PIPxResNet: Penalty Induced Prototype-Based eXplainable Residual Neural Network for Heartbeat Classification
Research Square (Research Square)(2021)
摘要
Abstract Early stage heartbeat classification using the electrocardiogram signals can prevent cardiovascular diseases that causes millions of deaths annually around the world. In the past, researchers have used deep neural networks to achieve significant performance for heartbeat classification but their black-box nature and prediction rationale limits real-world deployment. We propose a Penalty Induced Prototype based eXplainable Residual Neural Network (PIPxResNet) that addresses the black-box nature of deep neural networks. PIPxResNet encodes the temporal variations of heartbeats by employing pretrained residual neural network following the concept of task transfer learning. The algorithm further extracts prototypes that are most representative of the training dataset that explain model predictions to general physicians, making them clinically relevant. The prototypes of a particular class having close resemblance to other class prototypes are penalised and their contribution towards corresponding class is reduced. In addition, the classification performance is improved by synthesising regular and irregular heartbeats using a deep convolution conditional generative adversarial network. The proposed method can easily be adopted to other domains that requires explanations for the classification tasks. The PIPxResNet performs at par with existing state-of-the-art algorithms without compromising individual class performance when tested on four publicly available annotated datasets. The proposed model is capable to perform automated screening and provide medical attention by simulating a clinical decision support system for general physicians.
更多查看译文
关键词
heartbeat classification,explainable residual neural network,prototype-based
AI 理解论文
溯源树
样例
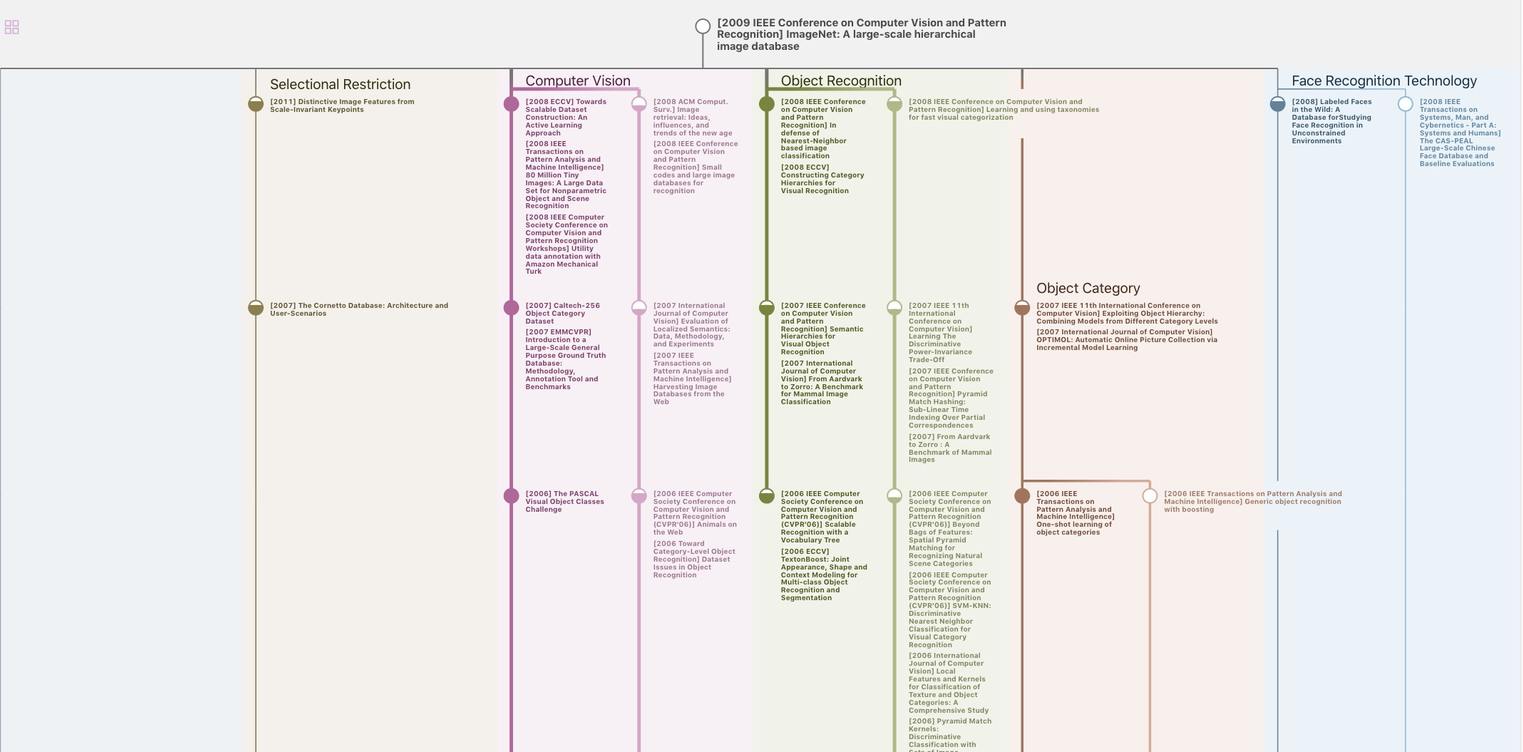
生成溯源树,研究论文发展脉络
Chat Paper
正在生成论文摘要