SubMo-GNN: Property- and Structure-Aware Diverse Molecular Selection with Representation Learning and Mathematical Diverse-Selection Framework
Research Square (Research Square)(2021)
摘要
Abstract Selecting diverse molecules from unexplored areas of chemical space is one of the most important tasks for discovering novel molecules and reactions. This paper develops a new method for selecting a diverse subset of molecules from a given molecular list by utilizing two techniques studied in machine learning and mathematical optimization: graph neural networks (GNNs) for learning vector representation of molecules and a diverse-selection framework called submodular function maximization. Our method first trains a GNN with property prediction tasks, and then the trained GNN transforms molecular graphs into molecular vectors, which capture both properties and structures of molecules. Finally, to obtain a diverse subset of molecules, we define a submodular function, which quantifies the diversity of molecular vectors, and find a subset of molecular vectors with a large submodular function value. This can be done efficiently by using the greedy algorithm, and the diversity of selected molecules measured by the submodular function value is mathematically guaranteed to be at least 63 % of that of an optimal selection. We also introduce a new evaluation criterion to measure the diversity of selected molecules based on molecular properties. Computational experiments confirm that our method successfully selects diverse molecules from the QM9 dataset regarding the property-based criterion, while performing comparably to existing methods regarding a standard structure-based criterion. The proposed method enables researchers to obtain diverse sets of molecules for discovering new molecules and novel chemical reactions, and the proposed diversity criterion is useful for discussing the diversity of molecular libraries from a new property-based perspective.
更多查看译文
关键词
molecular,representation learning,submo-gnn,structure-aware,diverse-selection
AI 理解论文
溯源树
样例
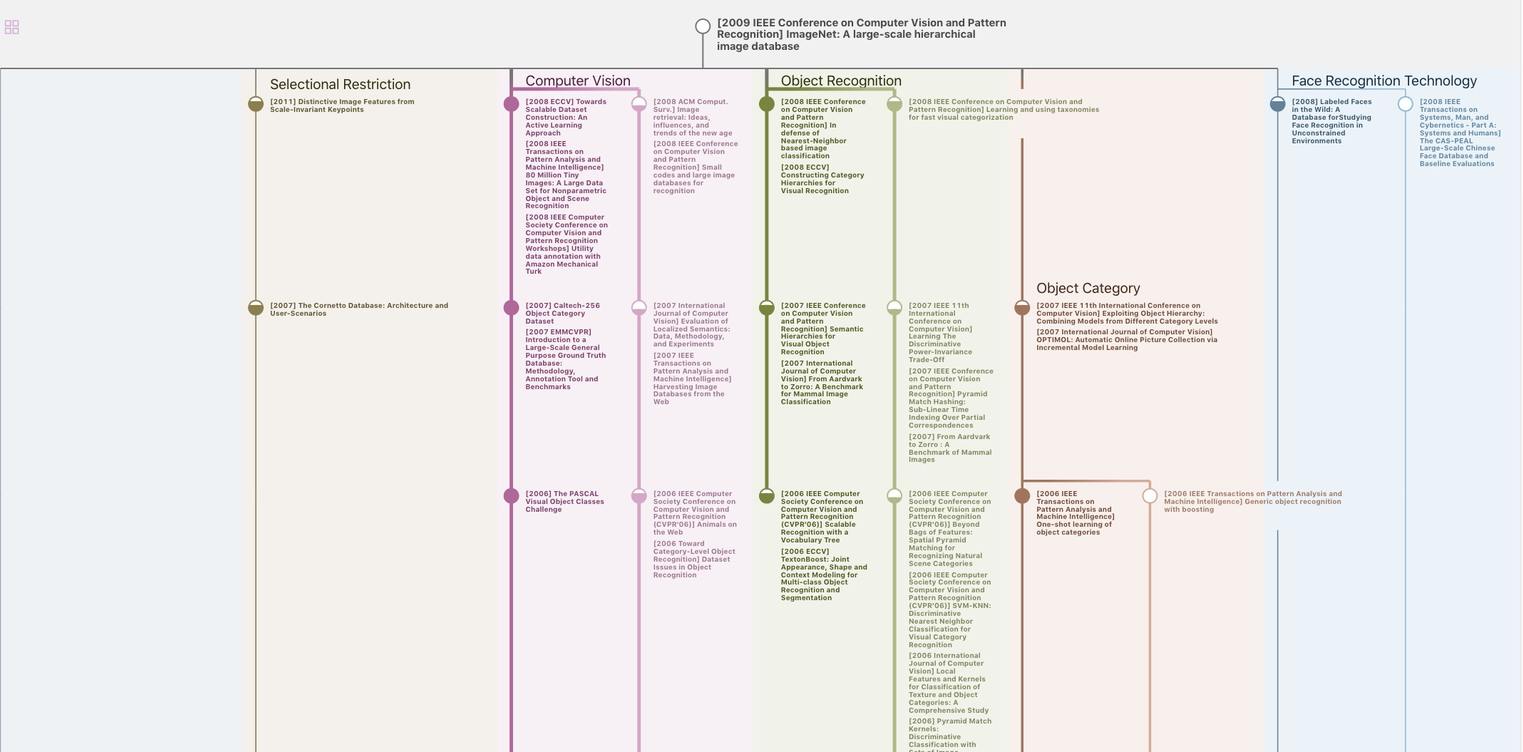
生成溯源树,研究论文发展脉络
Chat Paper
正在生成论文摘要