Accelerated Prediction of Photon Transport in Nanoparticle Media Using Machine Learning Trained With Monte Carlo Simulations
ASME JOURNAL OF HEAT AND MASS TRANSFER(2023)
摘要
Monte Carlo simulations for photon transport are commonly used to predict the spectral response, including reflectance, absorptance, and transmittance in nanoparticle laden media, while the computational cost could be high. In this study, we demonstrate a general purpose fully connected neural network approach, trained with Monte Carlo simulations, to accurately predict the spectral response while dramatically accelerating the computational speed. Monte Carlo simulations are first used to generate a training set with a wide range of optical properties covering dielectrics, semiconductors, and metals. Each input is normalized, with the scattering and absorption coefficients normalized on a logarithmic scale to accelerate the training process and reduce error. A deep neural network with ReLU activation is trained on this dataset with the optical properties and medium thickness as the inputs, and diffuse reflectance, absorptance, and transmittance as the outputs. The neural network is validated on a validation set with randomized optical properties, as well as nanoparticle medium examples including barium sulfate, aluminum, and silicon. The error in the spectral response predictions is within 1% which is sufficient for many applications, while the speedup is 1-3 orders of magnitude. This machine learning accelerated approach can allow for high throughput screening, optimization, or real-time monitoring of nanoparticle media's spectral response.
更多查看译文
关键词
machine learning, artificial neural network, radiative heat transfer, Monte Carlo, nanoparticle media
AI 理解论文
溯源树
样例
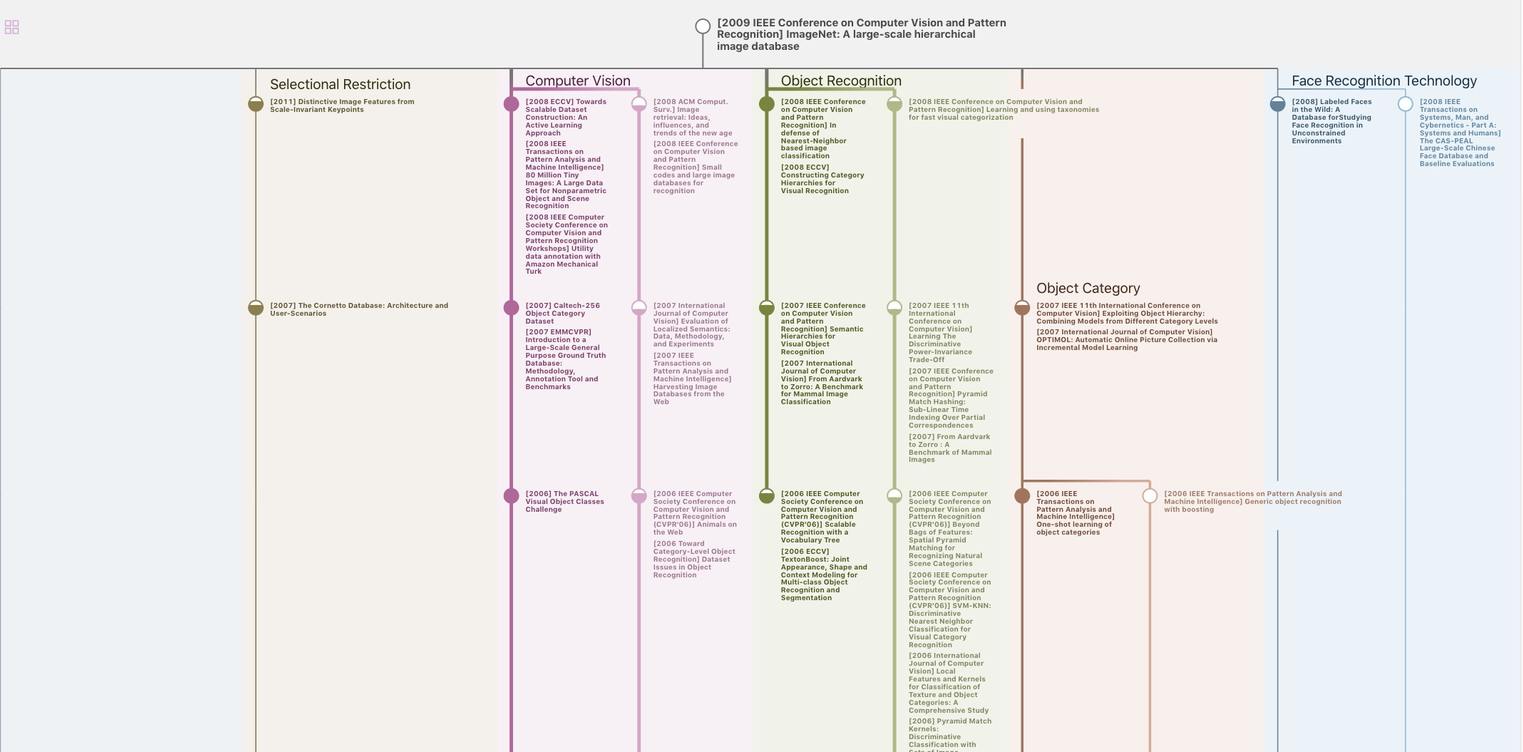
生成溯源树,研究论文发展脉络
Chat Paper
正在生成论文摘要