A Decision Support Framework for Pollution Source Detection via Coupled Forward-Inverse Optimization and Multi-Information Fusion
WATER RESOURCES RESEARCH(2023)
摘要
Due to the uncertainty in sensor data, low model accuracy, and high parameter heterogeneity in water quality modeling, pollution source detection (PSD) typically results in a problem of multiple possible solutions, which is the so-called non-uniqueness effect. Identifying unique solution to PSD problems is fundamentally essential for water quality control in surface water and groundwater systems. This study proposes a decision support framework to reduce the impact of uncertainty and identify a unique solution using a consensus-based multiple information fusion method. Multiple water quality information sources are fused in the framework via spatial clustering and temporal Bayesian updating. Considering the real-world complexity, the recession-curve displacement method is used to handle multi-sources scenario to enhance the accuracy of the framework. Meanwhile, a coupled forward-inverse model instead of random sampling is used to improve the solving efficiency of PSD. The framework is validated by a real water pollution event and a number of semi-hypothetical case studies. The results show that the prediction accuracy of the single-source and double-source pollution problems are 88.40% and 82.69%, with an average relative error of 9.75% and 11.42%, respectively. In addition, the uncertainty contribution quantified by a variance decomposition approach suggests that the interaction between parameter and measurement uncertainties has the greatest impact on the PSD results. The results reveal the advantages of the proposed decision framework for government organizations and communities involved in water environment management.Plain Language Summary There are numerous algorithms for identifying unknown sources of pollution in surface and groundwater resources, which work well in simulation experiments to predict the source of a pollution case. However, in a natural river system, uncertainty issues are literally unavoidable for pollution source detection (PSD) algorithms, such as channel meanders, bed material and aquatic vegetation, fluctuations in monitor data, and data deficits. Algorithms that do not consider the impact of uncertainty reduction measures are giants in computer simulations and dwarfs in practice, ending up with multiple candidate solutions and unable to guide practical water quality management decisions. This study introduces a new approach for PSD that takes uncertainty into account. This approach fuses multiple chemical transport information sources of water quality indicators to reduce the impact of uncertainty and improve the accuracy of PSD under a consensus-based decision framework. Through performance tests on real and computer-generated pollution events, our approach can help water managers identify pollution sources with high reliability.
更多查看译文
关键词
pollution source detection, multi-information fusion, decomposition method, Bayes' updating, uncertainty analysis
AI 理解论文
溯源树
样例
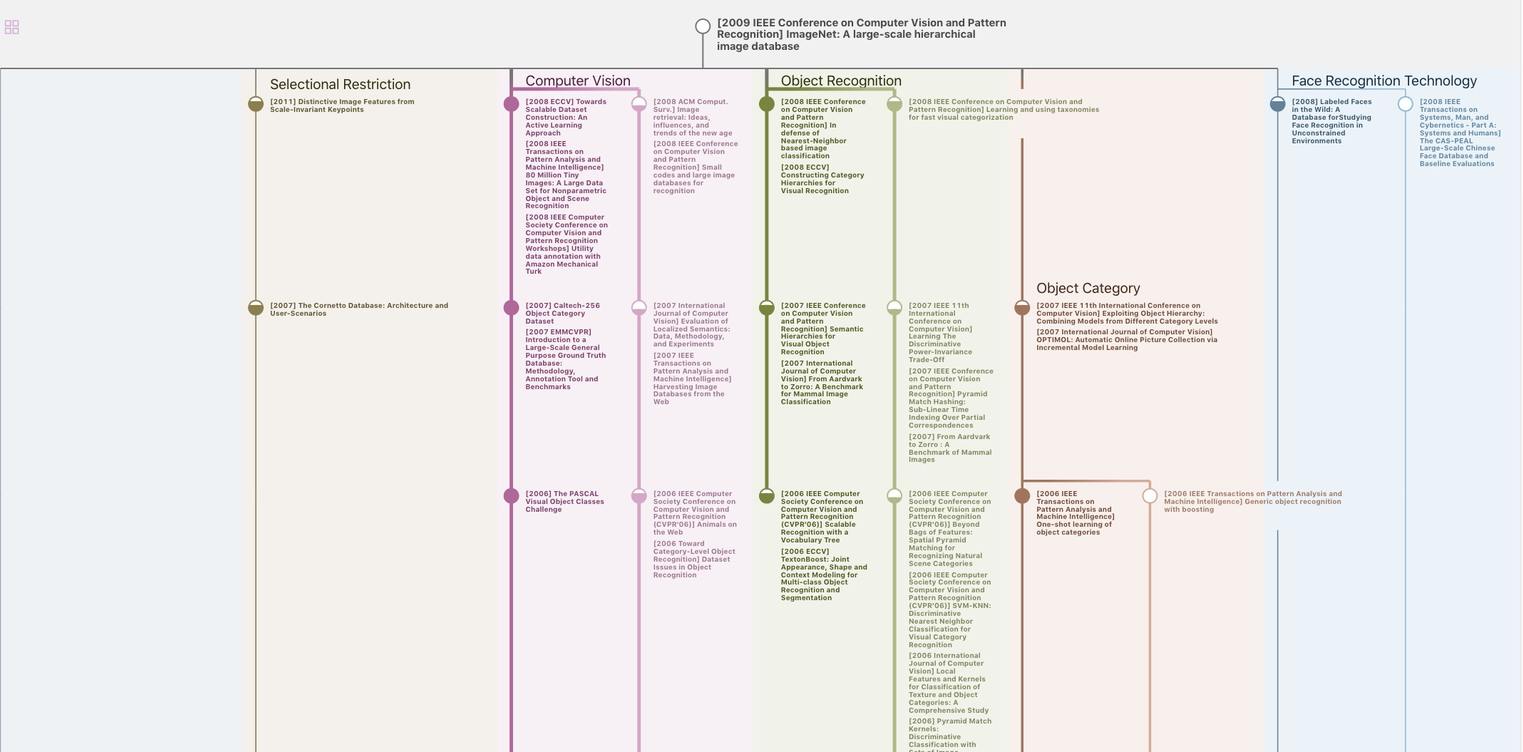
生成溯源树,研究论文发展脉络
Chat Paper
正在生成论文摘要