A systematic approach to predicting and mapping soil particle size distribution from unknown samples using large mid-infrared spectral libraries covering large-scale heterogeneous areas
GEODERMA(2023)
摘要
Soil spectroscopy has the potential to replace wet chemical methods. However, high prediction accuracy of unknown samples is necessary to confidently map predicted values across large spatial scales. This study developed a systematic approach using MIR spectroscopy to predict soil particle size of 9,009 unknown samples, representing an area of approximately 35,716 km(2) in Ireland. A systematic approach for MIR spectroscopy combined with chemometrics was augmented by including spectral control charts to identify unrepresentative spectra in predicting of unknown samples. Moreover, similar to 2% of the predicted values (external validation) were selected, covering a wide range of clay, sand and silt, and analysing them using the classical reference method. This step assesses the accuracy of predicted values and provided confidence when projecting predicted values onto soil particle size maps at multiple regional scales. The MIR models were based on the support vector regression algorithm, and were built using a calibration dataset of similar to 1,000 mineral and organo-mineral (MOM) soils, i.e., non-peat soils, analysed using the pipette method. The model was used to predict soil particle size from 9009 unknown samples described as MOM soils. Spectral control charts correctly identified 97.59% of the peat soils (3078 out of 3154 samples) as 'out of control' samples, which the spectral model should not predict, and about 90% of the MOM soils (5254 out of 5855 samples) were classified as 'under control' samples, which the spectra models can predict. The accuracy calculated for the similar to 2% of samples selected from the MOM unknown samples (n = 5254) was similar to the accuracy in the internal validation set (n = 280), with R-2 val values of 0.90, 0.84 and 0.71, RMSEP values of 2.21, 4.31 and 3.91%; RPIQval values of 4.97, 3.71 and 2.30, for clay, sand and silt prediction, respectively. This systematic approach can predict soil attributes using large spectral libraries, providing confidence for building regional and national scale soil maps.
更多查看译文
关键词
Soil particle size,MIR,Soil texture,Machine learning,Control chart,Chemometrics
AI 理解论文
溯源树
样例
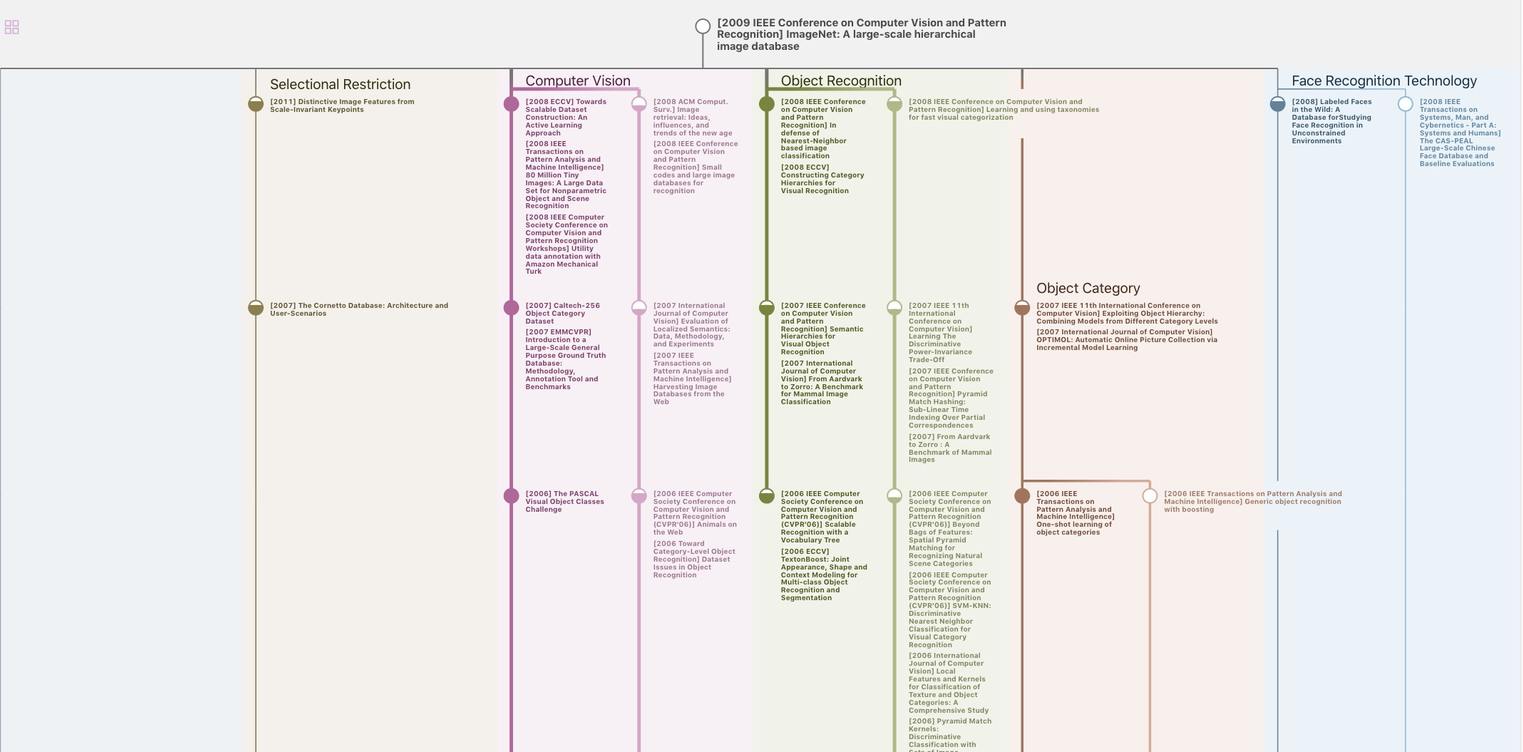
生成溯源树,研究论文发展脉络
Chat Paper
正在生成论文摘要