Automated Labeling of Chest X-ray Images using a Quantitative Explainable Atlas-Based AI Model
Research Square (Research Square)(2021)
摘要
Abstract The inability to accurately, efficiently label large, open-access medical imaging datasets limits the widespread implementation of artificial intelligence models in healthcare. There have been few attempts, however, to automate the annotation of such public databases; one approach, for example, focused on labor-intensive, manual labeling of subsets of these datasets to be used to train new models. In this study, we describe a method for standardized, automated labeling based on similarity to a previously validated, explainable AI model (xAI), using an atlas-based approach, for which the user can specify a quantitative threshold for a desired level of accuracy, the “probability-of-similarity” (pSim) metric. We showed that our xAI model, by calculating the pSim values for each feature based on comparison to its training-set derived reference atlas, could automatically label the external datasets to a user-selected, high level of accuracy, equaling or exceeding that of human experts.
更多查看译文
关键词
chest,images,x-ray,atlas-based
AI 理解论文
溯源树
样例
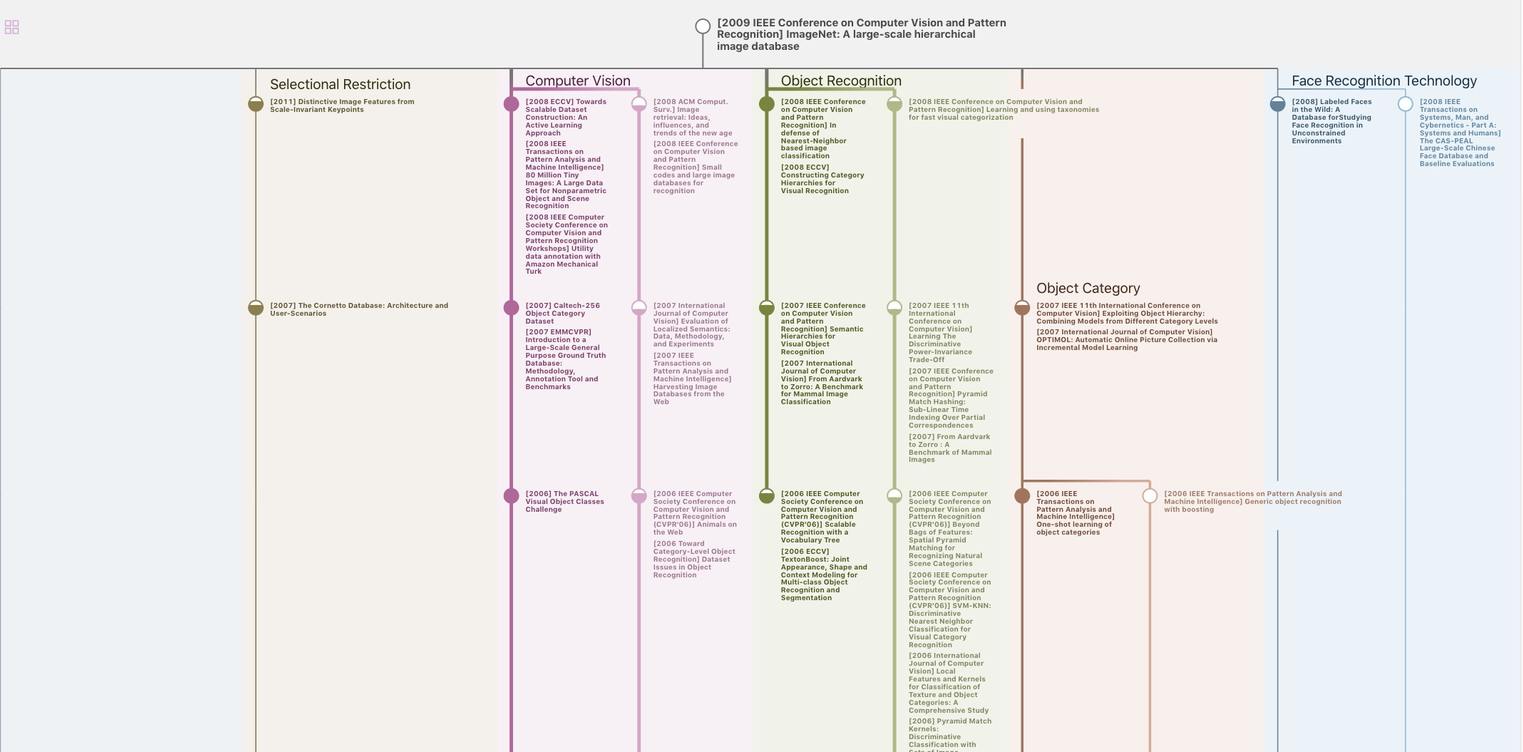
生成溯源树,研究论文发展脉络
Chat Paper
正在生成论文摘要