Identify Prognostic Factors and Establish a Risk Stratification Model to Predict Cancer-Specific Survival for Patients with Pancreatic Cancer Liver Metastasis: A Population-Based Study
crossref(2021)
摘要
Abstract Background Pancreatic cancer liver metastasis (PCLM) is a commonly fatal disease, but there are few prognostic models for these entities. The purpose of this study is to investigate prognostic factors based on clinicopathological characteristics and establish a prognostic nomogram predicting the cancer-specific survival (CSS) for PCLM patients. Methods The characteristics of 6015 patients with PCLM between 2010 and 2015 from Surveillance, Epidemiology, and End Results (SEER) database were analyzed. Prognostic factors and nomogram predicting CSS were developed by Cox proportional hazard regression model. The predictive accuracy and discriminative ability of nomogram were assessed by concordance index (C-index), calibration curve, decision curve analyses (DCAs) and receiver operating characteristic (ROC) curve. Moreover, a risk classification system was built according to the cut-off values off the nomogram. Results Based on the univariate and multivariate Cox regression analysis, significant prognostic factors were identified and included to establish the nomogram for CSS. The median survival time (MST) for all patients is 4.0 months (95% confidence interval [CI]:3.8–4.2) and CSS at 6, 12 and 18 months was 34.12%, 15.63% and 7.83%, respectively. The C-index of nomogram was 0.693 (95%CI: 0.689–0.697) and all verification results showed an accurate and discriminative ability in predicting CSS. Significant differences in Kaplan–Meier curves were observed in patients stratified into different risk groups (p < 0.001), with MST of 7.0 months (95% CI: 6.7–7.3), 3.0 months (95% CI: 2.7–3.3), and 2.0 months (95% CI: 1.8–2.2), respectively. Conclusions A prognostic nomogram and corresponding risk classification system were proposed to predict CSS for PCLM.
更多查看译文
AI 理解论文
溯源树
样例
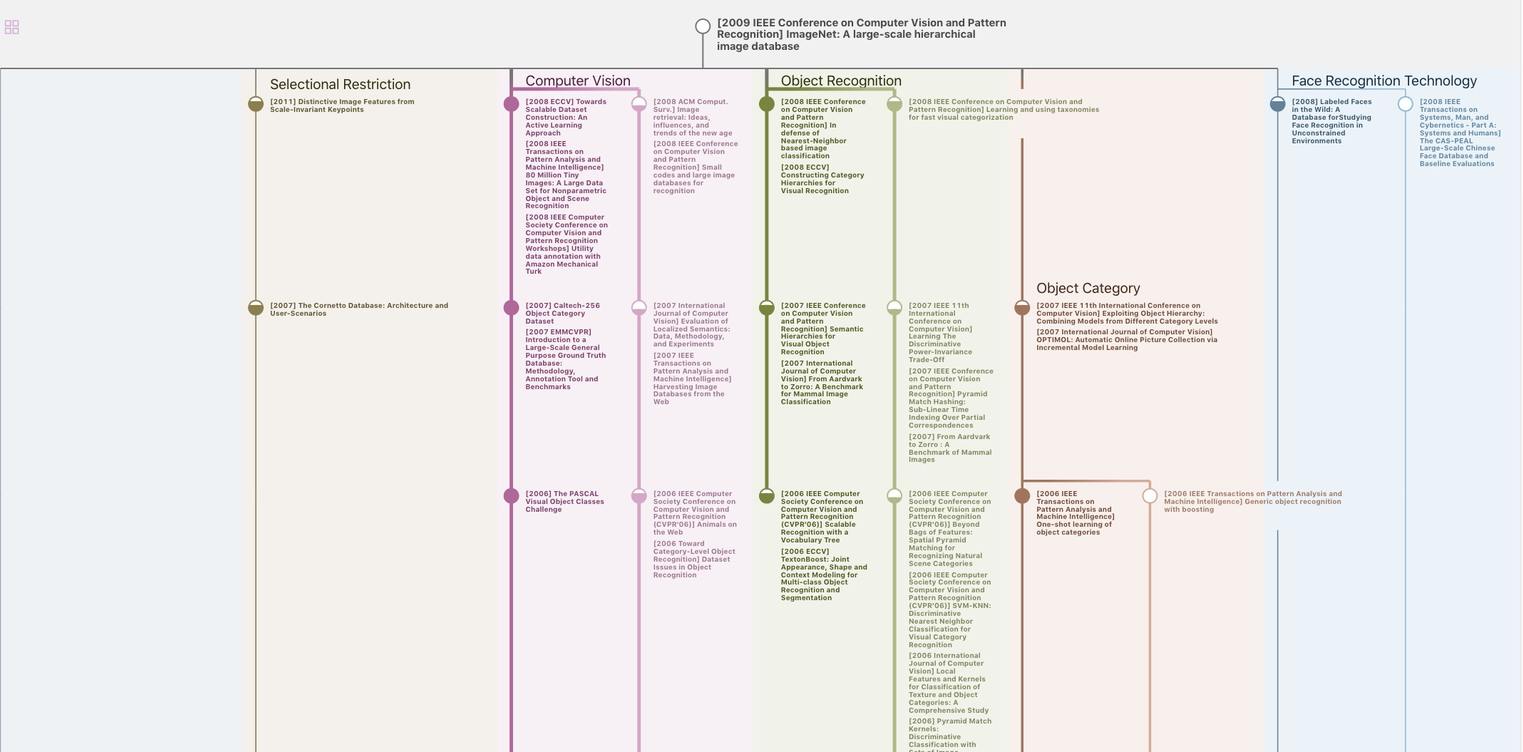
生成溯源树,研究论文发展脉络
Chat Paper
正在生成论文摘要