A Multimodal Deep Learning-based Platform that Assists in the Classification of Lupus Subtypes and Differential Diagnosis Among Lupus and Other Similar Skin Diseases
Research Square (Research Square)(2021)
摘要
Abstract Lupus erythematosus (LE) has the reputation of being the Great Imitator due to its various cutaneous manifestations, which often lead to difficulties in accurate diagnosis and classification. Even with the assistance of deep learning systems (DLSs), clinical skin image-based single modalities still experience difficulty distinguishing subtypes of LE. Here, we present an application of multimodal DLS (MMDLS), in which the training database consists of clinical skin images, multi-immunohistochemistry (multi-IHC) images and the 2019 European League Against Rheumatism/American College of Rheumatology (EULAR/ACR) scores, to assist in the classification of LE subtypes (discoid LE (DLE), annular subacute cutaneous LE (A-SCLE), papulosquamous subacute cutaneous LE (P-SCLE), systemic LE (SLE)) and to aid in the differential diagnosis of other similar skin diseases. A total of 386 cases with 580 clinical skin images, 3380 multi-IHC images and 2019 EULAR/ACR scores from 25 institutions in China were included, and EfficientNet-B3 and ResNet-18 were utilized in this study. By comparing the single-modal and dual-modal DLS performances in multiple classifications, we demonstrate the superiority of the MMDLS (Ave-Sen = 0.9116, Ave-Spe = 0.9921, Ave-Pre = 0.9281). Moreover, the performance of MMDLS on 13 classifications was superior to that of dermatologists and pathologists (F1 score: dermatologists = 0.4471, pathologists = 0.6691, MMDLS = 0.9582) with an average area under the curve (AUC) of 0.9956. These results highlight the potential of the combined application of MMDLS and multi-IHC images to assist pathologists and dermatologists in diagnosing LE subtypes and similar skin diseases.
更多查看译文
关键词
lupus subtypes,differential diagnosis,learning-based
AI 理解论文
溯源树
样例
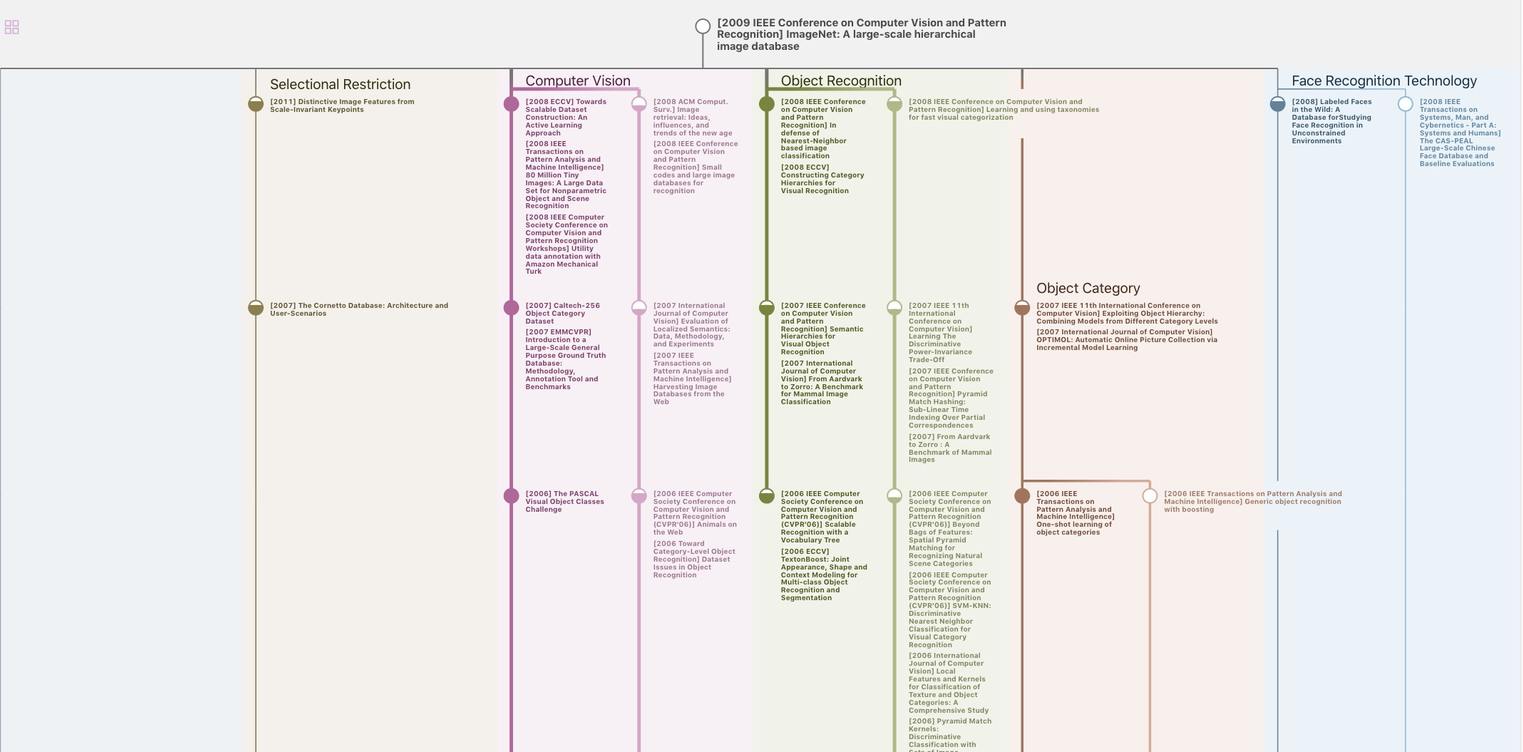
生成溯源树,研究论文发展脉络
Chat Paper
正在生成论文摘要