Assessing vehicle fuel efficiency using a dense network of CO<sub>2</sub> observations
crossref(2021)
摘要
Abstract. Transportation represents the largest sector of anthropogenic CO2 emissions in urban areas. Timely reductions in urban transportation emissions are critical to reaching climate goals set by international treaties, national policies, and local governments. Transportation emissions also remain one of the largest contributors to both poor air quality (AQ) and to inequities in AQ exposure. As municipal and regional governments create policy targeted at reducing transportation emissions, the ability to evaluate the efficacy of such emission reduction strategies at the spatial and temporal scales of neighborhoods is increasingly important. However, the current state of the art in emissions monitoring does not provide the temporal, sectoral, or spatial resolution necessary to track changes in emissions and provide feedback on the efficacy of such policies at a neighborhood scale. The BErkeley Air Quality and CO2 Network (BEACO2N) has previously been shown to provide constraints on emissions from the vehicle sector in aggregate over a ~1300 km2 multi-city spatial domain. Here, we focus on a 5 km, high volume, stretch of highway in the SF Bay area. We show that inversion of the BEACO2N measurements can be used to understand two factors that affect fuel efficiency: vehicle speed and fleet composition. The CO2 emission rate of the average vehicle (g/vkm) are shown to vary by as much as 27 % at different times of a typical weekday because of changes in vehicle speed and fleet composition. The BEACO2N-derived emissions estimates are consistent to within ~3 % of estimates derived from publicly available measures of vehicle type, number, and speed, providing direct observational support for the accuracy of the Emissions FACtor model (EMFAC) of vehicle fuel efficiency.
更多查看译文
AI 理解论文
溯源树
样例
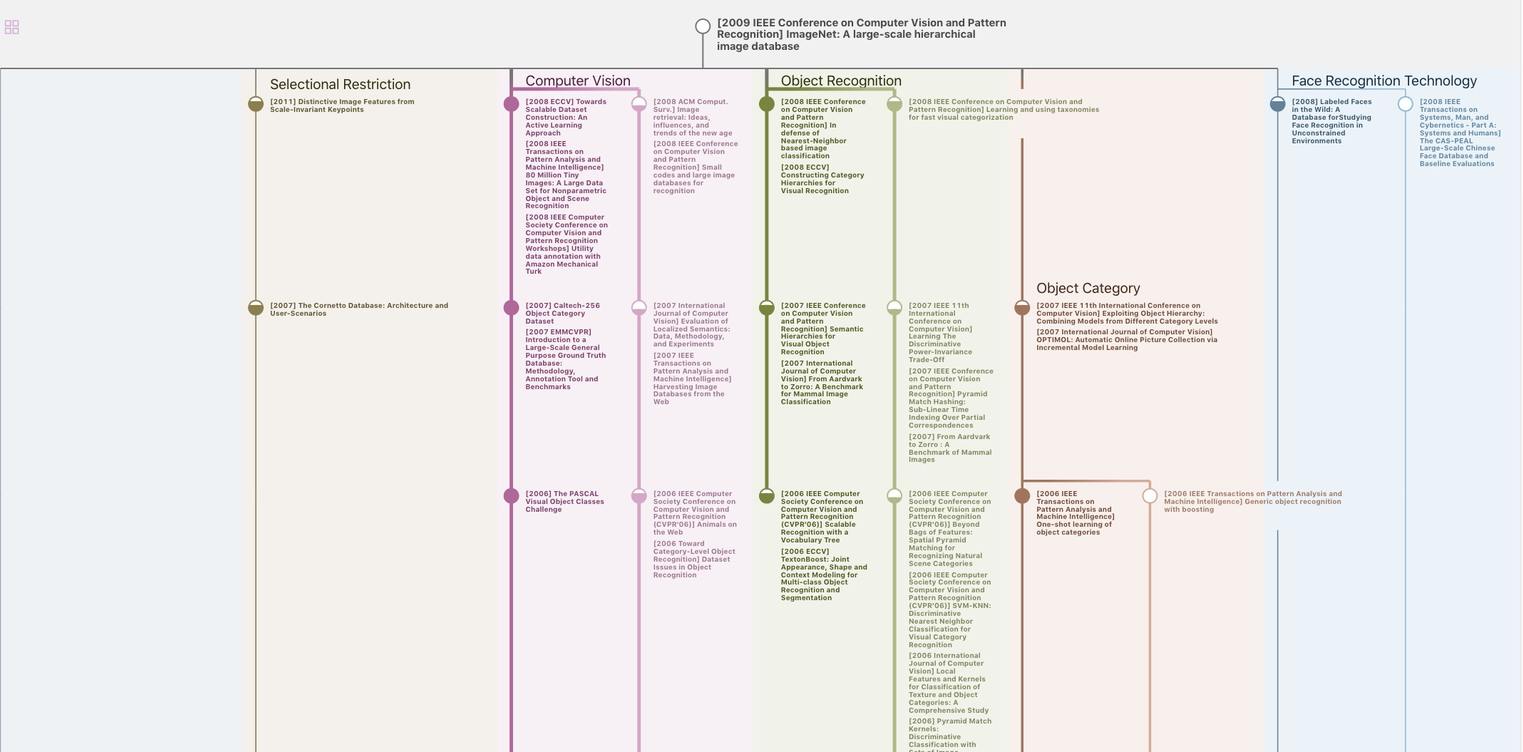
生成溯源树,研究论文发展脉络
Chat Paper
正在生成论文摘要