Thalamic hyperconnectivity as neurophysiological signature of major depressive disorder in two multicenter studies
crossref(2021)
摘要
The promise of machine learning has fueled the hope for developing diagnostic tools for psychiatry. Initial studies showed high accuracy for the identification of major depressive disorder (MDD) with resting-state connectivity, but progress has been hampered by the absence of large datasets. Here we used regular machine learning and advanced deep learning algorithms to differentiate patients with MDD from healthy controls and identify neurophysiological signatures of depression in two of the largest resting-state datasets for MDD. Resting-state functional magnetic resonance imaging data were obtained from the REST-meta-MDD (N=2338) and PsyMRI (N=1039) consortia. Classification of functional connectivity matrices was done using support vector machines (SVM) and graph convolutional neural networks (GCN) and performance was evaluated using 5-fold cross-validation. Results were visualized using GCN-Explainer, an ablation study and univariate t-testing.Mean classification accuracy was 61% for MDD versus controls. Mean accuracy for classifying (non-)medicated subgroups was 62%. Visualization of the results showed that classifications were driven by stronger thalamic connections in both datasets, while nearly all other connections were weaker with small univariate effect sizes.Whole brain resting-state connectivity is a reliable though poor biomarker for MDD, presumably due to disease heterogeneity. Deep learning revealed thalamic hyperconnectivity as a prominent neurophysiological signature of depression in both multicenter studies, which may guide the development of biomarkers in future studies.
更多查看译文
AI 理解论文
溯源树
样例
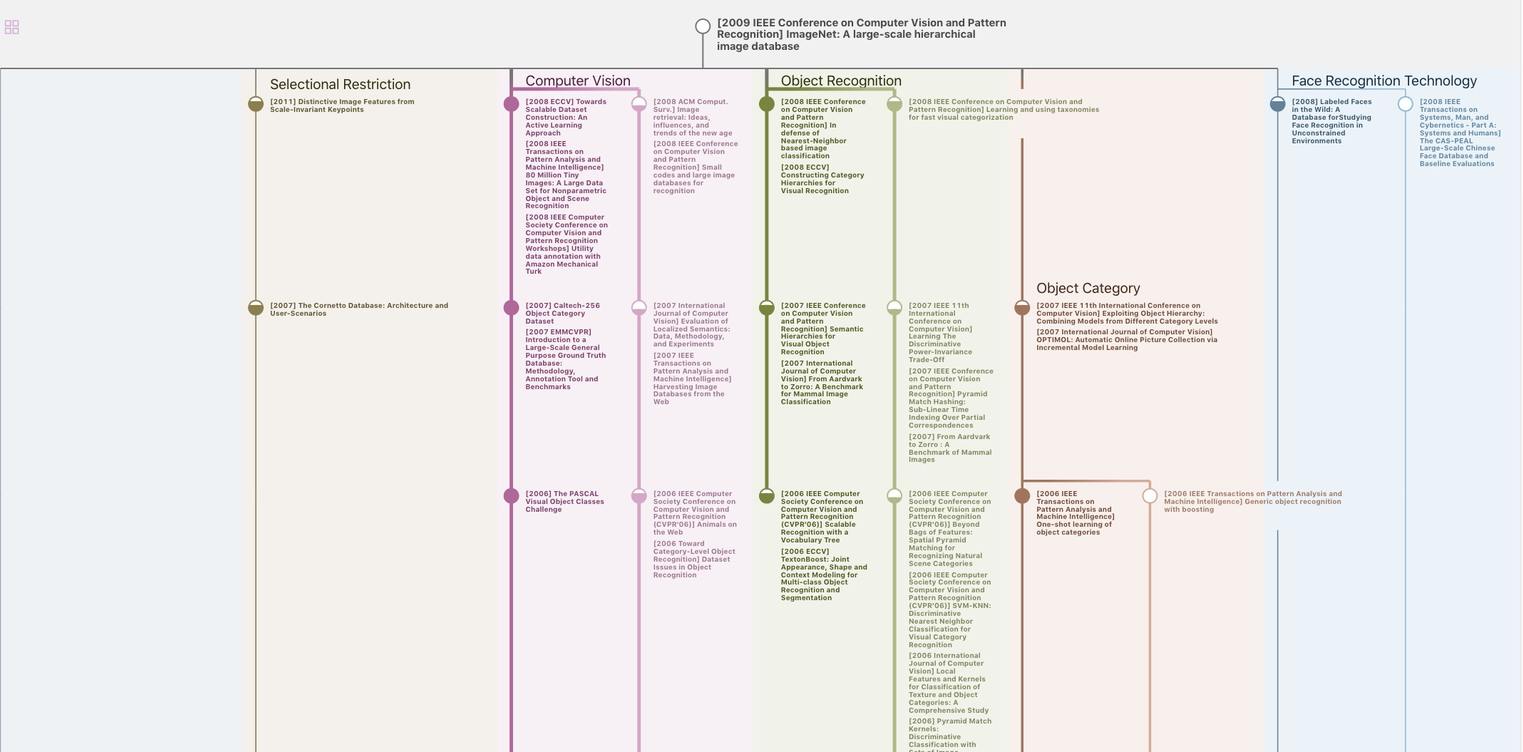
生成溯源树,研究论文发展脉络
Chat Paper
正在生成论文摘要