Accelerated exploration of high-performance multi-principal element alloys: data-driven high-throughput calculations and active learning method
MATERIALS RESEARCH LETTERS(2023)
摘要
We propose an active learning guided density functional theory calculation framework for rapid screening of multi-principal element alloys (MPEAs) with superior mechanical properties. Using this framework, we fast construct the datasets of the bulk modulus (B), shear modulus (G), yield strength, and Pugh's ratio of 12,698 noble metal MPEAs. These datasets were obtained with density functional theory prediction accuracy (R (2 )= 0.98 and 0.96 for B and G, respectively) based on active learning guided 120 DFT calculated data. Analysis of the dataset shows that Ni and Au would enhance the yield strength and the toughness of these noble MPEAs, respectively.IMPACT STATEMENTAn active learning guided density functional theory calculation framework, which reduces computation time by three orders of magnitude, was proposed for rapidly screening multi-principal element alloys with superior mechanical properties.
更多查看译文
关键词
Multi-principal element alloys,mechanical properties,density functional theory,active learning
AI 理解论文
溯源树
样例
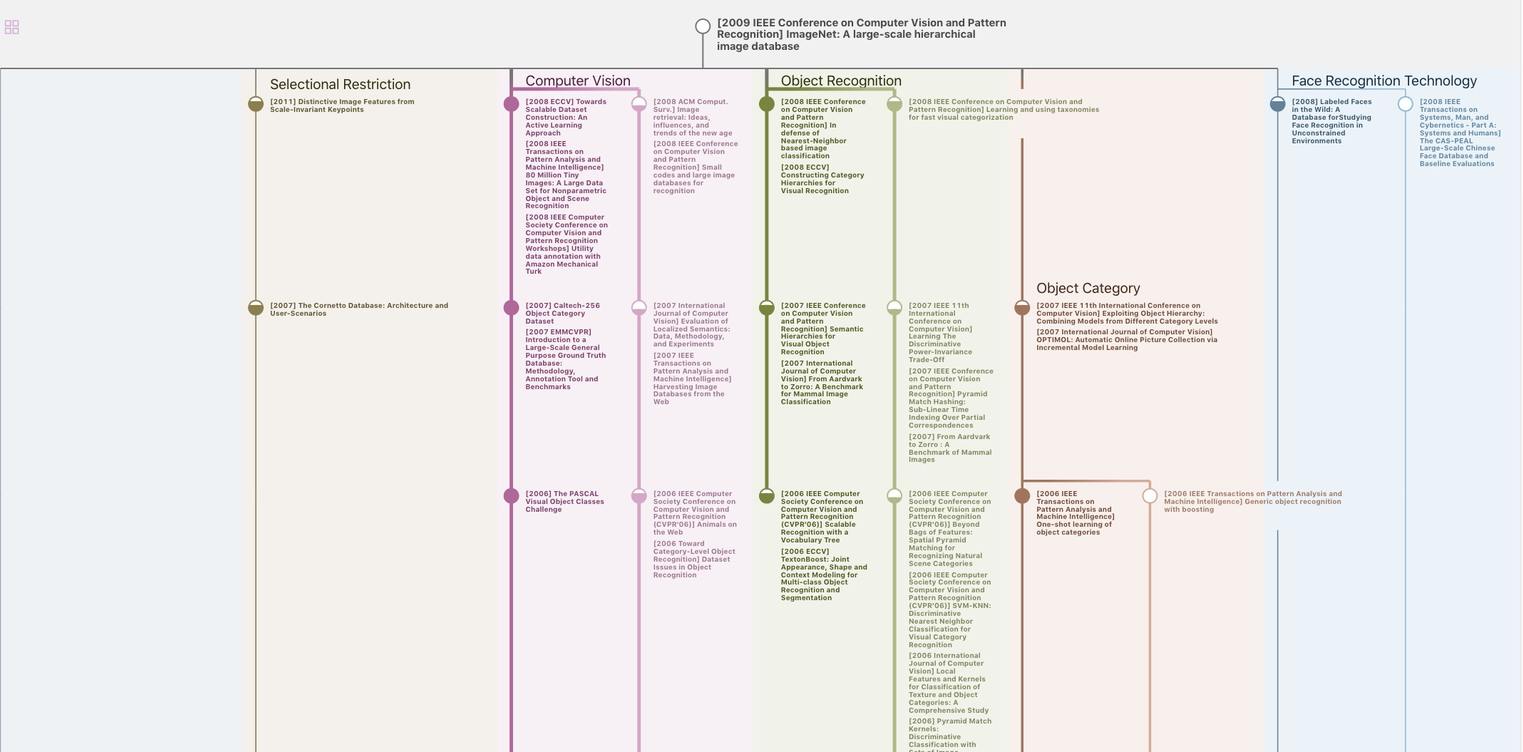
生成溯源树,研究论文发展脉络
Chat Paper
正在生成论文摘要