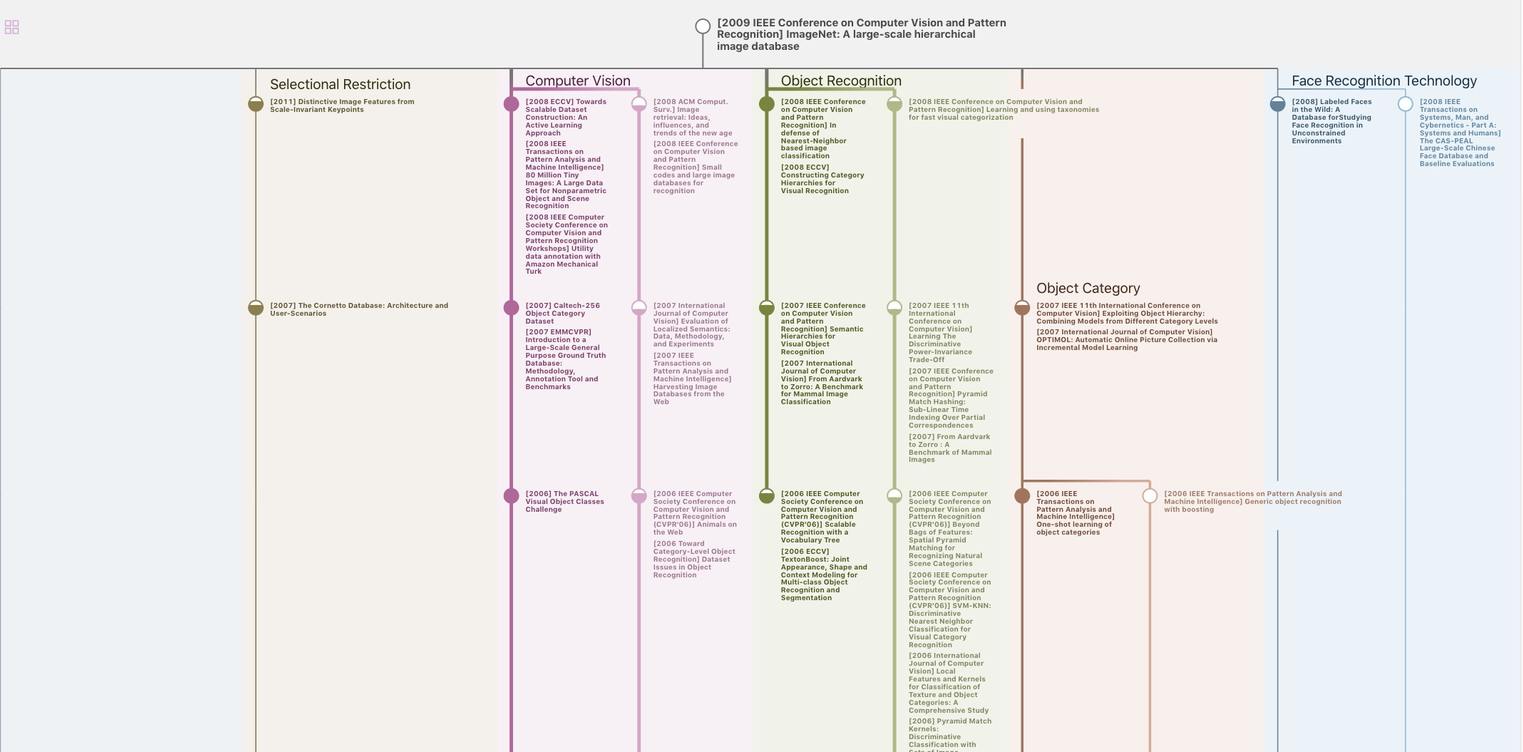
生成溯源树,研究论文发展脉络
Chat Paper
正在生成论文摘要