Fabric defect detection via feature fusion and total variation regularized low-rank decomposition
MULTIMEDIA TOOLS AND APPLICATIONS(2024)
摘要
Fabric defect detection plays a key role in quality control for textile products. Existing methods based on traditional image processing techniques suffer from low detection accuracy and poor adaptability. The low-rank decomposition model is able to decompose the images into sparse parts (defects) and low-rank parts (background), thus can be applied to fabric defect detection. However, such model is likely to maintain more sparse noises in the sparse parts, resulting in high false-positive rate. Meanwhile, it is difficult to comprehensively represent the complicated texture feature of fabric images using traditional single hand-crafted feature descriptors. To remedy the above two challenges, a novel fabric defect detection algorithm based on feature fusion and total variation regularized low-rank decomposition is proposed in this paper. Specifically, the first-order and second-order gradient features are extracted with a biologically inspired model, and then fused using Canonical Correlation Analysis (CCA) to more sufficiently characterize the fabric texture. Next, total variation regularization term is incorporated in low-rank decomposition model to separate fabric images into redundant background and sparse parts with less noise. Finally, the defects are inspected by segmenting the saliency map obtained from sparse matrix using threshold segmentation. The experiment results conducted on two datasets demonstrate that the proposed method has better performance and generalization comparing to the competing methods.
更多查看译文
关键词
Fabric defect detection,Feature fusion,Canonical correlation analysis,Low-rank decomposition,Total variation regularization
AI 理解论文
溯源树
样例
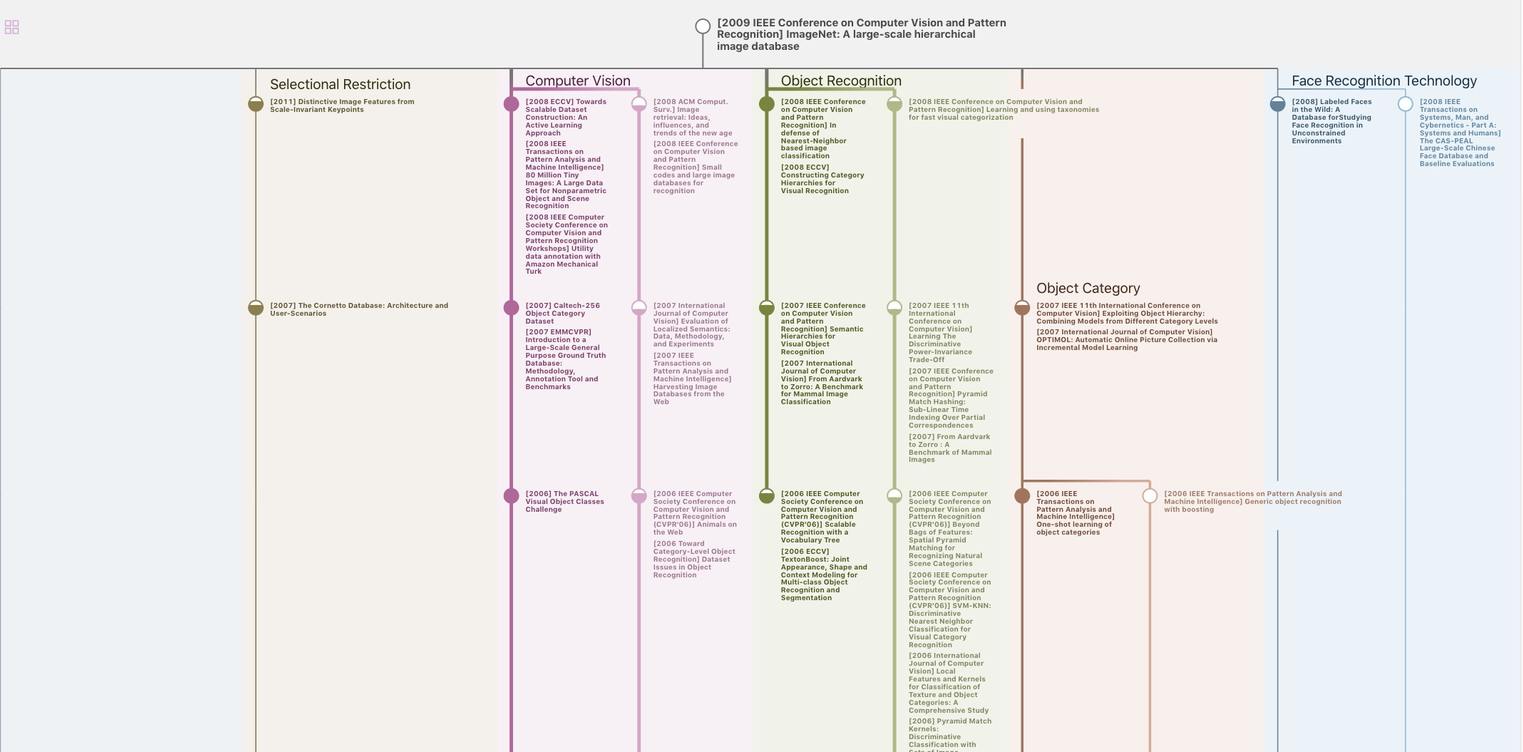
生成溯源树,研究论文发展脉络
Chat Paper
正在生成论文摘要