Development of a Clinical Nomogram for Prediction of Response to Neoadjuvant Chemotherapy in Patients with Advanced Gastric Cancer
Research Square (Research Square)(2021)
摘要
Abstract Objective:The efficacy of neoadjuvant chemotherapy (NAC) among advanced gastric cancer (GC) is still a controversial issue. Our aim is to find the factors associated with chemosensitivity of NAC, and provide optimal therapeutic strategy for GC patients who received NAC.Methods: Clinical information was collected from 230 gastric cancer patients who received NAC in West China Hospital from January 2016 to December 2020. LASSO logistic regression analysis was performed to find the possible predictors which a nomogram model for prediction of response to NAC was based on.Results: A total of 230 patients were finally included in this study, including 154 males (67.0%) and 76 females (33.0%). And mean age was (59.37±10.60) years, ranging from 24 to 80 years. Based on the TRG standard, there were 95 cases in the obvious response group (grade 0 or grade 1) and 135 cases in the non-obvious response group (grade 2 or grade 3), and the obvious response rate was 41.3%. LASSO analysis showed that four risk factors that were significantly related to the efficacy of NAC, which included tumor location (P<0.001) , histological differentiation (P=0.001), clinical T stage (P=0.008) , CA724 (P=0.008) . The C-index for prediction nomogram was 0.806, and the calibration curve revealed the predicted value exhibited good agreement with the actual value, decision curve analysis showed that the nomogram had a good value in clinical application.Conclusions: The nomogram which combined tumor location, histological differentiation,clinical T stage and CA724 showed satisfactory predictive power to response of NAC, and could be used by gastrointestinal surgeons to identify optimal treatment strategy for advanced gastric cancer patients.
更多查看译文
关键词
neoadjuvant chemotherapy,gastric cancer,clinical nomogram
AI 理解论文
溯源树
样例
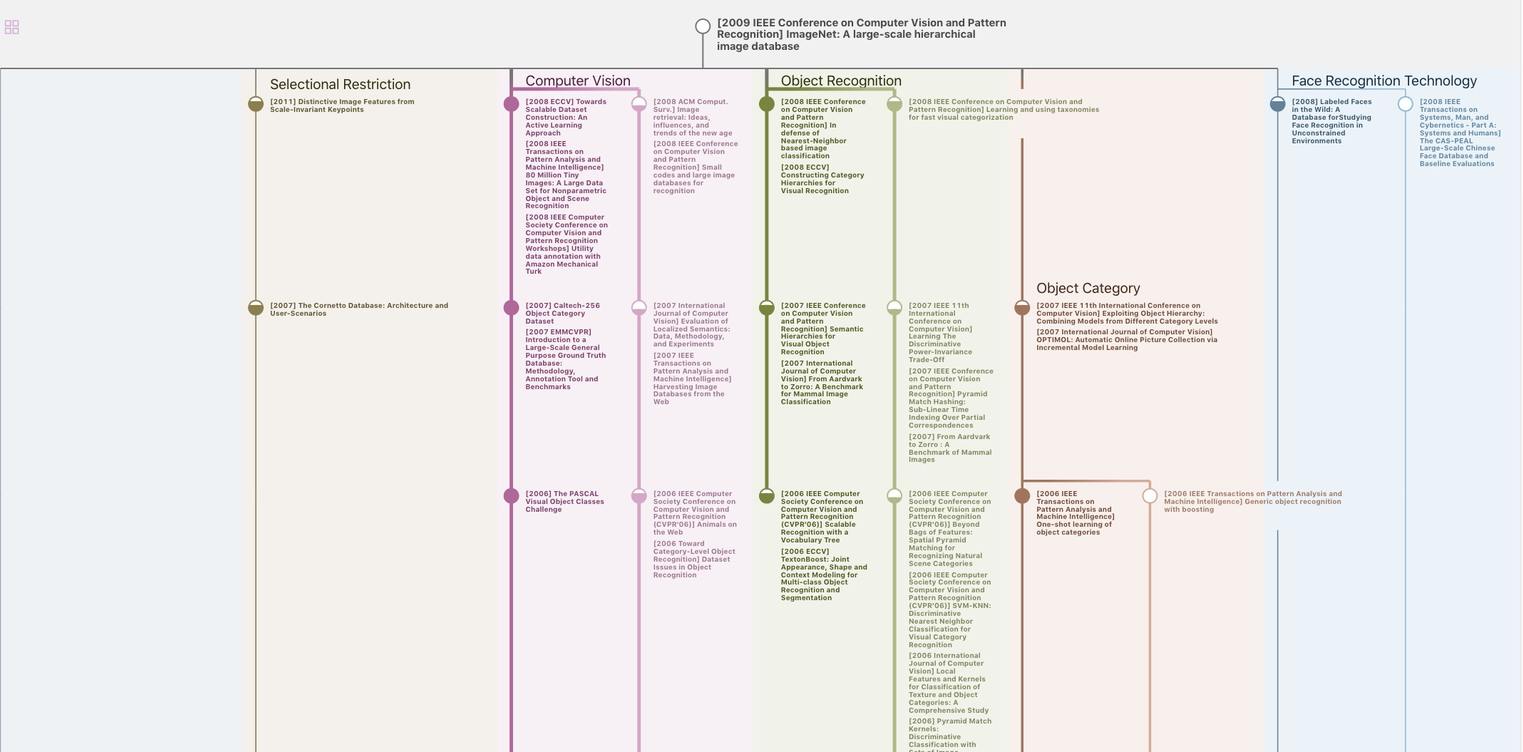
生成溯源树,研究论文发展脉络
Chat Paper
正在生成论文摘要